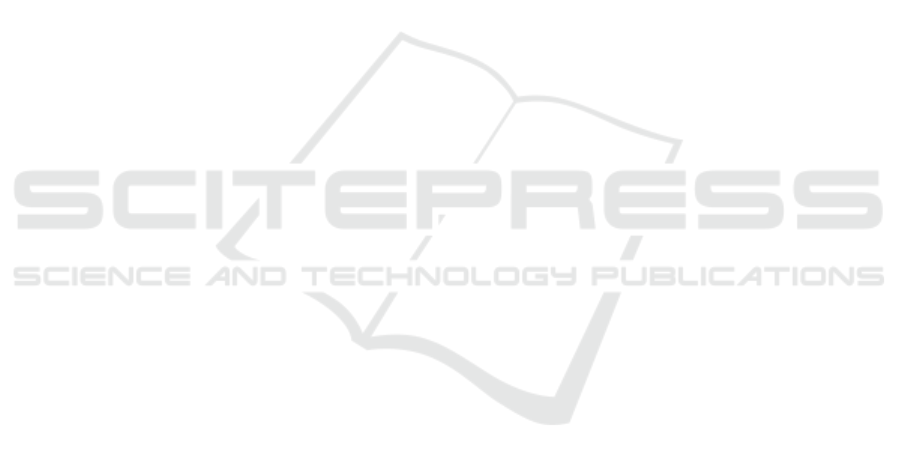
Greco, A., Saggese, A., and Vento, M. (2020b). Digi-
tal signage by real-time gender recognition from face
images. In 2020 IEEE International Workshop on
Metrology for Industry 4.0 & IoT, pages 309–313.
Greco, A., Saggese, A., Vento, M., and Vigilante, V. (2020).
A convolutional neural network for gender recogni-
tion optimizing the accuracy/speed tradeoff. IEEE Ac-
cess, 8:130771–130781.
Greco, A., Saggese, A., Vento, M., and Vigilante, V. (2021).
Performance assessment of face analysis algorithms
with occluded faces. In International Conference on
Pattern Recognition, pages 472–486. Springer.
Gurnani, A., Shah, K., Gajjar, V., Mavani, V., and Khand-
hediya, Y. (2019). Saf-bage: Salient approach for fa-
cial soft-biometric classification-age, gender, and fa-
cial expression. In 2019 IEEE Winter Conference on
Applications of Computer Vision (WACV), pages 839–
847. IEEE.
He, K., Zhang, X., Ren, S., and Sun, J. (2016). Deep resid-
ual learning for image recognition. In 2016 IEEE Con-
ference on Computer Vision and Pattern Recognition
(CVPR), pages 770–778.
Hsu, C.-Y., Lin, L.-E., and Lin, C. (2021). Age and gender
recognition with random occluded data augmentation
on facial images. Multimedia Tools and Applications.
Hu, J., Shen, L., and Sun, G. (2018). Squeeze-and-
excitation networks. In 2018 IEEE/CVF Conference
on Computer Vision and Pattern Recognition, pages
7132–7141.
Kazemi, V. and Sullivan, J. (2014). One millisecond face
alignment with an ensemble of regression trees. In
2014 IEEE Conference on Computer Vision and Pat-
tern Recognition, pages 1867–1874.
Levi, G. and Hassner, T. (2015). Age and gender classifica-
tion using convolutional neural networks. In Proceed-
ings of the IEEE Conference on Computer Vision and
Pattern Recognition Workshops, pages 34–42.
Li, X., Lai, S., and Qian, X. (2021). Dbcface: Towards pure
convolutional neural network face detection. IEEE
Transactions on Circuits and Systems for Video Tech-
nology.
Li, Y., Zeng, J., Shan, S., and Chen, X. (2019). Occlusion
aware facial expression recognition using cnn with at-
tention mechanism. IEEE Transactions on Image Pro-
cessing, 28(5):2439–2450.
Liu, X. and Milanova, M. (2018). Visual attention in deep
learning: a review. In ICRA 2018.
Liu, Y., Gao, Y., and Yin, W. (2020). An improved analysis
of stochastic gradient descent with momentum. arXiv:
Optimization and Control.
Min, R., Hadid, A., and Dugelay, J. (2011). Improving the
recognition of faces occluded by facial accessories.
In 2011 IEEE International Conference on Automatic
Face Gesture Recognition (FG), pages 442–447.
Ng, C.-B., Tay, Y.-H., and Goi, B.-M. (2015). A review of
facial gender recognition. Pattern Analysis and Appli-
cations, 18(4):739–755.
Ranjan, R., Patel, V. M., and Chellappa, R. (2017). Hy-
perface: A deep multi-task learning framework for
face detection, landmark localization, pose estimation,
and gender recognition. IEEE Transactions on Pattern
Analysis and Machine Intelligence, 41(1):121–135.
Roy, A. G., Navab, N., and Wachinger, C. (2018). Concur-
rent spatial and channel squeeze & excitation in fully
convolutional networks. ArXiv, abs/1803.02579.
Sandler, M., Howard, A., Zhu, M., Zhmoginov, A., and
Chen, L.-C. (2018). Mobilenetv2: Inverted residu-
als and linear bottlenecks. In Proceedings of the IEEE
Conference on Computer Vision and Pattern Recogni-
tion, pages 4510–4520.
Selvaraju, R. R., Cogswell, M., Das, A., Vedantam, R.,
Parikh, D., and Batra, D. (2017). Grad-cam: Visual
explanations from deep networks via gradient-based
localization. In 2017 IEEE International Conference
on Computer Vision (ICCV), pages 618–626.
Simanjuntak, F. and Azzopardi, G. (2019). Fusion of cnn-
and cosfire-based features with application to gender
recognition from face images. In Science and Infor-
mation Conference, pages 444–458. Springer.
Wang, Q., Wu, B., Zhu, P., Li, P., Zuo, W., and Hu, Q.
(2020). Eca-net: Efficient channel attention for deep
convolutional neural networks. 2020 IEEE/CVF Con-
ference on Computer Vision and Pattern Recognition
(CVPR), pages 11531–11539.
Woo, S., Park, J., Lee, J.-Y., and Kweon, I.-S. (2018).
Cbam: Convolutional block attention module. In
ECCV.
Xu, X., Sarafianos, N., and Kakadiaris, I. (2020). On im-
proving the generalization of face recognition in the
presence of occlusions. 2020 IEEE/CVF Conference
on Computer Vision and Pattern Recognition Work-
shops (CVPRW), pages 3470–3480.
Yang, S., Luo, P., Loy, C.-C., and Tang, X. (2016). Wider
face: A face detection benchmark. In Proceedings of
the IEEE Conference on Computer Vision and Pattern
Recognition, pages 5525–5533.
Yuan, Z. (2020). Face detection and recognition based on
visual attention mechanism guidance model in unre-
stricted posture. Scientific Programming, 2020:1–10.
yuan Wang, Z., Wang, G., Huang, B., Xiong, Z., Hong, Q.,
Wu, H., Yi, P., Jiang, K., Wang, N., Pei, Y., Chen, H.,
Miao, Y., Huang, Z., and Liang, J. (2020). Masked
face recognition dataset and application. ArXiv,
abs/2003.09093.
Zhang, W., Smith, M. L., Smith, L. N., and Farooq, A.
(2016). Gender and gaze gesture recognition for
human-computer interaction. Computer Vision and
Image Understanding, 149:32–50.
Zhou, B., Khosla, A., Lapedriza, A., Oliva, A., and Tor-
ralba, A. (2016). Learning deep features for discrim-
inative localization. In Proceedings of the IEEE con-
ference on computer vision and pattern recognition,
pages 2921–2929.
VISAPP 2022 - 17th International Conference on Computer Vision Theory and Applications
678