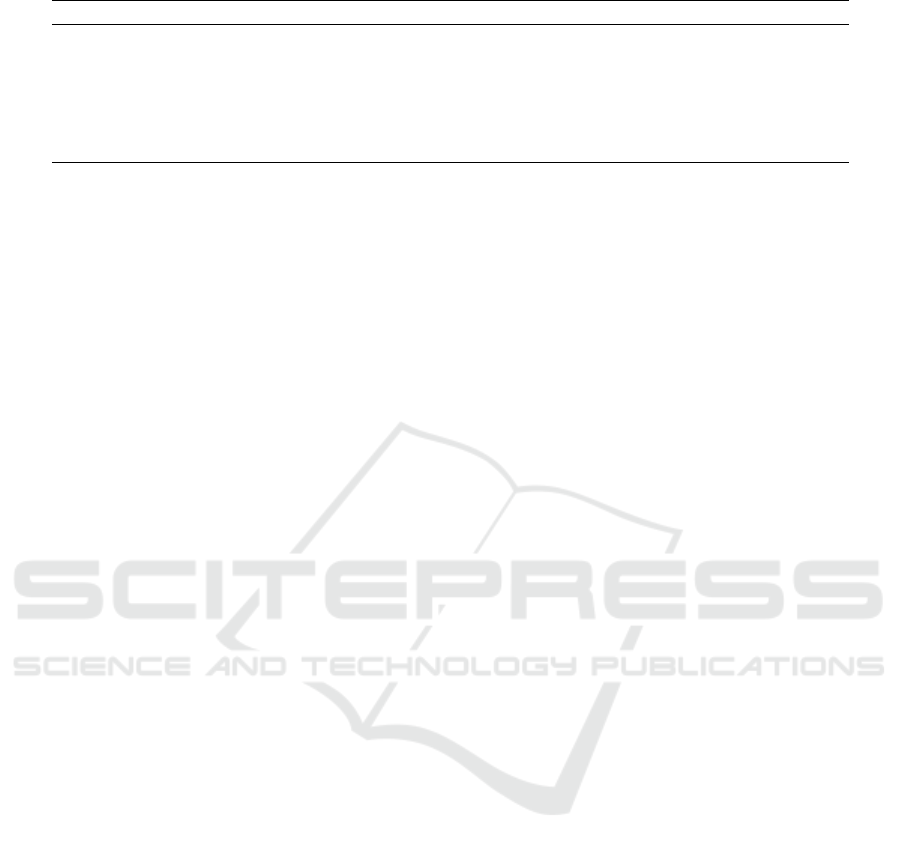
Table 6: The performance of classification model with different z value.
z value Normal cases Abnormal cases Accuracy Auc Recall Precision F1
1 268 226 0.5478 0.5892 0.5914 0.5815 0.5858
1.3 330 164 0.6812 0.6145 0.8707 0.7067 0.7799
1.5 359 135 0.7304 0.6705 0.9145 0.7565 0.8279
1.8 390 104 0.8058 0.7001 0.9814 0.8094 0.8871
2.0 405 89 0.8348 0.6703 0.9857 0.8395 0.9067
2.3 422 72 0.8580 0.6709 0.9966 0.8581 0.9222
and making decisions. Data collection and analysis
beyond the limited dataset we have explored so far is
likely to improve the quality of our algorithms and
lead to new types of insights particularly for long-
term predictions.
REFERENCES
Akram, U., Gardani, M., Riemann, D., Akram, A., Allen,
S. F., Lazuras, L., and Johann, A. F. (2020). Dys-
functional sleep-related cognition and anxiety mediate
the relationship between multidimensional perfection-
ism and insomnia symptoms. Cognitive Processing,
21(1):141–148.
Albanese, A. M., Bartz-Overman, C., Parikh, MD, T.,
and Thielke, S. M. (2020). Associations Between
Activities of Daily Living Independence and Mental
Health Status Among Medicare Managed Care Pa-
tients. Journal of the American Geriatrics Society,
68(6):1301–1306.
Alberdi Aramendi, A., Weakley, A., Aztiria Goenaga, A.,
Schmitter-Edgecombe, M., and Cook, D. J. (2018).
Automatic assessment of functional health decline in
older adults based on smart home data. Journal of
Biomedical Informatics, 81:119–130.
Aminikhanghahi, S., Wang, T., and Cook, D. J. (2019).
Real-Time Change Point Detection with Application
to Smart Home Time Series Data. IEEE Transactions
on Knowledge and Data Engineering, 31(5):1010–
1023.
Anderson, J. L., Rosen, L. N., Mendelson, W. B., Jacobsen,
F. M., Skwerer, R. G., Joseph-Vanderpool, J. R., Dun-
can, C. C., Wehr, T. A., and Rosenthal, N. E. (1994).
Sleep in fall/winter seasonal affective disorder: Ef-
fects of light and changing seasons. Journal of Psy-
chosomatic Research, 38(4):323–337.
Arifoglu, D. and Bouchachia, A. (2019). Detection of ab-
normal behaviour for dementia sufferers using Con-
volutional Neural Networks. Artificial Intelligence in
Medicine, 94:88–95.
Bergmeir, C. and Ben
´
ıtez, J. M. (2012). On the use of cross-
validation for time series predictor evaluation. Infor-
mation Sciences, 191:192–213.
Cohen-Mansfield, J. and Perach, R. (2012). Sleep Duration,
Nap Habits, and Mortality in Older Persons. Sleep,
35(7):1003–1009.
Cook, D. J., Crandall, A. S., Thomas, B. L., and Krish-
nan, N. C. (2013a). CASAS: A Smart Home in a Box.
Computer, 46(7):62–69.
Cook, D. J., Krishnan, N. C., and Rashidi, P. (2013b). Ac-
tivity Discovery and Activity Recognition: A New
Partnership. IEEE Transactions on Cybernetics,
43(3):820–828.
Cook, D. J. and Schmitter-Edgecombe, M. (2021). Fusing
Ambient and Mobile Sensor Features Into a Behav-
iorome for Predicting Clinical Health Scores. IEEE
Access, 9:65033–65043.
Dawadi, P. N., Cook, D. J., and Schmitter-Edgecombe,
M. (2016). Automated Cognitive Health As-
sessment From Smart Home-Based Behavior Data.
IEEE Journal of Biomedical and Health Informatics,
20(4):1188–1194.
Howedi, A., Lotfi, A., and Pourabdollah, A. (2020). An
Entropy-Based Approach for Anomaly Detection in
Activities of Daily Living in the Presence of a Visi-
tor. Entropy, 22(8):845.
Ilyas, I. F. and Chu, X. (2019). Data Cleaning. Association
for Computing Machinery, New York, NY, USA.
Kanti Majumdar, K. (2014). Relationship of Activity of
Daily Living with Quality of Life. British Medical
Bulletin, BBB[2][4][2014]:757–764.
Maclin, R. and Opitz, D. (1999). Popular Ensemble Meth-
ods: An Empirical Study. Journal of Artificial Intelli-
gence Research, 11:169–198.
Palmer, M. G. (2018). Experimenter presence in human be-
havior analytic laboratory studies: Confound it? Be-
havior Analysis: Research and Practice, 19(4):303.
Pazhoumand-Dar, H., Armstrong, L. J., and Tripathy, A. K.
(2020). Detecting deviations from activities of daily
living routines using kinect depth maps and power
consumption data. Journal of Ambient Intelligence
and Humanized Computing, 11(4):1727–1747.
Pedregosa, F., Varoquaux, G., Gramfort, A., Michel, V.,
Thirion, B., Grisel, O., Blondel, M., Prettenhofer, P.,
Weiss, R., Dubourg, V., Vanderplas, J., Passos, A.,
Cournapeau, D., Brucher, M., Perrot, M., and Duch-
esnay,
´
E. (2011). Scikit-learn: Machine Learning
in Python. Journal of Machine Learning Research,
12(85):2825–2830.
Pierleoni, P., Belli, A., Palma, L., Pellegrini, M., Pernini,
L., and Valenti, S. (2015). A High Reliability Wear-
able Device for Elderly Fall Detection. IEEE Sensors
Journal, 15(8):4544–4553.
Piovezan, R. D., Poyares, D., and Tufik, S. (2013). Frailty
and sleep disturbances in the elderly: possible con-
nections and clinical implications. Sleep Science,
6(4):175–179.
Predictive Behavioural Monitoring and Deviation Detection in Activities of Daily Living of Older Adults
909