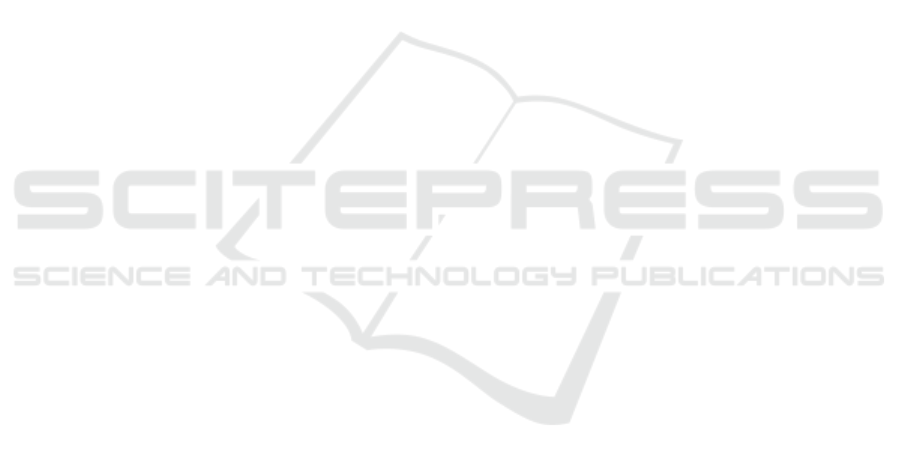
ACM SIGKDD International Conference on Knowl-
edge Discovery and Data Mining, pages 215–223,
Halifax NS Canada. ACM.
Hasida, K. (2014). Personal life repository as a distributed
PDS and its dissemination strategy for healthcare ser-
vices. In AAAI Spring Symposia.
Husebo, B. S., Heintz, H. L., Berge, L. I., Owoyemi, P.,
Rahman, A. T., and Vahia, I. V. (2020). Sensing
Technology to Monitor Behavioral and Psychological
Symptoms and to Assess Treatment Response in Peo-
ple With Dementia. A Systematic Review. Frontiers
in Pharmacology, 10:1699.
Kapoor, V., Singh, R., Reddy, R., and Churi, P. (2020). Pri-
vacy Issues in Wearable Technology: An Intrinsic Re-
view. SSRN Electronic Journal.
Karakostas, A., Briassouli, A., Avgerinakis, K., Kompat-
siaris, I., and Tsolaki, M. (2016). The Dem@Care
experiments and datasets: A technical report.
Karlekar, S., Niu, T., and Bansal, M. (2018). Detecting
Linguistic Characteristics of Alzheimer’s Dementia
by Interpreting Neural Models. In Proceedings of
the 2018 Conference of the North American Chap-
ter of the Association for Computational Linguistics:
Human Language Technologies, Volume 2 (Short Pa-
pers), pages 701–707, New Orleans, Louisiana. Asso-
ciation for Computational Linguistics.
Kelly, M. E., Duff, H., Kelly, S., McHugh Power, J. E.,
Brennan, S., Lawlor, B. A., and Loughrey, D. G.
(2017). The impact of social activities, social net-
works, social support and social relationships on the
cognitive functioning of healthy older adults: A sys-
tematic review. Systematic Reviews, 6(1):259.
Kong, W., Jang, H., and Carenini, G. (2019). A Neural
Model for Predicting Dementia from Language. In
Machine Learning for Healthcare Conference., pages
270–286.
Kourtis, L. C., Regele, O. B., Wright, J. M., and Jones, G. B.
(2019). Digital biomarkers for Alzheimer’s disease:
The mobile/wearable devices opportunity. npj Digital
Medicine, 2(1):9.
Last, B. S., Buttenheim, A. M., Timon, C. E., Mitra, N., and
Beidas, R. S. (2021). Systematic review of clinician-
directed nudges in healthcare contexts. BMJ Open,
11(7):e048801.
Li, J., Li, H., Umer, W., Wang, H., Xing, X., Zhao, S.,
and Hou, J. (2020). Identification and classification
of construction equipment operators’ mental fatigue
using wearable eye-tracking technology. Automation
in Construction, 109:103000.
Li, J., Rong, Y., Meng, H., Lu, Z., Kwok, T., and Cheng,
H. (2018). TATC: Predicting Alzheimer’s Disease
with Actigraphy Data. In Proceedings of the 24th
ACM SIGKDD International Conference on Knowl-
edge Discovery & Data Mining, pages 509–518, Lon-
don United Kingdom. ACM.
Lindbergh, C. A., Dishman, R. K., and Miller, L. S. (2016).
Functional Disability in Mild Cognitive Impairment:
A Systematic Review and Meta-Analysis. Neuropsy-
chology Review, 26(2):129–159.
Liu, Z. X., Liu, Y., and Gao, X. (2019). Using Mobile Eye
Tracking to Evaluate the Satisfaction with Service Of-
fice. In Marcus, A. and Wang, W., editors, Design,
User Experience, and Usability. Practice and Case
Studies, pages 183–195, Cham. Springer International
Publishing.
Luz, S., Haider, F., de la Fuente, S., Fromm, D.,
and MacWhinney, B. (2020). Alzheimer’s Demen-
tia Recognition through Spontaneous Speech: The
ADReSS Challenge. arXiv:2004.06833 [cs, eess,
stat].
Maher, N. A., Senders, J. T., Hulsbergen, A. F., Lamba, N.,
Parker, M., Onnela, J.-P., Bredenoord, A. L., Smith,
T. R., and Broekman, M. L. (2019). Passive data col-
lection and use in healthcare: A systematic review of
ethical issues. International Journal of Medical Infor-
matics, 129:242–247.
Marandi, R. Z. and Gazerani, P. (2019). Aging and eye
tracking: In the quest for objective biomarkers. Future
Neurology, 14(4):FNL33.
Mc Ardle, R., Del Din, S., Galna, B., Thomas, A., and
Rochester, L. (2020). Differentiating dementia dis-
ease subtypes with gait analysis: Feasibility of wear-
able sensors? Gait & Posture, 76:372–376.
Mc Ardle, R., Morris, R., Hickey, A., Del Din, S., Koychev,
I., Gunn, R. N., Lawson, J., Zamboni, G., Ridha, B.,
Sahakian, B., Rowe, J., Thomas, A., Zetterberg, H.,
MacKay, C., Lovestone, S., Rochesteron, L., Team (,
D., and Study, F. P. (2018). Gait in Mild Alzheimer’s
Disease: Feasibility of Multi-Center Measurement in
the Clinic and Home with Body-Worn Sensors: A Pi-
lot Study.
Najar, J.,
¨
Ostling, S., Gudmundsson, P., Sundh, V., Johans-
son, L., Kern, S., Guo, X., H
¨
allstr
¨
om, T., and Skoog, I.
(2019). Cognitive and physical activity and dementia:
A 44-year longitudinal population study of women.
Neurology, 92(12):e1322–e1330.
Okada, S., Inoue, K., Imai, T., Noguchi, M., and Kuwa-
mura, K. (2019). Dementia Scale Classification Based
on Ubiquitous Daily Activity and Interaction Sensing.
8th International Conference on Affective Computing
and Intelligent Interaction (ACII), page 7.
Orimaye, S. O., Wong, J. S.-M., and Wong, C. P. (2018).
Deep language space neural network for classifying
mild cognitive impairment and Alzheimer-type de-
mentia. PLOS ONE, 13(11):e0205636.
Pan, Y., Mirheidari, B., Reuber, M., Venneri, A., Blackburn,
D., and Christensen, H. (2019). Automatic Hierarchi-
cal Attention Neural Network for Detecting AD. In
Interspeech 2019, pages 4105–4109. ISCA.
Pavisic, I. M., Firth, N. C., Parsons, S., Rego, D. M., Shake-
speare, T. J., Yong, K. X. X., Slattery, C. F., Pa-
terson, R. W., Foulkes, A. J. M., Macpherson, K.,
Carton, A. M., Alexander, D. C., Shawe-Taylor, J.,
Fox, N. C., Schott, J. M., Crutch, S. J., and Prima-
tivo, S. (2017). Eyetracking Metrics in Young Onset
Alzheimer’s Disease: A Window into Cognitive Vi-
sual Functions. Frontiers in Neurology, 8:377.
Peelle, J. E. and Grossman, M. (2008). Language Pro-
cessing in Frontotemporal Dementia: A Brief Review.
Language and Linguistics Compass, 2(1):18–35.
Piau, A., Wild, K., Mattek, N., and Kaye, J. (2019). Cur-
rent State of Digital Biomarker Technologies for Real-
Life, Home-Based Monitoring of Cognitive Function
for Mild Cognitive Impairment to Mild Alzheimer
WHC 2022 - Special Session on Wearable HealthCare
870