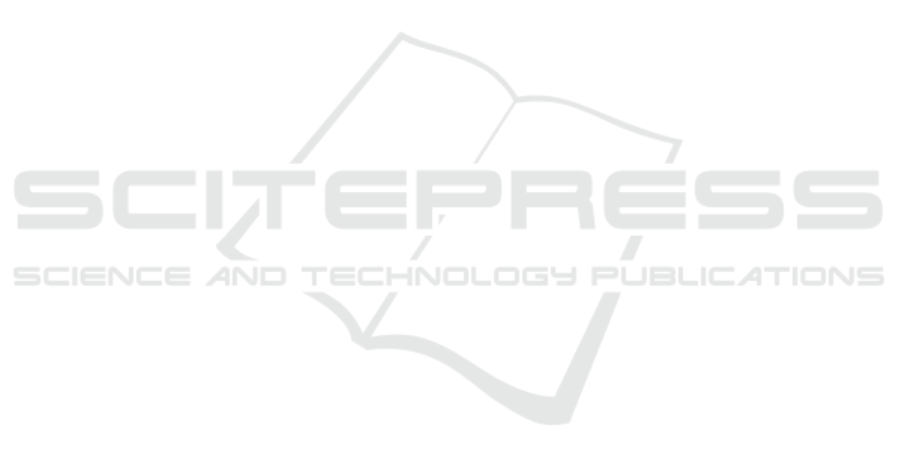
Understanding. Computing Research Repository,
abs/1702.01105.
Blaga, A., Militaru, C., Mezei, A.-D., and Tamas, L. (2021).
Augmented reality integration into mes for connected
workers. Robotics and Computer-Integrated Manu-
facturing, 68:102057.
Fooladgar, F. and Kasaei, S. (2020). A survey on indoor
RGB-D semantic segmentation: from hand-crafted
features to deep convolutional neural networks. Mul-
timedia Tools and Applications, 79(7):4499–4524.
Frohlich, R., Tamas, L., and Kato, Z. (2021). Absolute pose
estimation of central cameras using planar regions.
IEEE Transactions on Pattern Analysis and Machine
Intelligence, 43(2):377–391.
Ioffe, S. and Szegedy, C. (2015). Batch normalization: Ac-
celerating deep network training by reducing internal
covariate shift. In International conference on ma-
chine learning, pages 448–456. PMLR.
Jiao, Y. and Yin, Z. (2020). A Two-Phase Cross-Modality
Fusion Network for Robust 3D Object Detection. Sen-
sors, 20(21).
Le, T. and Duan, Y. (2018). PointGrid: A Deep Net-
work for 3D Shape Understanding. Proceedings of
the IEEE Computer Society Conference on Computer
Vision and Pattern Recognition, pages 9204–9214.
Liu, Z., Tang, H., Lin, Y., and Han, S. (2019). Point-Voxel
CNN for Efficient 3D Deep Learning. In Conference
on Neural Information Processing Systems (NeurIPS).
Maas, A. L., Hannun, A. Y., and Ng, A. Y. (2013). Rec-
tifier nonlinearities improve neural network acoustic
models. In in ICML Workshop on Deep Learning for
Audio, Speech and Language Processing.
Nathan Silberman, Derek Hoiem, P. K. and Fergus, R.
(2012). Indoor Segmentation and Support Inference
from RGBD Images. In ECCV.
Oleksiienko, I. and Iosifidis, A. (2021). Analysis of voxel-
based 3D object detection methods efficiency for real-
time embedded systems.
Ophoff, T., Van Beeck, K., and Goedem
´
e, T. (2019). Ex-
ploring RGB+depth fusion for real-time object detec-
tion. Sensors (Switzerland), 19(4).
Qi, C. R., Su, H., Mo, K., and Guibas, L. J. (2017a). Point-
net: Deep learning on point sets for 3d classification
and segmentation. In Proceedings of the IEEE Con-
ference on Computer Vision and Pattern Recognition
(CVPR).
Qi, C. R., Yi, L., Su, H., and Guibas, L. J. (2017b). Point-
net++: Deep hierarchical feature learning on point sets
in a metric space. Computing Research Repository,
abs/1706.02413.
Ronneberger, O., Fischer, P., and Brox, T. (2015). U-
Net: Convolutional networks for biomedical image
segmentation. In International Conference on Medi-
cal image computing and computer-assisted interven-
tion, pages 234–241. Springer.
Shi, S., Jiang, L., Deng, J., Wang, Z., Guo, C., Shi, J., Wang,
X., and Li, H. (2021). PV-RCNN++: Point-Voxel Fea-
ture Set Abstraction With Local Vector Representation
for 3D Object Detection. pages 1–17.
Tamas, L. and Cozma, A. (2021). Embedded real-time peo-
ple detection and tracking with time-of-flight camera.
In Proc. of SPIE Vol, volume 11736, page 117360B.
Tamas, L. and Goron, L. C. (2014). 3D semantic inter-
pretation for robot perception inside office environ-
ments. Engineering Applications of Artificial Intel-
ligence, 32:76–87.
Tamas, L. and Jensen, B. (2014). Robustness analysis of 3d
feature descriptors for object recognition using a time-
of-flight camera. In 22nd Mediterranean Conference
on Control and Automation, pages 1020–1025. IEEE.
Wu, Z., Song, S., Khosla, A., Yu, F., Zhang, L., Tang, X.,
and Xiao, J. (2015). 3D ShapeNets: A deep repre-
sentation for volumetric shapes. In IEEE Conference
on Computer Vision and Pattern Recognition (CVPR)
oral presentation.
Xu, Y., Tong, X., and Stilla, U. (2021). Voxel-based rep-
resentation of 3d point clouds: Methods, applications,
and its potential use in the construction industry. Au-
tomation in Construction, 126:103675.
Yi, L., Kim, V. G., Ceylan, D., Shen, I.-C., Yan, M., Su, H.,
Lu, C., Huang, Q., Sheffer, A., and Guibas, L. (2016).
A scalable active framework for region annotation in
3d shape collections. SIGGRAPH Asia.
Zhao, H., Jiang, L., Jia, J., Torr, P. H., and Koltun, V. (2021).
Point transformer. In Proceedings of the IEEE/CVF
International Conference on Computer Vision (ICCV),
pages 16259–16268.
VISAPP 2022 - 17th International Conference on Computer Vision Theory and Applications
856