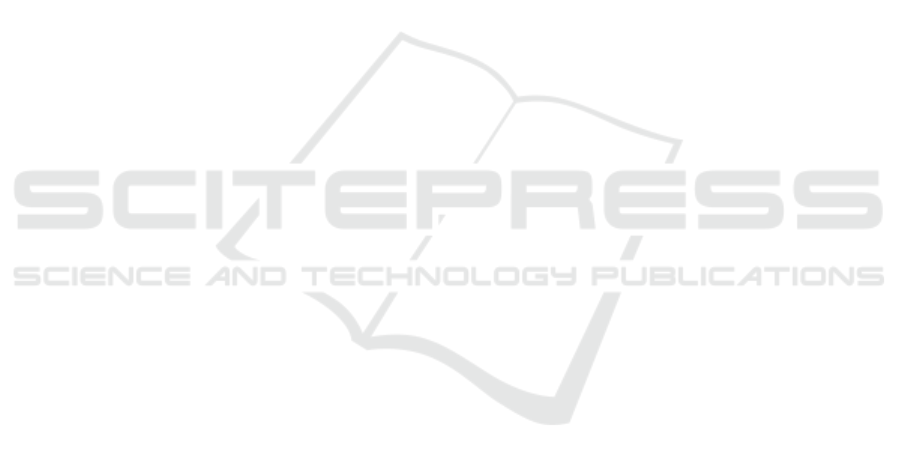
He, K., Zhang, X., Ren, S., and Sun, J. (2016). Deep resid-
ual learning for image recognition. In Proceedings of
the IEEE Conference on Computer Vision and Pattern
Recognition (CVPR).
Khamis, S., Fanello, S., Rhemann, C., Kowdle, A.,
Valentin, J., and Izadi, S. (2018). StereoNet:
Guided hierarchical refinement for real-time edge-
aware depth prediction. Lecture Notes in Computer
Science (including subseries Lecture Notes in Artifi-
cial Intelligence and Lecture Notes in Bioinformatics),
11219 LNCS:596–613.
Ma, N., Zhang, X., Zheng, H.-T., and Sun, J. (2018). Shuf-
flenet v2: Practical guidelines for efficient cnn archi-
tecture design. In Proceedings of the European Con-
ference on Computer Vision (ECCV).
Mayer, N., Ilg, E., H
¨
ausser, P., Fischer, P., Cremers, D.,
Dosovitskiy, A., and Brox, T. (2016). A large dataset
to train convolutional networks for disparity, optical
flow, and scene flow estimation. In IEEE International
Conference on Computer Vision and Pattern Recogni-
tion (CVPR).
Mayer, N., Ilg, E., H
¨
ausser, P., Fischer, P., Cremers, D.,
Dosovitskiy, A., and Brox, T. (2016). A large dataset
to train convolutional networks for disparity, optical
flow, and scene flow estimation. In 2016 IEEE Con-
ference on Computer Vision and Pattern Recognition
(CVPR), pages 4040–4048.
Menze, M., Heipke, C., and Geiger, A. (2015). Joint 3d esti-
mation of vehicles and scene flow. In ISPRS Workshop
on Image Sequence Analysis (ISA).
Menze, M., Heipke, C., and Geiger, A. (2018). Object scene
flow. ISPRS Journal of Photogrammetry and Remote
Sensing (JPRS).
Shen, Z., Dai, Y., and Rao, Z. (2020). Msmd-net: Deep
stereo matching with multi-scale and multi-dimension
cost volume. CoRR, abs/2006.12797.
Shomron, G., Banner, R., Shkolnik, M., and Weiser, U.
(2020). Thanks for nothing: Predicting zero-valued
activations with lightweight convolutional neural net-
works. In Computer Vision – ECCV 2020, pages 234–
250.
Szegedy, C., Liu, W., Jia, Y., Sermanet, P., Reed, S.,
Anguelov, D., Erhan, D., Vanhoucke, V., and Rabi-
novich, A. (2015). Going deeper with convolutions.
In Proceedings of the IEEE Conference on Computer
Vision and Pattern Recognition (CVPR).
Tankovich, V., H
¨
ane, C., Fanello, S. R., Zhang, Y., Izadi, S.,
and Bouaziz, S. (2020). Hitnet: Hierarchical iterative
tile refinement network for real-time stereo matching.
CoRR, abs/2007.12140.
Teerapittayanon, S., McDanel, B., and Kung, H. (2016).
Branchynet: Fast inference via early exiting from
deep neural networks. In 2016 23rd International
Conference on Pattern Recognition (ICPR), pages
2464–2469.
Wang, Q., Shi, S., Zheng, S., Zhao, K., and Chu, X. (2020).
Fadnet: A fast and accurate network for disparity es-
timation. In 2020 IEEE International Conference on
Robotics and Automation (ICRA), pages 101–107.
Wang, Y., Lai, Z., Huang, G., Wang, B. H., van der Maaten,
L., Campbell, M., and Weinberger, K. Q. (2019). Any-
time stereo image depth estimation on mobile devices.
In 2019 International Conference on Robotics and Au-
tomation (ICRA), pages 5893–5900.
Xing, J., Qi, Z., Dong, J., Cai, J., and Liu, H. (2020). Mab-
net: A lightweight stereo network based on multi-
branch adjustable bottleneck module. In Computer
Vision – ECCV 2020, pages 340–356.
Xu, H. and Zhang, J. (2020). Aanet: Adaptive aggregation
network for efficient stereo matching. In Proceedings
of the IEEE/CVF Conference on Computer Vision and
Pattern Recognition (CVPR).
Yee, K. and Chakrabarti, A. (2020). Fast deep stereo with
2d convolutional processing of cost signatures. In Pro-
ceedings of the IEEE/CVF Winter Conference on Ap-
plications of Computer Vision (WACV).
Zhang, F., Prisacariu, V., Yang, R., and Torr, P. H. (2019).
Ga-net: Guided aggregation net for end-to-end stereo
matching. In Proceedings of the IEEE/CVF Con-
ference on Computer Vision and Pattern Recognition
(CVPR).
VISAPP 2022 - 17th International Conference on Computer Vision Theory and Applications
766