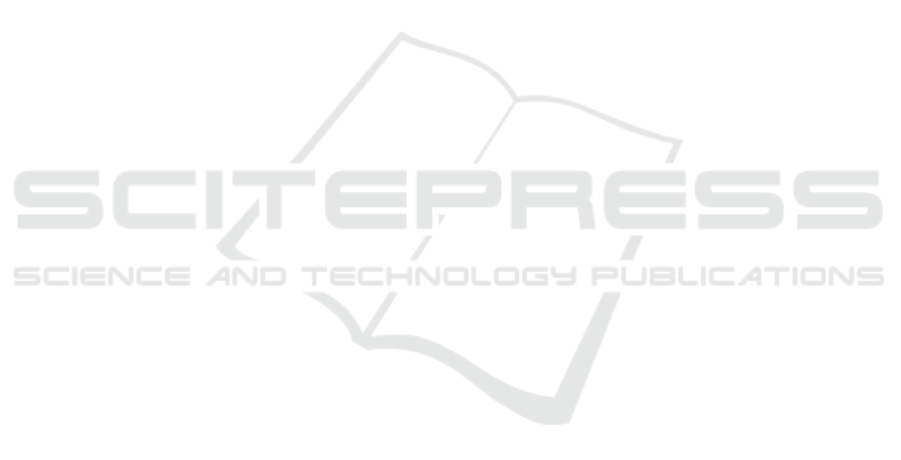
Fradi, H., Bracco, L., Canino, F., and Dugelay, J.-L. (2018).
Autonomous person detection and tracking frame-
work using unmanned aerial vehicles (uavs). In 2018
26th European Signal Processing Conference (EU-
SIPCO), pages 1047–1051. IEEE.
Fradi, H., Fradi, A., and Dugelay, J.-L. (2021). Multi-layer
feature fusion and selection from convolutional neural
networks for texture classification. In VISIGRAPP (4:
VISAPP), pages 574–581.
Ghose, D., Desai, S. M., Bhattacharya, S., Chakraborty, D.,
Fiterau, M., and Rahman, T. (2019). Pedestrian detec-
tion in thermal images using saliency maps. In Pro-
ceedings of the IEEE Conference on Computer Vision
and Pattern Recognition Workshops, pages 0–0.
Girshick, R. (2015). Fast r-cnn. In Proceedings of the IEEE
international conference on computer vision, pages
1440–1448.
Girshick, R., Donahue, J., Darrell, T., and Malik, J. (2014).
Rich feature hierarchies for accurate object detec-
tion and semantic segmentation. In Proceedings of
the IEEE conference on computer vision and pattern
recognition, pages 580–587.
Guan, D., Cao, Y., Yang, J., Cao, Y., and Yang,
M. Y. (2019). Fusion of multispectral data through
illumination-aware deep neural networks for pedes-
trian detection. Information Fusion, 50:148–157.
He, K., Gkioxari, G., Doll
´
ar, P., and Girshick, R. (2017a).
Mask r-cnn. In Proceedings of the IEEE international
conference on computer vision, pages 2961–2969.
He, S., Jiao, J., Zhang, X., Han, G., and Lau, R. W. (2017b).
Delving into salient object subitizing and detection. In
Proceedings of the IEEE International Conference on
Computer Vision, pages 1059–1067.
Hu, X., Fu, C.-W., Zhu, L., Wang, T., and Heng, P.-A.
(2020). Sac-net: Spatial attenuation context for salient
object detection. IEEE Transactions on Circuits and
Systems for Video Technology, 31(3):1079–1090.
Hwang, S., Park, J., Kim, N., Choi, Y., and So Kweon, I.
(2015a). Multispectral pedestrian detection: Bench-
mark dataset and baseline. In Proceedings of the IEEE
conference on computer vision and pattern recogni-
tion, pages 1037–1045.
Hwang, S., Park, J., Kim, N., Choi, Y., and So Kweon, I.
(2015b). Multispectral pedestrian detection: Bench-
mark dataset and baseline. In Proceedings of the IEEE
conference on computer vision and pattern recogni-
tion, pages 1037–1045.
Jiao, Y., Yao, H., and Xu, C. (2020). Pen: Pose-embedding
network for pedestrian detection. IEEE Transac-
tions on Circuits and Systems for Video Technology,
31(3):1150–1162.
Jun, H., Ko, B., Kim, Y., Kim, I., and Kim, J. (2019). Com-
bination of multiple global descriptors for image re-
trieval. arXiv preprint arXiv:1903.10663.
Kieu, M., Bagdanov, A. D., Bertini, M., and Bimbo,
A. D. (2020). Task-conditioned domain adaptation for
pedestrian detection in thermal imagery. In Computer
Vision - ECCV.
Kim, Y.-H., Shin, U., Park, J., and Kweon, I. S. (2021).
Ms-uda: Multi-spectral unsupervised domain adapta-
tion for thermal image semantic segmentation. IEEE
Robotics and Automation Letters, 6(4):6497–6504.
Konig, D., Adam, M., Jarvers, C., Layher, G., Neumann, H.,
and Teutsch, M. (2017). Fully convolutional region
proposal networks for multispectral person detection.
In Proceedings of the IEEE Conference on Computer
Vision and Pattern Recognition Workshops, pages 49–
56.
Li, C., Song, D., Tong, R., and Tang, M. (2018). Multi-
spectral pedestrian detection via simultaneous detec-
tion and segmentation. In British Machine Vision Con-
ference, BMVC.
Li, C., Song, D., Tong, R., and Tang, M. (2019).
Illumination-aware faster r-cnn for robust multi-
spectral pedestrian detection. Pattern Recognition,
85:161–171.
Lin, C., Lu, J., Wang, G., and Zhou, J. (2020). Graininess-
aware deep feature learning for robust pedestrian de-
tection. IEEE transactions on image processing,
29:3820–3834.
Lin, C., Lu, J., and Zhou, J. (2018). Multi-grained deep
feature learning for robust pedestrian detection. IEEE
Transactions on Circuits and Systems for Video Tech-
nology, 29(12):3608–3621.
Lin, T.-Y., Goyal, P., Girshick, R., He, K., and Doll
´
ar, P.
(2017). Focal loss for dense object detection. In
Proceedings of the IEEE international conference on
computer vision, pages 2980–2988.
Liu, J., Zhang, S., Wang, S., and Metaxas, D. (2016a). Mul-
tispectral deep neural networks for pedestrian detec-
tion. In Proceedings of the British Machine Vision
Conference (BMVC).
Liu, J.-J., Hou, Q., and Cheng, M.-M. (2020). Dynamic fea-
ture integration for simultaneous detection of salient
object, edge, and skeleton. IEEE Transactions on Im-
age Processing, 29:8652–8667.
Liu, N., Zhang, N., Wan, K., Shao, L., and Han, J. (2021).
Visual saliency transformer. In Proceedings of the
IEEE/CVF International Conference on Computer Vi-
sion, pages 4722–4732.
Liu, W., Anguelov, D., Erhan, D., Szegedy, C., Reed, S.,
Fu, C.-Y., and Berg, A. C. (2016b). Ssd: Single shot
multibox detector. In European conference on com-
puter vision, pages 21–37. Springer.
Liu, W., Liao, S., Ren, W., Hu, W., and Yu, Y. (2019). High-
level semantic feature detection: A new perspective
for pedestrian detection. In Proceedings of the IEEE
Conference on Computer Vision and Pattern Recogni-
tion, pages 5187–5196.
Marnissi, M. A., Fradi, H., Sahbani, A., and Amara, N.
E. B. (2021a). Thermal image enhancement using
generative adversarial network for pedestrian detec-
tion. In 2020 25th International Conference on Pat-
tern Recognition (ICPR), pages 6509–6516. IEEE.
Marnissi, M. A., Fradi, H., Sahbani, A., and Amara, N.
E. B. (2021b). Unsupervised thermal-to-visible do-
main adaptation method for pedestrian detection. Pat-
tern Recognition Letters.
Nagy, A. M. and Cz
´
uni, L. (2021). Detecting object de-
fects with fusioning convolutional siamese neural net-
works. In VISIGRAPP (5: VISAPP), pages 157–163.
Bispectral Pedestrian Detection Augmented with Saliency Maps using Transformer
283