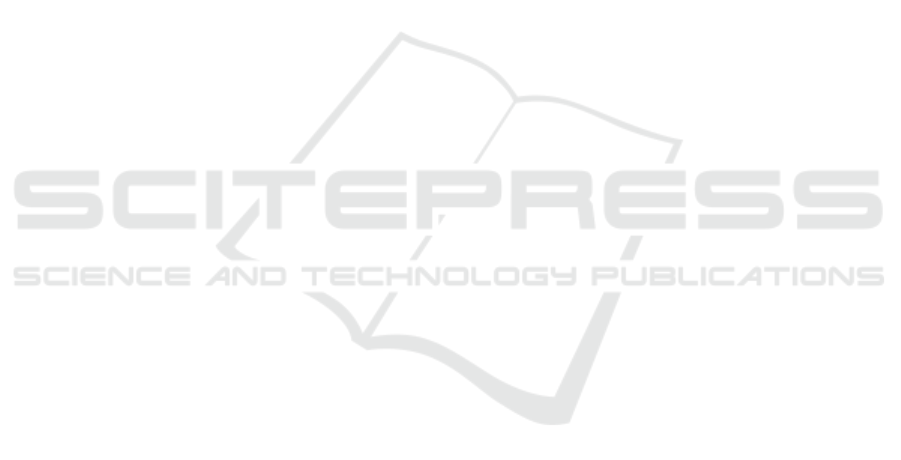
ple. While the results of the experiments leave room
for improvement, especially in terms of the sound
classification, we are confident that future work can
build on this and improve the performance, for exam-
ple by using Ambisonics microphones.
REFERENCES
Bach-y Rita, P. and Kercel, W. S. (2003). Sensory sub-
stitution and the human-machine interface. Trends in
cognitive sciences, 7(12):541–546.
Berra, S., Pernencar, C., and Almeida, F. (2020). Silent
augmented narratives: Inclusive communication with
augmented reality for deaf and hard of hearing. Media
& Jornalismo, 20(36):171–189.
Cie
´
sla, K., Wolak, T., Lorens, A., Heimler, B., Skar
˙
zy
´
nski,
H., and Amedi, A. (2019). Immediate improvement
of speech-in-noise perception through multisensory
stimulation via an auditory to tactile sensory sub-
stitution. Restorative neurology and neuroscience,
37(2):155–166.
Deroy, O. and Auvrey, M. (2012). Reading the World
through the Skin and Ears: A New Perspective on Sen-
sory Substitution. Frontiers in Psychology, 3:457.
Devlin, J., Chang, M.-W., Lee, K., and Toutanova, K.
(2019). BERT: Pre-training of deep bidirectional
transformers for language understanding. In Proceed-
ings of the 2019 Conference of the North American
Chapter of the Association for Computational Lin-
guistics: Human Language Technologies (NAACL),
Volume 1 (Long and Short Papers), pages 4171–4186,
Minneapolis, Minnesota. Association for Computa-
tional Linguistics.
Eckert, M., Blex, M., and Friedrich, C. M. (2018). Ob-
ject Detection Featuring 3D Audio Localization for
Microsoft HoloLens - A Deep Learning based Sen-
sor Substitution Approach for the Blind. In Proceed-
ings of the 11th International Joint Conference on
Biomedical Engineering Systems and Technologies,
pages 555–561. SCITEPRESS - Science and Technol-
ogy Publications.
Gemmeke, J. F., Ellis, D. P. W., Freedman, D., Jansen,
A., Lawrence, W., Moore, R. C., Plakal, M., and Rit-
ter, M. (2017). Audio Set: An ontology and human-
labeled dataset for audio events. In Proceedings of the
IEEE International Conference on Acoustics, Speech
and Signal Processing (ICASSP 2017), pages 776–
780.
Gong, Y., Chung, Y.-A., and Glass, J. (2021). AST: Audio
Spectrogram Transformer. In Proc. Interspeech 2021,
pages 571–575.
Grinberg, M. (2018). Flask Web Development. O’Reilly
Media, Inc, 2nd edition.
Kong, Q., Cao, Y., Iqbal, T., Wang, Y., Wang, W., and
Plumbley, M. D. (2020). Panns: Large-scale pre-
trained audio neural networks for audio pattern recog-
nition. IEEE/ACM Transactions on Audio, Speech,
and Language Processing, 28:2880–2894.
Krizhevsky, A., Sutskever, I., and Hinton, G. E. (2012).
Imagenet classification with deep convolutional neu-
ral networks. In Proceedings of the 25th Interna-
tional Conference on Neural Information Processing
Systems - Volume 1, NIPS’12, page 1097–1105, Red
Hook, NY, USA. Curran Associates Inc.
Kumar, A., Khadkevich, M., and F
¨
ugen, C. (2018). Knowl-
edge transfer from weakly labeled audio using convo-
lutional neural network for sound events and scenes.
In Proceedings of the IEEE International Conference
on Acoustics, Speech and Signal Processing (ICASSP
2018), pages 326–330. IEEE.
Mehra, R., Brimijoin, O., Robinson, P., and Lunner, T.
(2020). Potential of augmented reality platforms to
improve individual hearing aids and to support more
ecologically valid research. Ear and hearing, 41
Suppl 1:140S–146S.
Mense, E. (2020). Sound classification and direction deter-
mination with an Android App. Bachelor thesis, De-
partment of Computer Science, University of Applied
Sciences and Arts Dortmund, Germany.
Nguyen, T. N. T., Watcharasupat, K. N., Lee, Z. J., Nguyen,
N. K., Jones, D. L., and Gan, W. S. (2021a). What
makes sound event localization and detection diffi-
cult? insights from error analysis. In Proceed-
ings of the Detection and Classification of Acous-
tic Scenes and Events 2021 Workshop (DCASE2021),
pages 120–124, Barcelona, Spain.
Nguyen, T. N. T., Watcharasupat, K. N., Nguyen, N. K.,
Jones, D. L., and Gan, W. (2021b). DCASE 2021
task 3: Spectrotemporally-aligned features for poly-
phonic sound event localization and detection. ArXiv,
abs/2106.15190.
Piczak, K. J. (2015). ESC: Dataset for environmental sound
classification. In Zhou, X., Smeaton, A. F., Tian, Q.,
Bulterman, D. C., Shen, H. T., Mayer-Patel, K., and
Yan, S., editors, Proceedings of the 23rd ACM inter-
national conference on Multimedia - MM ’15, pages
1015–1018, New York, New York, USA. ACM Press.
Politis, A., Adavanne, S., Krause, D., Deleforge, A., Sri-
vastava, P., and Virtanen, T. (2021). A Dataset of
Dynamic Reverberant Sound Scenes with Directional
Interferers for Sound Event Localization and Detec-
tion. In Proceedings of the 6th Detection and Classifi-
cation of Acoustic Scenes and Events 2021 Workshop
(DCASE2021), pages 125–129, Barcelona, Spain.
Slaney, M., Lyon, R. F., Garcia, R., Kemler, B., Gnegy, C.,
Wilson, K., Kanevsky, D., Savla, S., and Cerf, V. G.
(2020). Auditory measures for the next billion users.
Ear and hearing, 41 Suppl 1:131S–139S.
Classification and Direction Detection of Ambient Sounds on Microsoft HoloLens to Support Hearing-impaired People
863