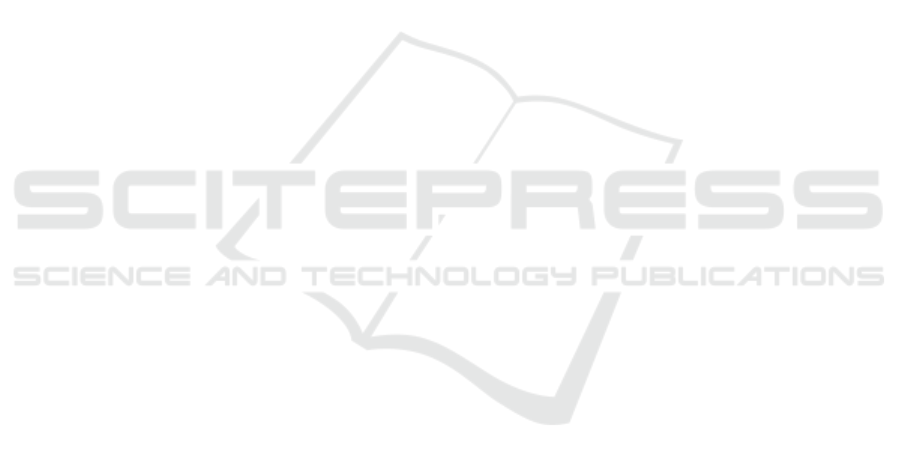
5 CONCLUSIONS
The experimental protocol presented in this paper
takes into consideration many of the sources of varia-
tion encountered in previous studies. Its main purpose
was to induce three states: relaxation, stress and neu-
tral/baseline.
The study was carried out in a constrained envi-
ronment and protocol validation was achieved using
both psychological self-reports and ground truth cor-
tisol levels which makes it reliable for machine learn-
ing algorithms. The dataset includes high-quality
physiological modalities commonly used in commer-
cial and medical devices for stress identification like
ECG, PPG, EDA, EMG and three axis gyroscope
data. Thanks to the high number of participant as
well as their diversity in terms of age and gender, it
is possible to draw reliable conclusions and statistical
generalisation.
The dataset will be made publicly available once
data cleaning and organisation are complete. It could
be used in many different ways to study the correla-
tion between various physiological signals with stress
and/or stress recovery in a uni-modal or multi-modal
approach. It could also be used to compare chest-
based ECG device to earlobe PPG in terms of signal
quality, prep-rocessing, and classification results. The
self-reports could be utilized to create personalised
models able to detect and predict a person’s specific
affective state.
ACKNOWLEDGEMENTS
Authors would like to thank Idex Sorbonne Univer-
sity for funding this experimental study as part of
french state support for ”Investissements d’Avenir’
program”. Also thanks to all the subjects and to IN-
SEAD lab for their expertise in participant recruit-
ment and management which made the process ex-
tremely easier.
ETHICS DECLARATIONS
All volunteers gave their informed written consent in
accordance with the Declaration of Helsinki and fol-
lowing approval from and in accordance with the IN-
SEAD Institutional Review Board (IRB : 202077).
REFERENCES
Adochiei, I., Adochiei, F., Cepisca, C., Seritan, G., Enache,
B., Argatu, F., and Ciucu, R. (2019). Complex em-
bedded system for stress quantification, 2019 (atee).
WOS: 000475904500049.
Alamudun, F., Choi, J., Gutierrez-Osuna, R., Khan, H., and
Ahmed, B. (2012). Removal of subject-dependent and
activity-dependent variation in physiological mea-
sures of stress. In 2012 6th International Conference
on Pervasive Computing Technologies for Healthcare
(PervasiveHealth) and Workshops, pages 115–122.
IEEE.
Bradley, M. M. and Lang, P. J. (1994). Measuring emotion:
the self-assessment manikin and the semantic differ-
ential. Journal of behavior therapy and experimental
psychiatry, 25(1):49–59.
Dalal, K. S., Chellam, S., and Toal, P. (2015). Anaesthesia
information booklet: Is it better than a pre-operative
visit? Indian journal of anaesthesia, 59(8):511.
Dickerson, S. S. and Kemeny, M. E. (2004). Acute stres-
sors and cortisol responses: a theoretical integration
and synthesis of laboratory research. Psychological
bulletin, 130(3):355.
Fink, G. (2016). Chapter 1—stress, definitions, mech-
anisms, and effects outlined: lessons from anxiety.
Stress: Concepts, Cognition, Emotion, and Behavior;
Fink, G., Ed, pages 3–11.
Hart, S. G. and Staveland, L. E. (1988). Development of
nasa-tlx (task load index): Results of empirical and
theoretical research. In Advances in psychology, vol-
ume 52, pages 139–183. Elsevier.
Healey, J. A. and Picard, R. W. (2005). Detecting stress dur-
ing real-world driving tasks using physiological sen-
sors. IEEE Transactions on intelligent transportation
systems, 6(2):156–166.
Kendler, K. S., Hettema, J. M., Butera, F., Gardner, C. O.,
and Prescott, C. A. (2003). Life event dimensions of
loss, humiliation, entrapment, and danger in the pre-
diction of onsets of major depression and generalized
anxiety. Archives of general psychiatry, 60(8):789–
796.
Koelstra, S., Muhl, C., Soleymani, M., Lee, J.-S., Yazdani,
A., Ebrahimi, T., Pun, T., Nijholt, A., and Patras, I.
(2011). Deap: A database for emotion analysis; using
physiological signals. IEEE transactions on affective
computing, 3(1):18–31.
Koldijk, S., Sappelli, M., Verberne, S., Neerincx, M. A., and
Kraaij, W. (2014). The swell knowledge work dataset
for stress and user modeling research. In Proceedings
of the 16th international conference on multimodal in-
teraction, pages 291–298.
Schmidt, P., Reiss, A., Duerichen, R., Marberger, C., and
Van Laerhoven, K. (2018). Introducing wesad, a
multimodal dataset for wearable stress and affect de-
tection. In Proceedings of the 20th ACM interna-
tional conference on multimodal interaction, pages
400–408.
Schneiderman, N., Ironson, G., and Siegel, S. D. (2005).
Stress and health: psychological, behavioral, and bi-
Mmsd: A Multi-modal Dataset for Real-time, Continuous Stress Detection from Physiological Signals
247