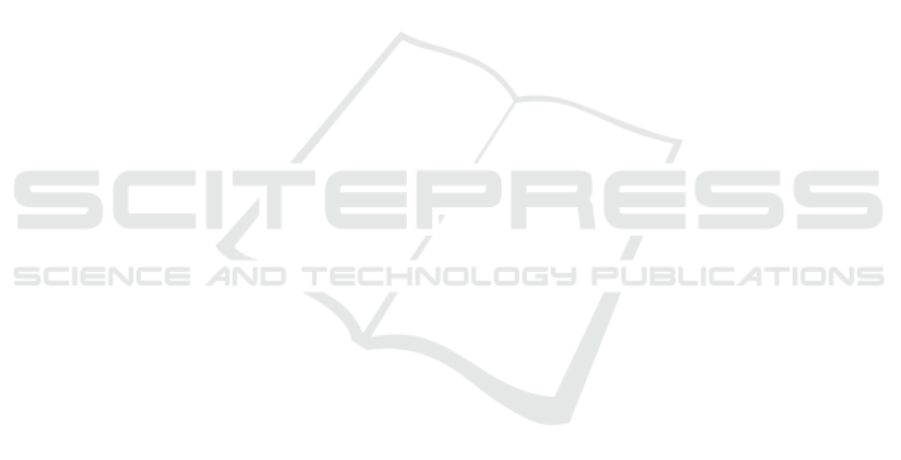
the variability in driving performance (Gemonet et al.,
2021).
In addition, our simulator did not inform the sub-
ject of his decisions and the choices of trajectories he
took, which led subjects to have very little confidence
in it and to remain very attentive to the road. Many
subjects reported that they considered themselves to
be fully attentive and that the distracting task had lit-
tle impact on their performance.
5.4 Perspectives for the Attention Model
Our results are encouraging but the attention model
is totally binary, meaning that one is considered by
the system to be either completely attentive or com-
pletely inattentive, and eye-on-road type phenomena
cannot be taken into account. It should be improved
by integrating real knowledge of the situation in order
to obtain noticeable differences in performance.
In particular, it would be necessary to add seman-
tic elements of the situation and to make assumptions
about elements of the scene. The ARI model proposes
a study of the road staring time, but it would be neces-
sary to give attention to the staring time of the impor-
tant elements in the scene such as the vehicle in front,
the obstacle or the other vehicles.
During the automation phase, numerous subjects
express verbally of by moving their body their dis-
agreement with the automated driving system deci-
sion (for example, slowing down). These visual and
verbal behaviors should be integrated in our model.
6 CONCLUSION AND FUTURE
WORKS
In this article, we present a new model of adaptive
visual feedback for takeover in highly automated ve-
hicle. This model is based on Herzberger’s attention
estimation. We developed a complete system in a
virtual reality device (CAVE-like environment) with
a driving simulator allowing the creation of specific
scenarios and the data collection of the user’s behav-
ior. Adaptive feedback were displayed through the
virtual environment (mixed reality).
Experiment were lead on around twenty partici-
pants of different ages. Results tend to show that user
have slightly better performances using an adapta-
tion model and feel less mental workload while taking
over the vehicle. However, the results should be cor-
roborated with future experiment on a more complex
model. This experiment allowed us to collect data on
driver’s gaze behavior and gestures during takeover.
We plan to improve this first model to obtain more
detailed information on driver readiness and thus im-
prove our adaptation model. Our work will aim at
correlating particular behaviors with some poor per-
formances recorded, in order to improve the feedback
and simplify the interface by restricting the number of
options displayed and then we will be able to test the
effectiveness of a more complex adaptive model.
ACKNOWLEDGEMENT
We thanks gratefully the FEDER (Fond europ
´
een de
d
´
eveloppement r
´
egional) and the UTC foundation for
research for their funding for this project. We also
thank Yohan Bouvet for his technical assistance on
the project.
REFERENCES
Bengler, K., Rettenmaier, M., Fritz, N., and Feierle, A.
(2020). From HMI to HMIs: Towards an HMI Frame-
work for Automated Driving. Information.
de Winter, J., Stanton, N., and Eisma, Y. (2021). Is the take-
over paradigm a mere convenience? Transportation
Research Interdisciplinary Perspectives.
Deo, N. and Trivedi, M. M. (2020). Looking at the
Driver/Rider in Autonomous Vehicles to Predict Take-
Over Readiness. IEEE Transactions on Intelligent Ve-
hicles.
Endsley, M. R. (1999). Level of automation effects on per-
formance, situation awareness and workload in a dy-
namic control task. Ergonomics.
Eriksson, A., Petermeijer, S. M., Zimmermann, M., de Win-
ter, J. C. F., Bengler, K. J., and Stanton, N. A.
(2019). Rolling Out the Red (and Green) Carpet:
Supporting Driver Decision Making in Automation-
to-Manual Transitions. IEEE Transactions on Human-
Machine Systems, 49.
Gemonet, E., Bougard, C., Honnet, V., Poueyo, M., Mas-
frand, S., and Mestre, D. R. (2021). Drivers’ perfor-
mances and their subjective feelings about their driv-
ing during a 40-min test on a circuit versus a dynamic
simulator. Transportation Research Part F: Traffic
Psychology and Behaviour.
Gold, C., K
¨
orber, M., Lechner, D., and Bengler, K. (2016).
Taking Over Control From Highly Automated Vehi-
cles in Complex Traffic Situations The Role of Traffic
Density. Human Factors: The Journal of the Human
Factors and Ergonomics Society.
Haeuslschmid, R., Shou, Y., O’Donovan, J., Burnett, G.,
and Butz, A. (2016). First Steps towards a View Man-
agement Concept for Large-sized Head-up Displays
with Continuous Depth. In Proceedings of the 8th
International Conference on Automotive User Inter-
faces and Interactive Vehicular Applications - Auto-
motive’UI 16, Ann Arbor, MI, USA. ACM Press.
HUCAPP 2022 - 6th International Conference on Human Computer Interaction Theory and Applications
106