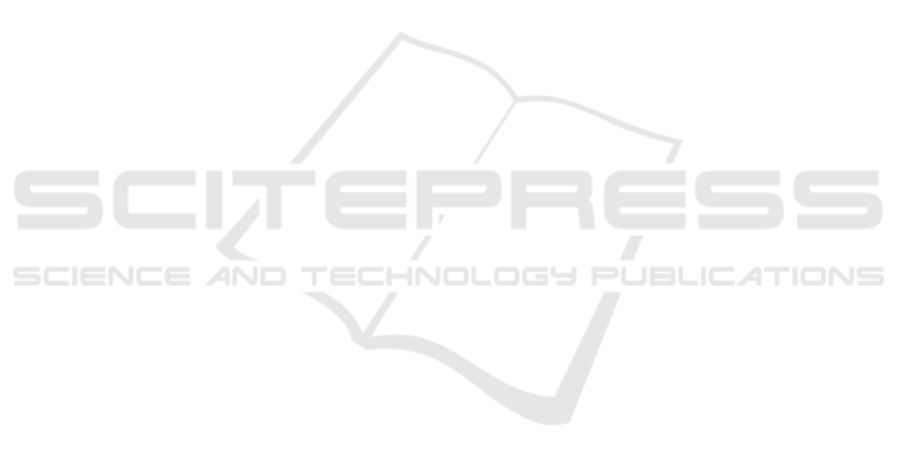
face shape descriptor, referred to as Evolutional Nor-
mal Maps, that assimilates, modifies and optimises a
subset composed of six of these normal map represen-
tations. The proposed descriptor is extensively eval-
uated on three benchmarking 3D face datasets with
very promising results. The descriptor is computa-
tionally efficient and most importantly, it outperforms
the state of the art methods in 3D face recognition.
The proposed Evolutional Normal Maps have
many potential applications, apart from 3D face
recognition. For instance, can can be used for data
augmentation and in generative adversarial networks
to render and develop synthetic 3D data. The future
plans also include their use for 3D face reconstruc-
tion and 3D face analysis for emotion, pose and age
estimation.
ACKNOWLEDGEMENTS
Part of the research in this paper uses the CASIA-3D
FaceV1 collected by the Chinese Academy of Sci-
ences Institute of Automation (CASIA).
This work is partially supported by a grant of the
BMWi ZIM program, no. ZF4029424HB9.
REFERENCES
Abate, A. F., Nappi, M., Ricciardi, S., and Sabatino, G.
(2005). Fast 3d face recognition based on normal
map. In Proceedings of the 2005 International Con-
ference on Image Processing, ICIP 2005, Genoa,
Italy, September 11-14, 2005, pages 946–949. IEEE.
Ahonen, T., Hadid, A., and Pietik
¨
ainen, M. (2004). Face
recognition with local binary patterns. In Pajdla,
T. and Matas, J., editors, Computer Vision - ECCV
2004, 8th European Conference on Computer Vision,
Prague, Czech Republic, May 11-14, 2004. Proceed-
ings, Part I, volume 3021 of Lecture Notes in Com-
puter Science, pages 469–481. Springer.
Aleny
`
a, G., Foix, S., and Torras, C. (2014). Using tof and
RGBD cameras for 3d robot perception and manip-
ulation in human environments. Intelligent Service
Robotics, 7(4):211–220.
Aly
¨
uz, N., G
¨
okberk, B., Dibeklioglu, H., Savran, A., Salah,
A. A., Akarun, L., and Sankur, B. (2008). 3d face
recognition benchmarks on the bosphorus database
with focus on facial expressions. In Schouten, B.
A. M., Juul, N. C., Drygajlo, A., and Tistarelli, M., ed-
itors, Biometrics and Identity Management, First Eu-
ropean Workshop, BIOID 2008, Roskilde, Denmark,
May 7-9, 2008. Revised Selected Papers, volume 5372
of Lecture Notes in Computer Science, pages 57–66.
Springer.
Danner, M., Raetsch, M., Huber, P., Awais, M., Feng, Z.,
and Kittler, J. (2019). Texture-based 3D face recog-
nition using deep neural networks for unconstrained
human-machine interaction. In Proceedings of the
15th International Joint Conference on Computer Vi-
sion, Imaging and Computer Graphics Theory and
Applications VISAPP 2020. SCITEPRESS.
Daoudi, M., Srivastava, A., and Veltkamp, R. (2013). 3D
Face Modeling, Analysis and Recognition. Wiley.
Dou, P., Shah, S. K., and Kakadiaris, I. A. (2017). End-to-
end 3d face reconstruction with deep neural networks.
In 2017 IEEE Conference on Computer Vision and
Pattern Recognition, CVPR 2017, Honolulu, HI, USA,
July 21-26, 2017, pages 1503–1512. IEEE Computer
Society.
Emambakhsh, M. and Evans, A. N. (2017). Nasal patches
and curves for expression-robust 3d face recognition.
IEEE Trans. Pattern Anal. Mach. Intell., 39(5):995–
1007.
Feng, Z.-H., Huber, P., Kittler, J., Hancock, P., Wu, X.-J.,
Zhao, Q., Koppen, P., and R
¨
atsch, M. (2018). Evalua-
tion of dense 3D reconstruction from 2D face images
in the wild. In 2018 13th IEEE International Confer-
ence on Automatic Face & Gesture Recognition (FG
2018), pages 780–786. IEEE.
Gilani, S. Z. and Mian, A. (2018). Learning from mil-
lions of 3d scans for large-scale 3d face recogni-
tion. In 2018 IEEE Conference on Computer Vision
and Pattern Recognition, CVPR 2018, Salt Lake City,
UT, USA, June 18-22, 2018, pages 1896–1905. IEEE
Computer Society.
Gilani, S. Z., Mian, A. S., Shafait, F., and Reid, I. (2018).
Dense 3d face correspondence. IEEE Trans. Pattern
Anal. Mach. Intell., 40(7):1584–1598.
He, K., Zhang, X., Ren, S., and Sun, J. (2016). Deep resid-
ual learning for image recognition. In 2016 IEEE Con-
ference on Computer Vision and Pattern Recognition,
CVPR 2016, Las Vegas, NV, USA, June 27-30, 2016,
pages 770–778. IEEE Computer Society.
Huang, Y., Wang, Y., and Tan, T. (2006). Combining
statistics of geometrical and correlative features for 3d
face recognition. In Chantler, M. J., Fisher, R. B.,
and Trucco, E., editors, Proceedings of the British
Machine Vision Conference 2006, Edinburgh, UK,
September 4-7, 2006, pages 879–888. British Machine
Vision Association.
Huber, P., Hu, G., Tena, J. R., Mortazavian, P., Koppen,
W. P., Christmas, W. J., R
¨
atsch, M., and Kittler, J.
(2016). A multiresolution 3D morphable face model
and fitting framework. In Magnenat-Thalmann, N.,
Richard, P., Linsen, L., Telea, A., Battiato, S., Imai,
F. H., and Braz, J., editors, Proceedings of the 11th
Joint Conference on Computer Vision, Imaging and
Computer Graphics Theory and Applications (VISI-
GRAPP 2016) - Volume 4: VISAPP, Rome, Italy,
February 27-29, 2016, pages 79–86. SciTePress.
Jafari-Khouzani, K. and Soltanian-Zadeh, H. (2005). Radon
transform orientation estimation for rotation invariant
texture analysis. IEEE Trans. Pattern Anal. Mach. In-
tell., 27(6):1004–1008.
Kakadiaris, I. A., Passalis, G., Toderici, G., Murtuza, M. N.,
and Theoharis, T. (2006). 3d face recognition. In
Evolutional Normal Maps: 3D Face Representations for 2D-3D Face Recognition, Face Modelling and Data Augmentation
273