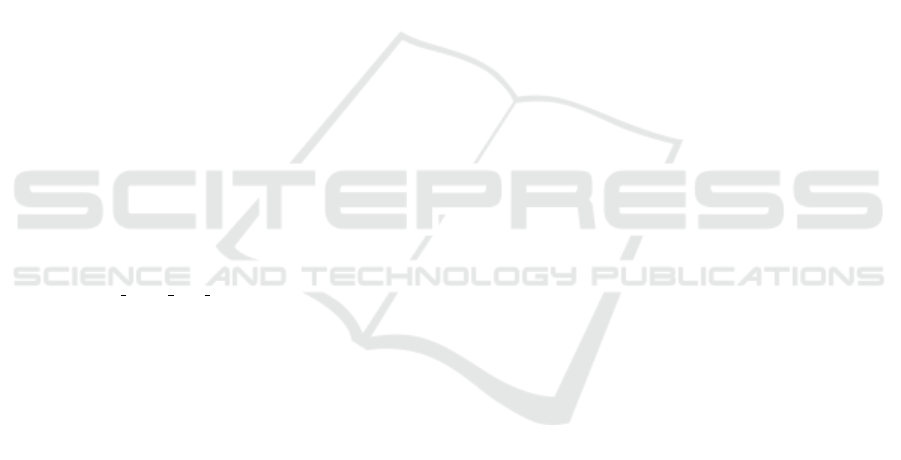
project RFI OIC EXAN. The internship work of
L. Vincent was supported by the FAME research
cluster (Human Factors for Medical Technologies,
NExT/ANR-16-IDEX-0007). All authors would like
to thank F. Dama, PhD student, whose contribution in
the development of the DBLBS generator is invalu-
able. The software development and execution of ex-
periments were carried out at the CCIPL (Centre de
Calcul Intensif des Pays de la Loire, Nantes, France).
REFERENCES
Brown, S.-A. (2016). Patient similarity: emerging concepts
in systems and precision medicine. Frontiers in Phys-
iology, 7:561.
Choudhury, N. and Begum, S. (2016). A survey on case-
based reasoning in medicine. International Jour-
nal of Advanced Computer Science and Applications,
7(8):132–136.
Christ, M., Braun, N., Neuffer, J., and Kempa-Liehr, A.
(2018). Time Series FeatuRe Extraction on basis of
Scalable Hypothesis tests (tsfresh – A Python pack-
age). Neurocomputing, 307:72–77.
Dama, F. and Sinoquet, C. (2021). Partially Hidden Markov
Chain Linear Autoregressive model: inference and
forecasting. arXiv:2102.12584.
Dau, H., Keogh, E., Kamgar, K., Yeh, C.-C., Zhu, Y.,
Gharghabi, S., Ratanamahatana, C., Yanping, Hu,
B., Begum, N., Bagnall, A., Mueen, A., Batista,
G., and Hexagon-ML (2018). The UCR time se-
ries classification archive. https://www.cs.ucr.edu/ ea-
monn/time
series data 2018.
Erdogan, T. and Tarhan, A. (2018). A goal-driven evalu-
ation method based on process mining for healthcare
processes. Applied Sciences, 8(6):894.
Fang, H., Tan, N., Tan, W., Oei, R., Lee, M., and Hsu,
W. (2021). Patient similarity analytics for explainable
clinical risk prediction. BMC Medical Informatics and
Decision Making, 21(1):207.
Forestier, G., Petitjean, F., Riffaud, L., and Jannin, P.
(2017). Automatic matching of surgeries to pre-
dict surgeons’ next actions. Artificial Intelligence in
Medicine, 81:3–11.
Franke, S., Meixensberger, J., and Neumuth, T. (2013).
Intervention time prediction from surgical low-level
tasks. Journal of Biomedical Informatics, 46(1):152–
09.
Ganzinger, M., Schrodt, J., and Knaup-Gregori, P. (2019).
A concept for graph-based temporal similarity of pa-
tient data. Studies in Health Technology and Informat-
ics, 264:138–142.
Gharghabi, S., Imani, S., Bagnall, A., Darvishzadeh, A.,
and Keogh, E. (2018). Matrix Profile XII: MPdist: a
novel time series distance measure to allow data min-
ing in more challenging scenarios. In IEEE Inter-
national Conference on Data Mining (ICDM), pages
965–970.
Goel, A. and Diaz-Agudo, B. (2017). What’s hot in case-
based reasoning. In Thirty-first AAAI Conference on
Artificial Intelligence (AAAI), pages 5067–5069.
Itakura, F. (1975). Minimum prediction residual principle
applied to speech recognition. IEEE Transactions on
Acoustics, Speech, and Signal Processing, 23(1):67–
72.
Khalid, S., Goldenberg, M., Grantcharov, T., Taati, B., and
Rudzicz, F. (2020). Evaluation of Deep Learning
models for identifying surgical actions and measuring
performance. JAMA Network Open 3(3):e201664.
Liu, Z., Zhu, Z., Gao, J., and Xu, C. (2021). Forecast meth-
ods for time series data: a survey. IEEE Access, 606–
617:3091162.
Meißner, C., Meixensberger, J., Pretschner, A., and Neu-
muth, T. (2014). Sensor-based surgical activity recog-
nition in unconstrained environments. Minimally In-
vasive Therapy & Allied Technologies, 23:198–205.
M
¨
ul
ˆ
ayim, M. and Arcos, J. (2020). Fast anytime retrieval
with confidence in large-scale temporal case bases.
Knowledge-Based Systems, 206:106374.
M
¨
uller, M., Mattes, H., and Kurth, F. (2006). An effi-
cient multiscale approach to audio synchronization.
In International Conference on Music Information Re-
trieval (ISMIR), pages 192–197.
Nagendran, M., Gurusamy, K., Aggarwal, R., Loizidou, M.,
and Davidson, B. (2013). Virtual reality training for
surgical trainees in laparoscopic surgery. Cochrane
Database of Systematic Reviews, 8:CD00657.
Ng, K., Sun, J., Hu, J., and Wang, F. (2015). Personal-
ized predictive modeling and risk factor identification
using patient similarity. MIA Joint Summits on Trans-
lational Science proceedings, pages 132–136.
Parimbelli, E., Marini, S., Sacchi, L., and Bellazzi, R.
(2018). Patient similarity for precision medicine: a
systematic review. Journal of Biomedical Informat-
ics, 83:87–96.
Qi, D., Ryason, A., Milef, N., Alfred, S., Abu-Nuwar, M.,
Kappus, M., De, S., and Jones, D. (2021). Virtual
reality operating room with AI guidance: design and
validation of a fire scenario. Surgical Endoscopy,
35(2):779–786.
Sakoe, H. and Chiba, S. (1971). A dynamic programming
approach to continuous speech recognition. In ICA,
Paper 20 CI3.
Sakoe, H. and Chiba, S. (1978). Dynamic programming
algorithm optimization for spoken word recognition.
IEEE Transactions on Acoustics, Speech and Signal
Processing, 26(1):43–49.
Salvador, S. and Chan, P. (2007). Toward accurate dynamic
time warping in linear time and space. Intelligent Data
Analysis, 11(5):561–580.
Sha, Y., Venugopalan, J., and Wang, M. (2016). A novel
temporal similarity measure for patients based on ir-
regularly measured data in electronic health records.
In Seventh ACM International Conference on Bioin-
formatics, Computational Biology, and Health Infor-
matics (BCB), pages 337–344.
Stauder, R., Okur, A., Peter, L., Schneider, A., Kranzfelder,
M., Feussner, H., and Navab, N. (2014). Random
HEALTHINF 2022 - 15th International Conference on Health Informatics
238