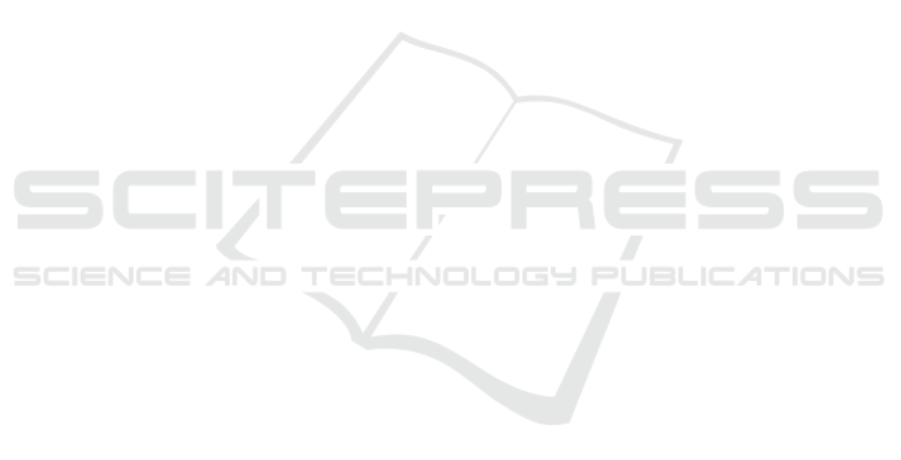
Delbruck, T., Flickner, M., and Modha, D. (2017). A
Low Power, Fully Event-Based Gesture Recognition
System. In Computer Vision and Pattern Recognition
(CVPR), pages 7388–7397, Honolulu, HI. IEEE.
Bi, Y., Chadha, A., Abbas, A., Bourtsoulatze, E., and An-
dreopoulos, Y. (2019). Graph-based object classifica-
tion for neuromorphic vision sensing.
Chin, T.-J., Bagchi, S., Eriksson, A., and Schaik, A. V.
(2019). Star tracking using an event camera. 2019
IEEE/CVF Conference on Computer Vision and Pat-
tern Recognition Workshops (CVPRW).
Cohen, G., Afshar, S., van Schaik, A., Wabnitz, A., Bessell,
T., Rutten, M., and Morreale, B. (2017). Event-based
sensing for space situational awareness.
Cordone, L., Miramond, B., and Ferrante, S. (2021). Learn-
ing from Event Cameras with Sparse Spiking Con-
volutional Neural Networks. In IEEE, editor, Inter-
national Joint Conference On Neural Networks 2021
(IJCNN 2021), page 8, Conf
´
erence virtuelle, China.
Davies, M., Srinivasa, N., Lin, T.-H., Chinya, G., Cao, Y.,
Choday, S. H., Dimou, G., Joshi, P., Imam, N., Jain,
S., Liao, Y., Lin, C.-K., Lines, A., Liu, R., Math-
aikutty, D., McCoy, S., Paul, A., Tse, J., Venkatara-
manan, G., Weng, Y.-H., Wild, A., Yang, Y., and
Wang, H. (2018). Loihi: A neuromorphic many-
core processor with on-chip learning. IEEE Micro,
38(1):82–99.
Fang, W., Yu, Z., Chen, Y., Masquelier, T., Huang, T., and
Tian, Y. (2021). Incorporating learnable membrane
time constant to enhance learning of spiking neural
networks. In International Conference on Computer
Vision (ICCV), pages 2661–2671.
Furber, S. B., Lester, D. R., Plana, L. A., Garside, J. D.,
Painkras, E., Temple, S., and Brown, A. D. (2013).
Overview of the spinnaker system architecture. IEEE
Transactions on Computers, 62(12):2454–2467.
Gallego, G., Delbruck, T., Orchard, G., and al. (2020).
Event-based vision: A survey. IEEE Transactions on
Pattern Analysis and Machine Intelligence.
Gehrig, D., Gehrig, M., Hidalgo-Carri
´
o, J., and Scara-
muzza, D. (2020). Video to events: Recycling video
datasets for event cameras. In IEEE Conf. Comput.
Vis. Pattern Recog. (CVPR).
Glover, A. and Bartolozzi, C. (2016). Event-driven ball de-
tection and gaze fixation in clutter. 2016 IEEE/RSJ In-
ternational Conference on Intelligent Robots and Sys-
tems (IROS).
Gruel, A., Martinet, J., Linares-Barranco, B., and Serrano-
Gotarredona, T. (2021). Stakes of foveation on event
cameras. In ORASIS 2021, Saint Ferr
´
eol, France. Cen-
tre National de la Recherche Scientifique [CNRS].
Huang, C. (2021). Event-based timestamp image encod-
ing network for human action recognition and antic-
ipation. In 2021 International Joint Conference on
Neural Networks (IJCNN), pages 1–9.
Kim, H., Leutenegger, S., and Davison, A. J. (2016). Real-
time 3d reconstruction and 6-dof tracking with an
event camera. Computer Vision – ECCV 2016 Lecture
Notes in Computer Science, page 349–364.
Lagorce, X., Ieng, S.-H., Clady, X., Pfeiffer, M., and Benos-
man, R. (2015). Spatiotemporal features for asyn-
chronous event-based data. Front. Neurosci. - Neu-
romorphic Engineering.
Lenz, G., Chaney, K., Shrestha, S. B., Oubari, O., Pi-
caud, S., and Zarrella, G. (2021). Tonic: event-based
datasets and transformations. Documentation avail-
able under https://tonic.readthedocs.io.
Lichtsteiner, P., Posch, C., and Delbruck, T. (2008). A
128x128 120 db 15 us latency asynchronous tempo-
ral contrast vision sensor. IEEE Journal of Solid-State
Circuits.
Mahowald, M. and Mead, C. (1991). The Silicon Retina.
Sci. American.
Orchard, G., Benosman, R., Etienne-Cummings, R., and
Thakor, N. V. (2013). A spiking neural network ar-
chitecture for visual motion estimation. 2013 IEEE
Biomedical Circuits and Systems Conference (Bio-
CAS).
Paredes-Valles, F., Scheper, K. Y. W., and Croon, G. C. H.
E. D. (2020). Unsupervised learning of a hierarchi-
cal spiking neural network for optical flow estimation:
From events to global motion perception. IEEE Trans-
actions on Pattern Analysis and Machine Intelligence,
42(8):2051–2064.
Rabbath, C. and Corriveau, D. (2019). A comparison
of piecewise cubic hermite interpolating polynomials,
cubic splines and piecewise linear functions for the
approximation of projectile aerodynamics. 31st Inter-
national Symposium on Ballistics.
Rebecq, H., Horstschaefer, T., and Scaramuzza, D. (2017).
Real-time visual-inertial odometry for event cameras
using keyframe-based nonlinear optimization.
Rebecq, H., Ranftl, R., Koltun, V., and Scaramuzza, D.
(2019). Events-to-video: Bringing modern computer
vision to event cameras. IEEE Conf. Comput. Vis. Pat-
tern Recog. (CVPR).
Rebecq, H., Ranftl, R., Koltun, V., and Scaramuzza, D.
(2021). High speed and high dynamic range video
with an event camera. IEEE Transactions on Pattern
Analysis and Machine Intelligence, 43(6):1964–1980.
Vidal, A. R., Rebecq, H., Horstschaefer, T., and Scara-
muzza, D. (2018). Ultimate slam? combining events,
images, and imu for robust visual slam in hdr and
high-speed scenarios. IEEE Robotics and Automation
Letters, 3(2):994–1001.
Zhang, L., Zhang, H., Zhu, C., Guo, S., Chen, J., and Wang,
L. (2021a). Fine-grained video deblurring with event
camera. In Loko
ˇ
c, J., Skopal, T., Schoeffmann, K.,
Mezaris, V., Li, X., Vrochidis, S., and Patras, I., ed-
itors, MultiMedia Modeling, pages 352–364, Cham.
Springer International Publishing.
Zhang, Z., Yezzi, A., and Gallego, G. (2021b). Im-
age reconstruction from events. why learn it?
arXiv:2112.06242.
Event Data Downscaling for Embedded Computer Vision
253