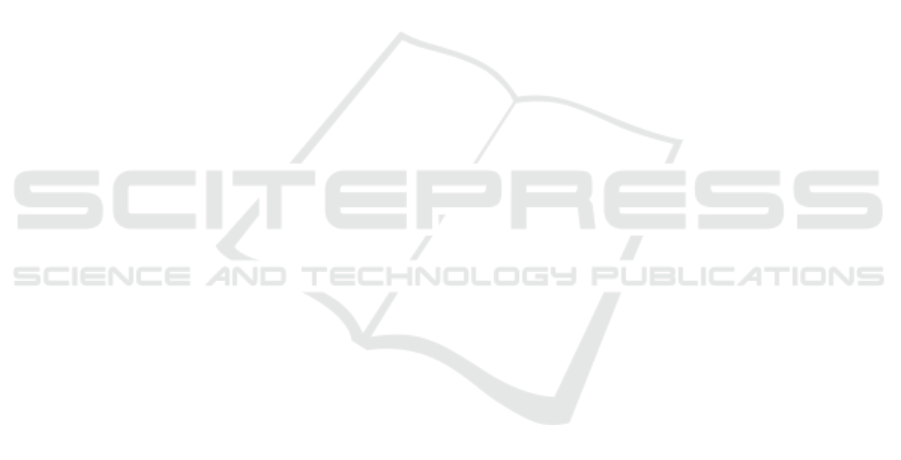
Li, Y., Yang, J., Song, Y., Cao, L., Luo, J., and Li, L.-J.
(2017). Learning from noisy labels with distillation.
In Proceedings of the IEEE International Conference
on Computer Vision, pages 1910–1918.
Liao, Y.-H., Kar, A., and Fidler, S. (2021). Towards
good practices for efficiently annotating large-scale
image classification datasets. In Proceedings of the
IEEE/CVF Conference on Computer Vision and Pat-
tern Recognition, pages 4350–4359.
Liu, S., Niles-Weed, J., Razavian, N., and Fernandez-
Granda, C. (2020). Early-learning regularization pre-
vents memorization of noisy labels. Advances in Neu-
ral Information Processing Systems, 33.
Liu, T. and Tao, D. (2015). Classification with noisy labels
by importance reweighting. IEEE Transactions on
pattern analysis and machine intelligence, 38(3):447–
461.
Ma, X., Huang, H., Wang, Y., Romano, S., Erfani, S., and
Bailey, J. (2020). Normalized loss functions for deep
learning with noisy labels. In International Confer-
ence on Machine Learning, pages 6543–6553. PMLR.
Malach, E. and Shalev-Shwartz, S. (2017). Decoupling”
when to update” from” how to update”. Advances in
Neural Information Processing Systems, 30:960–970.
Mandal, D., Bharadwaj, S., and Biswas, S. (2020). A novel
self-supervised re-labeling approach for training with
noisy labels. In Proceedings of the IEEE/CVF Win-
ter Conference on Applications of Computer Vision,
pages 1381–1390.
Natarajan, N., Dhillon, I. S., Ravikumar, P. K., and Tewari,
A. (2013). Learning with noisy labels. Advances
in neural information processing systems, 26:1196–
1204.
Nigam, N., Dutta, T., and Gupta, H. P. (2020). Impact of
noisy labels in learning techniques: a survey. In Ad-
vances in data and information sciences, pages 403–
411. Springer.
Nishi, K., Ding, Y., Rich, A., and Hollerer, T. (2021). Aug-
mentation strategies for learning with noisy labels. In
Proceedings of the IEEE/CVF Conference on Com-
puter Vision and Pattern Recognition, pages 8022–
8031.
Patrini, G., Rozza, A., Krishna Menon, A., Nock, R., and
Qu, L. (2017). Making deep neural networks robust
to label noise: A loss correction approach. In Pro-
ceedings of the IEEE conference on computer vision
and pattern recognition, pages 1944–1952.
Pham, H., Dai, Z., Xie, Q., and Le, Q. V. (2021). Meta
pseudo labels. In Proceedings of the IEEE/CVF Con-
ference on Computer Vision and Pattern Recognition,
pages 11557–11568.
Sohn, K., Berthelot, D., Li, C.-L., Zhang, Z., Carlini, N.,
Cubuk, E. D., Kurakin, A., Zhang, H., and Raffel, C.
(2020). Fixmatch: Simplifying semi-supervised learn-
ing with consistency and confidence. arXiv preprint
arXiv:2001.07685.
Sun, C., Shrivastava, A., Singh, S., and Gupta, A. (2017).
Revisiting unreasonable effectiveness of data in deep
learning era. In Proceedings of the IEEE international
conference on computer vision, pages 843–852.
Tan, C., Xia, J., Wu, L., and Li, S. Z. (2021). Co-learning:
Learning from noisy labels with self-supervision. In
Proceedings of the 29th ACM International Confer-
ence on Multimedia, pages 1405–1413.
Wang, F. and Liu, H. (2021). Understanding the behaviour
of contrastive loss. In Proceedings of the IEEE/CVF
Conference on Computer Vision and Pattern Recogni-
tion, pages 2495–2504.
Wang, Y., Ma, X., Chen, Z., Luo, Y., Yi, J., and Bailey, J.
(2019). Symmetric cross entropy for robust learning
with noisy labels. In Proceedings of the IEEE/CVF
International Conference on Computer Vision, pages
322–330.
Wu, S., Xia, X., Liu, T., Han, B., Gong, M., Wang, N.,
Liu, H., and Niu, G. (2020). Multi-class classifica-
tion from noisy-similarity-labeled data. arXiv preprint
arXiv:2002.06508.
Wu, S., Xia, X., Liu, T., Han, B., Gong, M., Wang, N., Liu,
H., and Niu, G. (2021). Class2simi: A noise reduc-
tion perspective on learning with noisy labels. In In-
ternational Conference on Machine Learning, pages
11285–11295. PMLR.
Xiao, T., Xia, T., Yang, Y., Huang, C., and Wang, X. (2015).
Learning from massive noisy labeled data for image
classification. In Proceedings of the IEEE conference
on computer vision and pattern recognition, pages
2691–2699.
Yu, X., Han, B., Yao, J., Niu, G., Tsang, I., and Sugiyama,
M. (2019). How does disagreement help generaliza-
tion against label corruption? In International Confer-
ence on Machine Learning, pages 7164–7173. PMLR.
Zhang, C., Bengio, S., Hardt, M., Recht, B., and Vinyals,
O. (2021a). Understanding deep learning (still) re-
quires rethinking generalization. Communications of
the ACM, 64(3):107–115.
Zhang, X., Liu, Z., Xiao, K., Shen, T., Huang, J., Yang, W.,
Samaras, D., and Han, X. (2021b). Codim: Learn-
ing with noisy labels via contrastive semi-supervised
learning. arXiv preprint arXiv:2111.11652.
Zhang, Z. and Pfister, T. (2021). Learning fast sample re-
weighting without reward data. In Proceedings of the
IEEE/CVF International Conference on Computer Vi-
sion, pages 725–734.
Zhang, Z. and Sabuncu, M. R. (2018). Generalized cross
entropy loss for training deep neural networks with
noisy labels. In 32nd Conference on Neural Informa-
tion Processing Systems (NeurIPS).
Zheltonozhskii, E., Baskin, C., Mendelson, A., Bronstein,
A. M., and Litany, O. (2021). Contrast to divide: Self-
supervised pre-training for learning with noisy labels.
arXiv preprint arXiv:2103.13646.
VISAPP 2022 - 17th International Conference on Computer Vision Theory and Applications
686