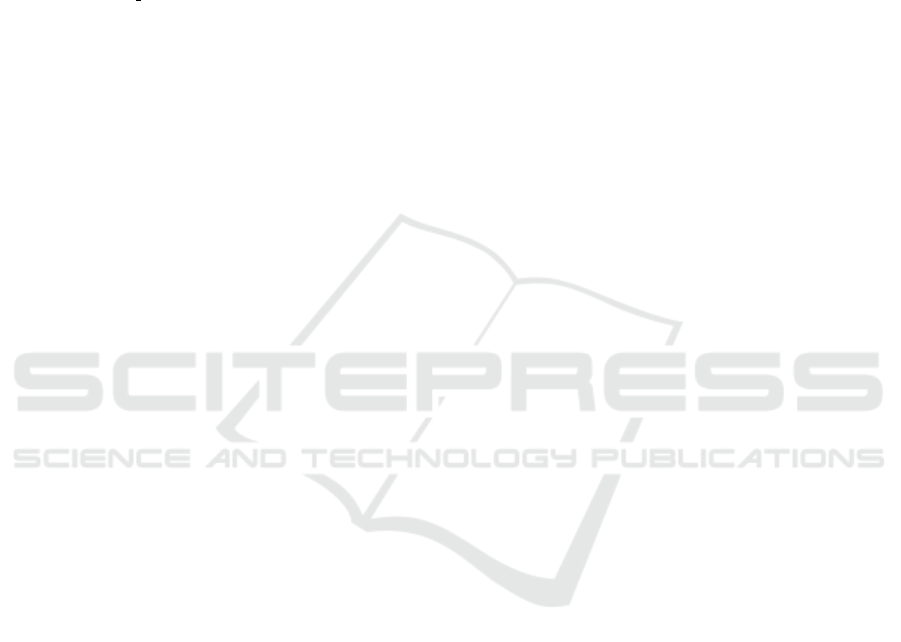
editors, 13th International Conference on Medical In-
formation Processing and Analysis, volume 10572,
pages 326 – 336. Int. Society for Optics and Photon-
ics, SPIE.
Haindl, M. (2012). Visual data recognition and model-
ing based on local markovian models. In Florack,
L., Duits, R., Jongbloed, G., Lieshout, M.-C., and
Davies, L., editors, Mathematical Methods for Signal
and Image Analysis and Representation, volume 41
of Computational Imaging and Vision, chapter 14,
pages 241–259. Springer London. 10.1007/978-1-
4471-2353-8 14.
He, K., Zhang, X., Ren, S., and Sun, J. (2016). Deep resid-
ual learning for image recognition. In Proceedings of
the IEEE conference on computer vision and pattern
recognition, pages 770–778.
Hosny, K. M., Kassem, M. A., and Foaud, M. M. (2020).
Skin melanoma classification using roi and data aug-
mentation with deep convolutional neural networks.
Multimedia Tools and Applications, 79(33):24029–
24055.
Kawahara, J., BenTaieb, A., and Hamarneh, G. (2016).
Deep features to classify skin lesions. In 2016 IEEE
13th International Symposium on Biomedical Imaging
(ISBI), pages 1397–1400. IEEE.
Kawahara, J. and Hamarneh, G. (2019). Visual diagnosis of
dermatological disorders: Human and machine per-
formance.
Khan, M. A., Zhang, Y.-D., Sharif, M., and Akram,
T. (2021). Pixels to classes: intelligent learning
framework for multiclass skin lesion localization and
classification. Computers & Electrical Engineering,
90:106956.
Krizhevsky, A., Sutskever, I., and Hinton, G. E. (2012). Im-
agenet classification with deep convolutional neural
networks. In Advances in neural information process-
ing systems, volume 25, pages 1097–1105.
Mahbod, A., Schaefer, G., Ellinger, I., Ecker, R., Pitiot, A.,
and Wang, C. (2019). Fusing fine-tuned deep features
for skin lesion classification. Computerized Medical
Imaging and Graphics, 71:19–29.
Navarrete-Dechent, C., Dusza, S. W., Liopyris, K.,
Marghoob, A. A., Halpern, A. C., and Marchetti,
M. A. (2018). Automated dermatological diagnosis:
hype or reality? The Journal of investigative derma-
tology, 138(10):2277.
Nida, N., Irtaza, A., Javed, A., Yousaf, M. H., and Mah-
mood, M. T. (2019). Melanoma lesion detection and
segmentation using deep region based convolutional
neural network and fuzzy c-means clustering. Inter-
national journal of medical informatics, 124:37–48.
Reme
ˇ
s, V. and Haindl, M. (2018). Rotationally invariant
bark recognition. In X., B., E., H., T., H., R., W., B.,
B., and A., R.-K., editors, IAPR Joint International
Workshop on Statistical Techniques in Pattern Recog-
nition and Structural and Syntactic Pattern Recogni-
tion (S+SSPR 2018), volume 11004 of Lecture Notes
in Computer Science, pages 22 – 31. Springer Nature
Switzerland AG.
Rotemberg, V., Kurtansky, N., Betz-Stablein, B., Caffery,
L., Chousakos, E., Codella, N., Combalia, M., Dusza,
S., Guitera, P., Gutman, D., et al. (2021). A patient-
centric dataset of images and metadata for identify-
ing melanomas using clinical context. Scientific data,
8(1):1–8.
Shakourian Ghalejoogh, G., Montazery Kordy, H., and
Ebrahimi, F. (2019). A hierarchical structure based on
stacking approach for skin lesion classification. Ex-
pert Systems with Applications, 145:113127.
Szegedy, C., Liu, W., Jia, Y., Sermanet, P., Reed, S.,
Anguelov, D., Erhan, D., Vanhoucke, V., and Rabi-
novich, A. (2015). Going deeper with convolutions.
In Proceeding of the IEEE conference on computer
vision and pattern recognition, pages 1–9.
Tschandl, P., Rosendahl, C., and Kittler, H. (2018). The
ham10000 dataset, a large collection of multi-source
dermatoscopic images of common pigmented skin le-
sions. Scientific data, 5(1):1–9.
Tschandl, P., Sinz, C., and Kittler, H. (2019). Domain-
specific classification-pretrained fully convolutional
network encoders for skin lesion segmentation. Com-
puters in biology and medicine, 104:111–116.
Zhang, J., Xie, Y., Xia, Y., and Shen, C. (2019). Attention
residual learning for skin lesion classification. IEEE
transactions on medical imaging, 38(9):2092–2103.
Zhang, N., Cai, Y.-X., Wang, Y.-Y., Tian, Y.-T., Wang, X.-
L., and Badami, B. (2020). Skin cancer diagnosis
based on optimized convolutional neural network. Ar-
tificial intelligence in medicine, 102:101756.
Melanoma Recognition
729