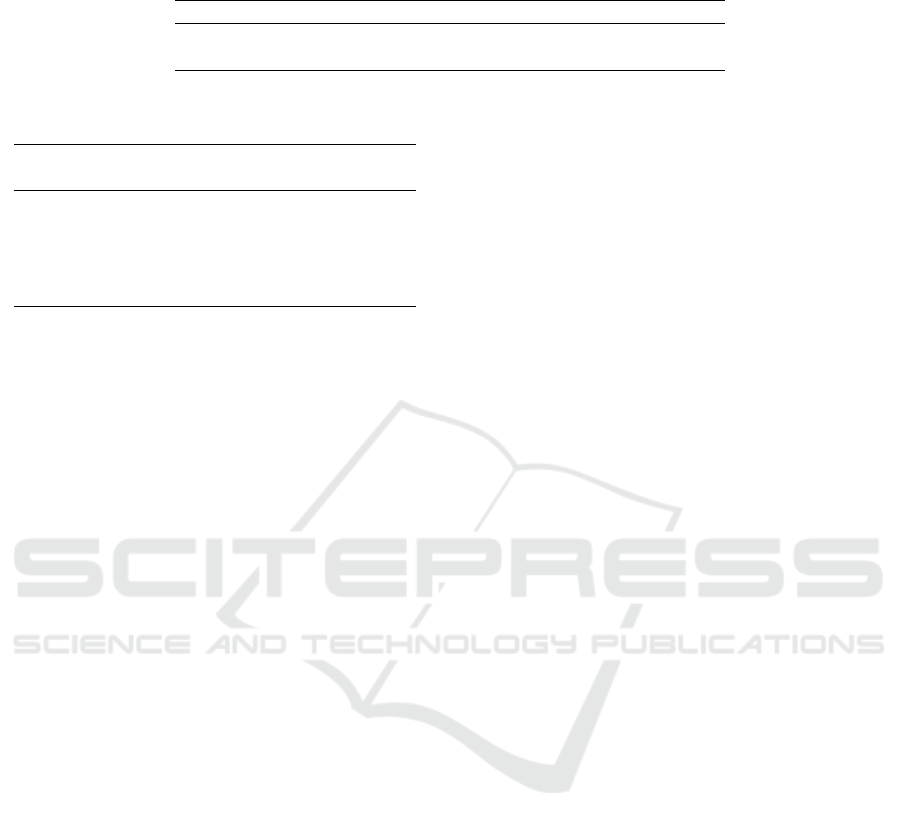
Table 6: Results for the prediction of visible terms. All values are averages with their standard deviations from the extraction
for 329 captions (317 for keywords) using 5-fold cross validation.
Method AUC Prec Rec # of pred.
Vis. terms 0.84 ± 0.17 0.37 ± 0.28 0.80 ± 0.32 7.4 ± 8.6
Key terms 0.80 ± 0.24 0.44 ± 0.32 0.64 ± 0.38 4.4 ± 3.2
Table 7: Coefficients for features in the logistic regression
models.
Vis. Term Keyword
Feature Coefficient Coefficient
idf 0.00 ± 0.00 0.05 ± 0.01
caption similarity 3.75 ± 0.17 4.49 ± 0.18
concreteness 8.63 ± 0.18 0.47 ± 0.07
pattern 3.03 ± 0.67 1.57 ± 0.57
position 0.39 ± 0.03 1.03 ± 0.31
For future work we plan to use contextualised word
embeddings as features for the term classification. We
expect e.g. that the term Fish bone will have different
embeddings in the context Picture of a fish bone than
in the context . . . extracted from fish bone. We expect
that a classifier can use these differences to distinguish
depicted from other concepts.
REFERENCES
Algarabel, S., Ruiz, J. C., and Sanmartin, J. (1988). The
University of Valencia’s computerized word pool. Be-
havior Research Methods, Instruments, & Computers,
20(4):398–403.
Brysbaert, M., Warriner, A. B., and Kuperman, V. (2014).
Concreteness ratings for 40 thousand generally known
English word lemmas. Behavior Research Methods,
46(3):904–911.
Charbonnier, J. and Wartena, C. (2019). Predicting word
concreteness and imagery. In Proceedings of the 13th
International Conference on Computational Semantics
- Long Papers, pages 176–187, Gothenburg, Sweden.
Association for Computational Linguistics.
Clark, J. M. and Paivio, A. (2004). Extensions of the Paivio,
Yuille, and Madigan (1968) norms. Behavior Research
Methods, Instruments, & Computers, 36(3):371–383.
Frank, E., Paynter, G. W., Witten, I. H., Gutwin, C.,
and Nevill-Manning, C. G. (1999). Domain-Specific
Keyphrase Extraction. In Proceedings of the Sixteenth
International Joint Conference on Artificial Intelli-
gence, IJCAI ’99, pages 668–673, San Francisco, CA,
USA. Morgan Kaufmann Publishers Inc.
Friendly, M., Franklin, P. E., Hoffman, D., and Rubin, D. C.
(1982). The Toronto Word Pool: Norms for imagery,
concreteness, orthographic variables, and grammatical
usage for 1,080 words. Behavior Research Methods &
Instrumentation, 14(4):375–399.
Gong, Z., Cheang, C. W., et al. (2006). Web image indexing
by using associated texts. Knowledge and information
systems, 10(2):243–264.
Gong, Z. and Liu, Q. (2009). Improving keyword based
web image search with visual feature distribution and
term expansion. Knowledge and Information Systems,
21(1):113–132.
Hessel, J., Mimno, D., and Lee, L. (2018). Quantifying the
visual concreteness of words and topics in multimodal
datasets. In Proceedings of the 2018 Conference of
the North American Chapter of the Association for
Computational Linguistics: Human Language Tech-
nologies, Volume 1 (Long Papers), pages 2194–2205,
New Orleans, Louisiana. Association for Computa-
tional Linguistics.
Jeong, J.-W., Wang, X.-J., and Lee, D.-H. (2012). Towards
measuring the visualness of a concept. In Proceedings
of the 21st ACM international conference on Informa-
tion and knowledge management, pages 2415–2418.
ACM.
Kehat, G. and Pustejovsky, J. (2017). Integrating vision
and language datasets to measure word concreteness.
In Proceedings of the Eighth International Joint Con-
ference on Natural Language Processing (Volume 2:
Short Papers), pages 103–108, Taipei, Taiwan. Asian
Federation of Natural Language Processing.
Krippendorff, K. (1970). Estimating the reliability, system-
atic error and random error of interval data. Educa-
tional and Psychological Measurement, 30(1):61–70.
Leong, C. W. and Mihalcea, R. (2009). Explorations in
automatic image annotation using textual features. In
Proceedings of the Third Linguistic Annotation Work-
shop (LAW III), pages 56–59.
Leong, C. W., Mihalcea, R., and Hassan, S. (2010). Text
mining for automatic image tagging. In Coling 2010:
Posters, pages 647–655.
Lin, T.-Y., Maire, M., Belongie, S., Hays, J., Perona, P.,
Ramanan, D., Dollár, P., and Zitnick, C. L. (2014).
Microsoft coco: Common objects in context. In Euro-
pean conference on computer vision, pages 740–755.
Springer.
Martinec, R. and Salway, A. (2005). A system for image–text
relations in new (and old) media. Visual communica-
tion, 4(3):337–371.
Mikolov, T., Sutskever, I., Chen, K., Corrado, G., and Dean,
J. (2013). Distributed representations of words and
phrases and their compositionality. In Proceedings of
the 26th International Conference on Neural Informa-
tion Processing Systems - Volume 2, NIPS’13, pages
3111–3119, USA. Curran Associates Inc.
Otto, C., Springstein, M., Anand, A., and Ewerth, R. (2019).
Understanding, categorizing and predicting seman-
tic image-text relations. In Proceedings of the 2019
on International Conference on Multimedia Retrieval,
ICMR ’19, pages 168–176, New York, NY, USA.
ACM.
KDIR 2022 - 14th International Conference on Knowledge Discovery and Information Retrieval
168