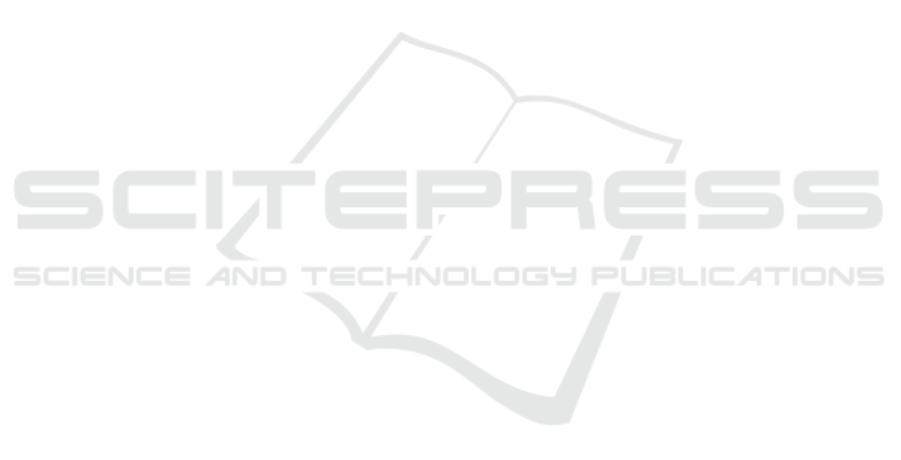
6 CONCLUSIONS
In conclusion, our work shows that machine learning
is capable of making a short-term prediction of the
OGTT glucose values. The outcomes of the study
may provide useful support to health care providers
in early detection of diabetes, making more informed
decisions for the prevention of serious consequences
and overall management of diabetes.
A limitation of this research paper is its small sam-
ple of historical OGTT data. Hence, to establish a
more accurate and reliable prediction model follow-
up OGTT data should be considered in the analysis.
As future work, we aim to evaluate the perfor-
mance of more regression models like Support Vector
Machine (SVM) and Neural Networks. Furthermore,
our purpose is to investigate the OGTT data from in-
dividuals diagnosed with either diabetes or IGT. Fi-
nally, it would be challenging to study the usefulness
of machine and/or deep learning on the same prob-
lem on elderly individuals, women with gestational
diabetes (de Wit et al., 2019) and, also emphasize the
shape of the OGTT glucose curves since the shape
has been used as a predictor of treatment outcomes
(Jagannathan et al., 2020).
ACKNOWLEDGEMENTS
This work has been partially supported by the
European Union’s H2020 research and innovation
programme SmartWork under grant agreement No
826343, SC1-DTH-03-2018 - Adaptive smart work-
ing and living environments supporting active and
healthy ageing and GATEKEEPER under grant
agreement No 857223, SC1-FA-DTS-2018-2020 -
Smart living homes-whole interventions demonstra-
tor for people at health and social risks.
REFERENCES
Abdul-Ghani, M., DeFronzo, R. A., Del Prato, S., Chilton,
R., Singh, R., and Ryder, R. E. (2017). Cardiovascular
disease and type 2 diabetes: has the dawn of a new era
arrived? Diabetes care, 40(7):813–820.
Alexiou, S., Dritsas, E., Kocsis, O., Moustakas, K., and
Fakotakis, N. (2021). An approach for personalized
continuous glucose prediction with regression trees.
In 2021 6th South-East Europe Design Automation,
Computer Engineering, Computer Networks and So-
cial Media Conference (SEEDA-CECNSM), pages 1–
6. IEEE.
Altuve, M., Perpi
˜
nan, G., Severeyn, E., and Wong, S.
(2016). Comparing glucose and insulin data from
the two-hour oral glucose tolerance test in metabolic
syndrome subjects and marathon runners. In 2016
38th Annual International Conference of the IEEE En-
gineering in Medicine and Biology Society (EMBC),
pages 5290–5293.
Alyass, A., Almgren, P., Akerlund, M., Dushoff, J., Isomaa,
B., Nilsson, P., Tuomi, T., Lyssenko, V., Groop, L.,
and Meyre, D. (2015). Modelling of ogtt curve iden-
tifies 1 h plasma glucose level as a strong predictor of
incident type 2 diabetes: results from two prospective
cohorts. Diabetologia, 58(1):87–97.
Baranowska-Jurkun, A., Matuszewski, W., and Bandurska-
Stankiewicz, E. (2020). Chronic microvascular com-
plications in prediabetic states—an overview. Journal
of Clinical Medicine, 9(10):3289.
Bide, P. and Padalkar, A. (2020). Survey on diabetes mel-
litus and incorporation of big data, machine learning
and iot to mitigate it. In 2020 6th International Con-
ference on Advanced Computing and Communication
Systems (ICACCS), pages 1–10.
Charoentong, P., Naiyanetr, P., and Neatpisanvanit, C.
(2004). Effects of body mass on ogtt-derived insulin
sensitivity indexes in healthy subjects. In 2004 IEEE
Region 10 Conference TENCON 2004., volume B,
pages 446–449 Vol. 2.
de Wit, L., Bos, D., van Rossum, A., van Rijn, B., and
Boers, K. (2019). Repeated oral glucose tolerance
tests in women at risk for gestational diabetes melli-
tus. European Journal of Obstetrics & Gynecology
and Reproductive Biology, 242:79–85.
Denil, M., Matheson, D., and De Freitas, N. (2014). Nar-
rowing the gap: Random forests in theory and in prac-
tice. In International conference on machine learning,
pages 665–673. PMLR.
Edinburgh, R. M., Hengist, A., Smith, H. A., Travers,
R. L., Koumanov, F., Betts, J. A., Thompson,
D., Walhin, J.-P., Wallis, G. A., Hamilton, D. L.,
et al. (2018). Preexercise breakfast ingestion ver-
sus extended overnight fasting increases postpran-
dial glucose flux after exercise in healthy men.
American Journal of Physiology-Endocrinology and
Metabolism, 315(5):E1062–E1074.
Fazakis, N., Kocsis, O., Dritsas, E., Alexiou, S., Fakotakis,
N., and Moustakas, K. (2021). Machine learning tools
for long-term type 2 diabetes risk prediction. IEEE
Access, 9:103737–103757.
Fiorentino, T. V., Marini, M. A., Andreozzi, F., Arturi,
F., Succurro, E., Perticone, M., Sciacqua, A., Hribal,
M. L., Perticone, F., and Sesti, G. (2015). One-
hour postload hyperglycemia is a stronger predic-
tor of type 2 diabetes than impaired fasting glucose.
The Journal of Clinical Endocrinology & Metabolism,
100(10):3744–3751.
Islam, M. S., Belhaouari, S. B., Abdul-Ghani, M., and
Qaraqe, M. K. (2021). Data mining techniques for
prediction of type 2 diabetes leading to cardiovascular
disease. In 2021 IEEE 7th World Forum on Internet of
Things (WF-IoT), pages 321–325.
Jagannathan, R., Neves, J. S., Dorcely, B., Chung, S. T.,
Tamura, K., Rhee, M., and Bergman, M. (2020). The
oral glucose tolerance test: 100 years later. Diabetes,
HEALTHINF 2022 - 15th International Conference on Health Informatics
254