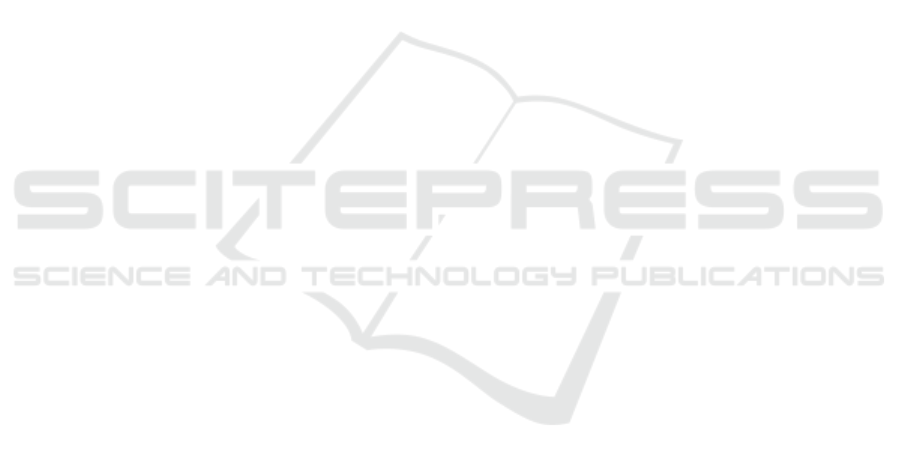
avoiding symbolic representation (i.e., numbers) and
metric rendering, like length extensions and angles.
In vague visualizations, these quantities are rendered
in terms of visual clues that are generally hard to in-
terpret in quantitative terms (see, e.g. (Cleveland and
McGill, 1984)), that is are hard to be mapped into
clear-cut categories of numbers, like color shading
or saturation and brightness gradients. This feature,
which in other contexts could be misinterpreted as a
bug or defect, is purposely intended to convey to the
readers an embodied sense of uncertainty and vague-
ness as a strategy to have readers actually understand
the visualized estimates, such as risks, odds, disper-
sion, not just look at them in abstract terms. For
this reason, vague visualizations require additional
attention (with respect to traditional visualizations)
and must be assessed on the basis of the extent they
suggest correct interpretations without making use of
numbers or visual elements that can be easily con-
verted into numerical values (such as linear exten-
sions or points in Cartesian planes).
2 METHODS
As mentioned above, for this user study we conceived
and designed two data visualizations. These two data
visualizations were conceived during two participa-
tory design sessions that involved the authors of this
article and the clinicians involved in the development
of the statistical model presented in (Cabitza et al.,
2021). Before starting the sessions, the clinicians had
been introduced to the requirements of the vague visu-
alizations framework mentioned above and were in-
vited to co-design a visualization that could better fit
their colleagues, that is experts in interpreting labo-
ratory tests, and a simpler visualization that could be
more familiar to the tested patients.
The resulting visualizations were based on differ-
ent metaphors: one visualization (depicted in Fig-
ure 1) was based on the litmus test, that is a com-
mon test for acidity that is familiar to any chemistry
student, and the bubble level metaphors, which was
chosen to more precisely denote the probabilistic out-
come of the test, while not relying on any number (see
Figures 1 and 2).
The second data visualization (see Figure 3)
adopted the test stick metaphor (see Figures 3 and 4),
widely adopted in, e.g., pregnancy tests, and thus fa-
miliar to the general public.
The user study was then conceived to understand:
1) if the test stick metaphor, as an apparently straight-
forward and common way to present test results, was
adequate in case of a delicate response like the one re-
garding COVID-19 positivity, or, as observed in some
studies (Pike et al., 2013), it would end up by mis-
leading lay people too often. And 2) if a more techni-
cal data visualization, the one designed for healthcare
practitioners, could be understandable also by non-
specialist users.
In the bubble level visualization the test result is
mainly rendered in terms of the position of a circular
bubble within a three-color (litmus alike) bar, that is
in terms of its proximity to one of two bar extremes
to indicate either a COVID-19-positive or a negative
condition (on the leftmost red extreme, and on the
rightmost blue extreme, respectively). Uncertain (i.e.,
low reliability) results are thus indicated in terms of
a substantial equidistance of the bubble from the ex-
tremal anchors, that is when this indicator is in the
middle grey area of the litmus bar. Uncertainty is also
rendered in terms of the size of the bubble, as in a
reinforcing affordance: the bigger the bubble is, the
greater the confidence interval of the probability esti-
mate.
The test stick visualization renders the same in-
formation displayed by the bubble level visualization,
but through different affordances and visual cues. To
this aim, this visualization exploits the visibility of
two red bands: one to indicate the reliability of the re-
sponse and denoted with a capital C (“control”); and
one indicating the result of the test, denoted with a
single plus mark (+). In other words, this visualiza-
tion renders the model output in terms of bar opacity:
so that the more transparent (and less visible) the +
and C bands, the lower the probability that the test
is associated with a positive condition and the over-
all test reliability, respectively (see Figures 3 and 4).
An almost certainly negative test is then rendered by
a stick where only the C bar is clearly visible, while
an invalid test is represented by a stick where no red
bands is visible.
2.1 Visualization Assessment
We assess the above data visualizations in terms of
information effectiveness, that is in terms of their ca-
pability not to mislead the reader, and therefore al-
low them to correctly interpret the displayed informa-
tion, both in regard to the test result and its reliability.
Therefore, we related this dimension to the error rate
detected in a user study where respondents were sup-
posed to read two test results, one associated to high
reliability and a clear response (see Figures 2, 3) and
the other one associated to a border-line case and a
low-reliability test (see Figures 1, 4); and then choose
one answer among several alternatives to report what
they read on the data visualization.
IVAPP 2022 - 13th International Conference on Information Visualization Theory and Applications
196