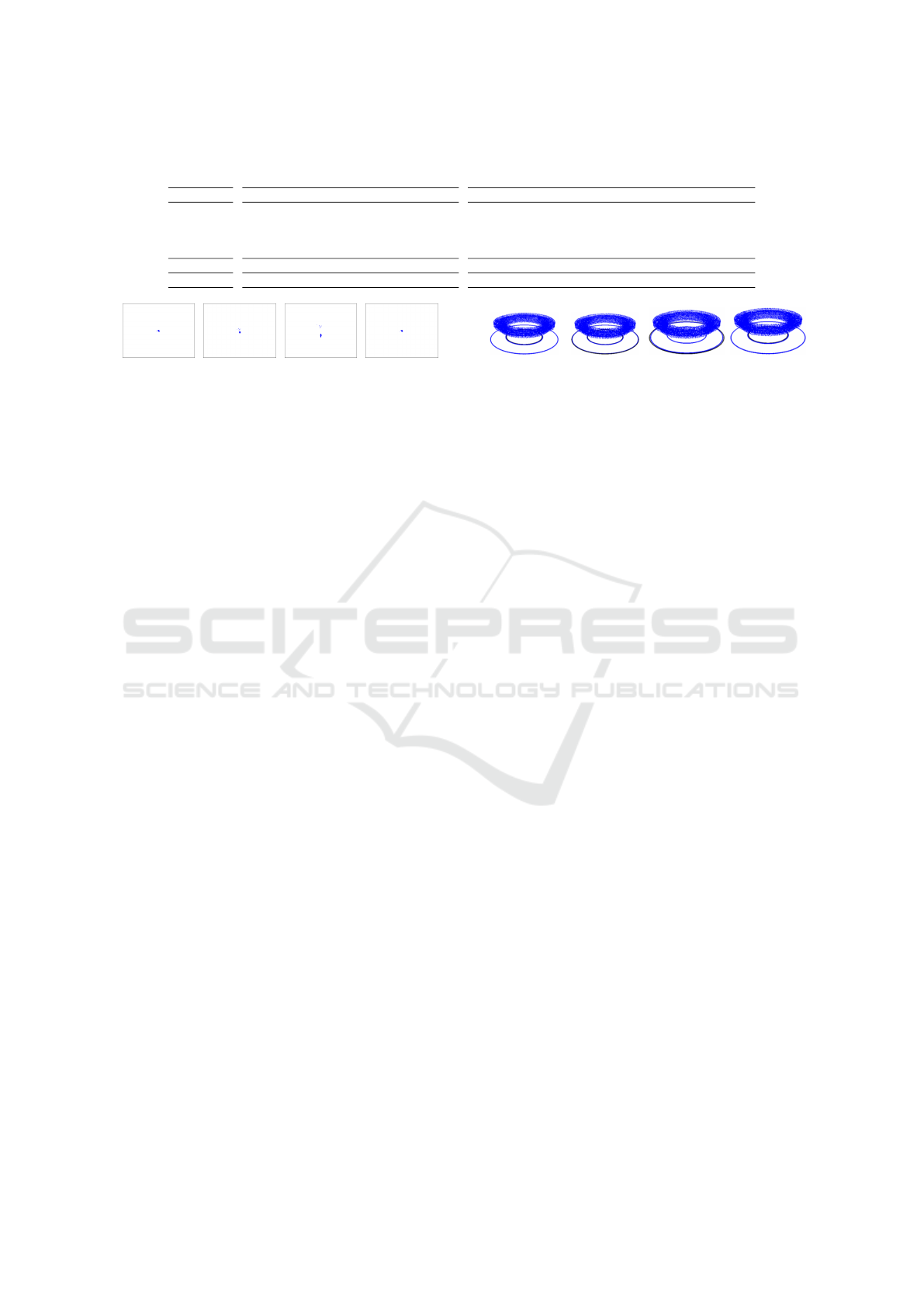
Table 2: NEMA-22 statistics.
Object(s)
Body
Bottom
Shaft
Top
Charuco
All
(a) Visibility.
min(v) max(v) µ(v) ± σ(v)
35.20% 60.76% 46.76 ± 04.35 %
35.26% 61.51% 48.33 ± 04.33 %
32.18% 41.19% 36.67 ± 01.09 %
95.38% 98.44% 96.77 ± 00.46 %
81.84% 87.87% 84.78 ± 01.36 %
32.33% 98.44% 62.72 ± 23.80 %
(a) Depth error.
µ(δ
d
) ± σ(δ
d
) µ(|δ
d
|) ± σ(|δ
d
|) Outliers
−2.275 ± 3.676 mm 3.108 ± 6.165 3.834 %
−0.671 ± 4.803 mm 2.686 ± 5.251 3.664 %
0.819 ± 1.836 mm 1.527 ± 1.487 30.078 %
1.143 ± 4.162 mm 3.163 ± 3.564 11.652 %
1.369 ± 2.595 mm 2.078 ± 2.189 2.628 %
1.089 ± 3.048 mm 2.235 ± 2.606 10.371 %
(a) Bottom (b) Body (c) Top (d) Shaft
Figure 11: NEMA on-screen locations.
(a) Bottom (b) Body (c) Top (d) Shaft
Figure 12: NEMA points of views.
deep models, or that relied on global optimization to
save time that can fail for some images.
Our protocol factories the manual steps and allow
for fast and robust image + label semi-automatic ac-
quisition. It can be applied to any camera. Other hard-
ware pieces are mostly 3D printed. We make the 3D
CAD models, printing settings, software to record the
dataset and the complete dataset available to anyone
for free. We hope people will contribute to the dataset
our create their own using our tools and protocol.
REFERENCES
Association, N. E. M. (2001). Nema ics 16, industrial
control and systems, motion/position, control motors,
controls and feedback devices. Standard, National
Electrical Manufacturers Association, 1300 N. 17th
street, Rosslyn, Virginia 22209.
Besl, P. and McKay, N. D. (1992). A method for registration
of 3-d shapes. IEEE Transactions on Pattern Analysis
and Machine Intelligence, 14(2):239–256.
Brachmann, E., Krull, A., Michel, F., Gumhold, S., Shotton,
J., and Rother, C. (2014). Learning 6d object pose es-
timation using 3d object coordinates. In Fleet, D., Pa-
jdla, T., Schiele, B., and Tuytelaars, T., editors, Com-
puter Vision – ECCV 2014, pages 536–551, Cham.
Springer International Publishing.
Calli, B., Singh, A., Bruce, J., Walsman, A., Konolige, K.,
Srinivasa, S., Abbeel, P., and Dollar, A. M. (2017).
Yale-cmu-berkeley dataset for robotic manipulation
research. The International Journal of Robotics Re-
search, 36(3):261–268.
Garrido-Jurado, S., Mu
˜
noz-Salinas, R., Madrid-Cuevas,
F. J., and Mar
´
ın-Jim
´
enez, M. J. (2014). Auto-
matic generation and detection of highly reliable fidu-
cial markers under occlusion. Pattern Recognition,
47(6):2280–2292.
Hinterstoisser, S., Holzer, S., Cagniart, C., Ilic, S., Kono-
lige, K., Navab, N., and Lepetit, V. (2011). Multi-
modal templates for real-time detection of texture-less
objects in heavily cluttered scenes. pages 858–865.
Hinterstoisser, S., Lepetit, V., Ilic, S., Holzer, S., Bradski,
G., Konolige, K., and Navab, N. (2013). Model based
training, detection and pose estimation of texture-less
3d objects in heavily cluttered scenes. In Lee, K. M.,
Matsushita, Y., Rehg, J. M., and Hu, Z., editors, Com-
puter Vision – ACCV 2012, pages 548–562, Berlin,
Heidelberg. Springer Berlin Heidelberg.
Hoda
ˇ
n, T., Haluza, P., Obdr
ˇ
z
´
alek,
ˇ
S., Matas, J., Lourakis,
M., and Zabulis, X. (2017). T-LESS: An RGB-D
dataset for 6D pose estimation of texture-less objects.
IEEE Winter Conference on Applications of Computer
Vision (WACV).
Hoda
ˇ
n, T., Sundermeyer, M., Drost, B., Labb
´
e, Y., Brach-
mann, E., Michel, F., Rother, C., and Matas, J. (2020).
BOP challenge 2020 on 6D object localization. Euro-
pean Conference on Computer Vision Workshops (EC-
CVW).
Osher, S. and Fedkiw, R. (2003). Signed Distance Func-
tions, pages 17–22. Springer New York, New York,
NY.
Peng, S., Liu, Y., Huang, Q., Zhou, X., and Bao, H. (2019).
Pvnet: Pixel-wise voting network for 6dof pose esti-
mation. In CVPR.
Pitteri, G., Ramamonjisoa, M., Ilic, S., and Lepetit, V.
(2019). On object symmetries and 6d pose estimation
from images. International Conference on 3D Vision.
Rad, M. and Lepetit, V. (2017). BB8: A scalable, accurate,
robust to partial occlusion method for predicting the
3d poses of challenging objects without using depth.
CoRR, abs/1703.10896.
Tekin, B., Sinha, S. N., and Fua, P. (2017). Real-time
seamless single shot 6d object pose prediction. CoRR,
abs/1711.08848.
Tremblay, J., To, T., and Birchfield, S. (2018). Falling
things: A synthetic dataset for 3d object detection and
pose estimation. CoRR, abs/1804.06534.
Xiang, Y., Schmidt, T., Narayanan, V., and Fox, D. (2017).
Posecnn: A convolutional neural network for 6d
object pose estimation in cluttered scenes. CoRR,
abs/1711.00199.
VISAPP 2022 - 17th International Conference on Computer Vision Theory and Applications
690