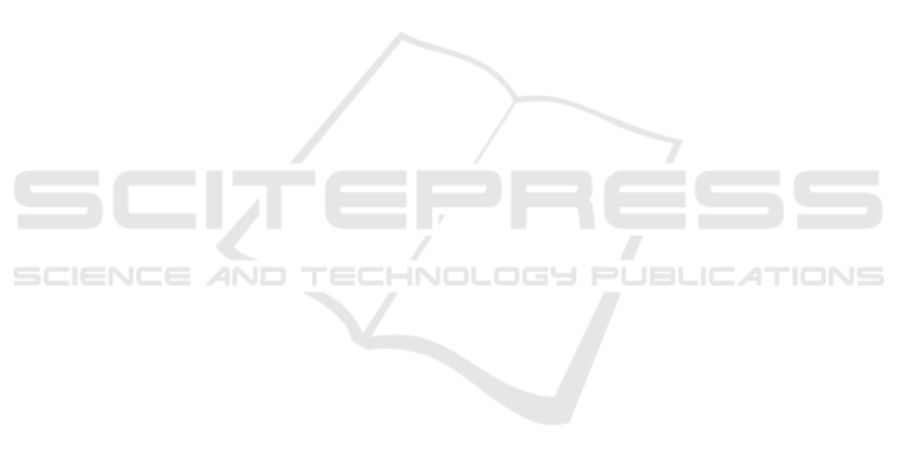
ACKNOWLEDGEMENTS
This work is supported by the ICUB project 2017
ANR program : ANR-17-CE22-0011.
REFERENCES
Ainouz, S., Morel, O., Fofi, D., Mosaddegh, S., and
Bensrhair, A. (2013). Adaptive processing of cata-
dioptric images using polarization imaging: towards
a pola-catadioptric model. Optical engineering,
52(3):037001.
Aldibaja, M., Suganuma, N., and Yoneda, K. (2016). Im-
proving localization accuracy for autonomous driving
in snow-rain environments. In 2016 IEEE/SICE In-
ternational Symposium on System Integration (SII),
pages 212–217. IEEE.
Bass, M., Van Stryland, E. W., Williams, D. R., and
Wolfe, W. L. (1995). Handbook of optics, volume 2.
McGraw-Hill New York.
Bijelic, M., Gruber, T., Mannan, F., Kraus, F., Ritter, W.,
Dietmayer, K., and Heide, F. (2020). Seeing through
fog without seeing fog: Deep multimodal sensor fu-
sion in unseen adverse weather. In Proceedings of the
IEEE/CVF Conference on Computer Vision and Pat-
tern Recognition, pages 11682–11692.
Bijelic, M., Gruber, T., and Ritter, W. (2018). Benchmark-
ing image sensors under adverse weather conditions
for autonomous driving. In 2018 IEEE Intelligent Ve-
hicles Symposium (IV), pages 1773–1779. IEEE.
Blin, R., Ainouz, S., Canu, S., and Meriaudeau, F. (2019).
Road scenes analysis in adverse weather conditions by
polarization-encoded images and adapted deep learn-
ing. In 2019 IEEE Intelligent Transportation Systems
Conference (ITSC), pages 27–32. IEEE.
Blin, R., Ainouz, S., Canu, S., and Meriaudeau, F. (2020).
A new multimodal rgb and polarimetric image dataset
for road scenes analysis. In Proceedings of the
IEEE/CVF Conference on Computer Vision and Pat-
tern Recognition Workshops, pages 216–217.
Bodla, N., Singh, B., Chellappa, R., and Davis, L. S. (2017).
Soft-nms–improving object detection with one line of
code. In Proceedings of the IEEE international con-
ference on computer vision, pages 5561–5569.
Fan, W., Ainouz, S., Meriaudeau, F., and Bensrhair, A.
(2018). Polarization-based car detection. In 2018 25th
IEEE International Conference on Image Processing
(ICIP), pages 3069–3073. IEEE.
Felzenszwalb, P., McAllester, D., and Ramanan, D. (2008).
A discriminatively trained, multiscale, deformable
part model. In 2008 IEEE Conference on Computer
Vision and Pattern Recognition, pages 1–8. IEEE.
Feng, D., Haase-Schütz, C., Rosenbaum, L., Hertlein,
H., Glaeser, C., Timm, F., Wiesbeck, W., and Diet-
mayer, K. (2020). Deep multi-modal object detection
and semantic segmentation for autonomous driving:
Datasets, methods, and challenges. IEEE Transac-
tions on Intelligent Transportation Systems.
Gu, S., Lu, T., Zhang, Y., Alvarez, J. M., Yang, J., and
Kong, H. (2018). 3-d lidar+ monocular camera:
An inverse-depth-induced fusion framework for urban
road detection. IEEE Transactions on Intelligent Ve-
hicles, 3(3):351–360.
He, K., Zhang, X., Ren, S., and Sun, J. (2016). Deep resid-
ual learning for image recognition. In Proceedings of
the IEEE conference on computer vision and pattern
recognition, pages 770–778.
Kingma, D. P. and Ba, J. (2014). Adam: A
method for stochastic optimization. arXiv preprint
arXiv:1412.6980.
Lin, T.-Y., Goyal, P., Girshick, R., He, K., and Dollár, P.
(2017). Focal loss for dense object detection. In
Proceedings of the IEEE international conference on
computer vision, pages 2980–2988.
Major, B., Fontijne, D., Ansari, A., Teja Sukhavasi, R.,
Gowaikar, R., Hamilton, M., Lee, S., Grzechnik,
S., and Subramanian, S. (2019). Vehicle detec-
tion with automotive radar using deep learning on
range-azimuth-doppler tensors. In Proceedings of the
IEEE/CVF International Conference on Computer Vi-
sion (ICCV) Workshops.
Nie, J., Yan, J., Yin, H., Ren, L., and Meng, Q. (2020). A
multimodality fusion deep neural network and safety
test strategy for intelligent vehicles. IEEE Transac-
tions on Intelligent Vehicles, pages 1–1.
Pinchon, N., Cassignol, O., Nicolas, A., Bernardin, F.,
Leduc, P., Tarel, J.-P., Brémond, R., Bercier, E., and
Brunet, J. (2018). All-weather vision for automotive
safety: which spectral band? In International Forum
on Advanced Microsystems for Automotive Applica-
tions, pages 3–15. Springer.
Rashed, H., Ramzy, M., Vaquero, V., El Sallab, A., Sistu,
G., and Yogamani, S. (2019). Fusemodnet: Real-time
camera and lidar based moving object detection for ro-
bust low-light autonomous driving. In Proceedings of
the IEEE/CVF International Conference on Computer
Vision (ICCV) Workshops.
Yu, F., Chen, H., Wang, X., Xian, W., Chen, Y., Liu, F.,
Madhavan, V., and Darrell, T. (2020). Bdd100k: A
diverse driving dataset for heterogeneous multitask
learning. In Proceedings of the IEEE/CVF conference
on computer vision and pattern recognition, pages
2636–2645.
VISAPP 2022 - 17th International Conference on Computer Vision Theory and Applications
244