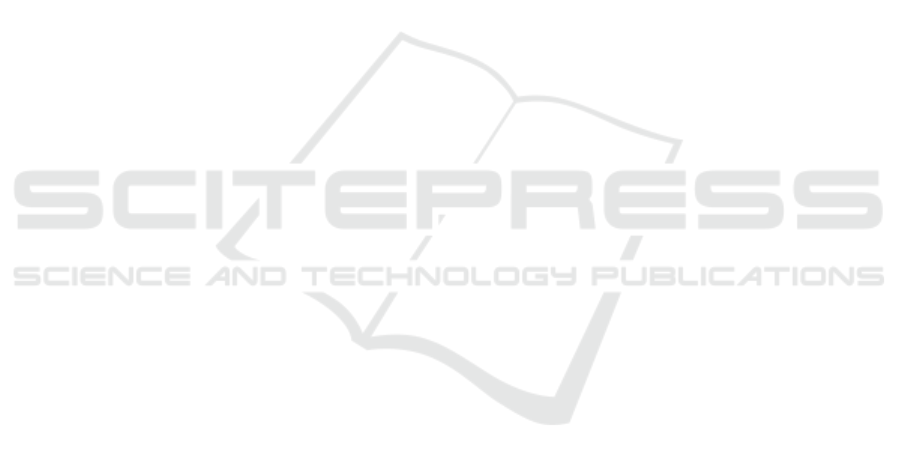
REFERENCES
Aghabozorgi, S. R., Shirkhorshidi, A. S., and Teh, Y. W.
(2015). Time-series clustering - A decade review. Inf.
Syst., 53:16–38.
Barredo Arrieta, A., Diaz-Rodriguez, N., Del Ser, J., Ben-
netot, A., Tabik, S., Barbado, A., Garcia, S., Gil-
Lopez, S., Molina, D., Benjamins, R., Chatila, R., and
Herrera, F. (2020). Explainable artificial intelligence
(XAI): Concepts, taxonomies, opportunities and chal-
lenges toward responsible AI. Inf. Fusion, 58:82–115.
Bennetot, A., Donadello, I., Qadi, A. E., Dragoni, M.,
Frossard, T., Wagner, B., Saranti, A., Tulli, S.,
Trocan, M., Chatila, R., Holzinger, A., d’Avila
Garcez, A., and D
´
ıaz Rodr
´
ıguez, N. (2021). A
Practical Tutorial on Explainable AI Techniques.
https://arxiv.org/abs/2111.14260.
Braud, A., Ganc¸arski, P., Grac, C., Herrmann, A., Le Ber,
F., and Vernier, H. (2021). Classification de s
´
eries
temporelles h
´
et
´
erog
`
enes pour le suivi de l’
´
etat des
cours d’eau. volume E-37 of RNTI, pages 71–82.
Carlos-Sandberg, L. and Clack, C. D. (2021). Incorpora-
tion of causality structures to complex network analy-
sis of time-varying behaviour of multivariate time se-
ries. Scientific Reports, 11(1):18880.
Chraibi Kaadoud, I., Fahed, L., and Lenca, P. (2021). Ex-
plainable AI: a narrative review at the crossroad of
knowledge discovery, knowledge representation and
representation learning. In MRC@IJCAI, vol 2995 of
CEUR Workshop Proceedings, pages 28–40.
Chraibi Kaadoud, I., Rougier, N. P., and Alexandre, F.
(2022). Knowledge extraction from the learning of
sequences in a long short term memory (LSTM) ar-
chitecture. Knowl. Based Syst., 235:107657.
Cortez, P. and Embrechts, M. J. (2013). Using sensitiv-
ity analysis and visualization techniques to open black
box data mining models. Inf. Sci., 225:1–17.
Desmarais, M. C. and Lemieux, F. (2013). Clustering and
visualizing study state sequences. In EDM, pages
224–227.
Ghassempour, S., Girosi, F., and Maeder, A. (2014). Clus-
tering multivariate time series using hidden markov
models. Int. J. of environmental research and public
health, 11(3):2741–2763.
Giorgino, T. (2009). Computing and visualizing dynamic
time warping alignments in R: the dtw package. J.
Stat. Softw., 31:1–24.
Guespin-Michel, J. (2016). La science des syst
`
emes com-
plexes. https://urlz.fr/hIxs. Visited: 2022-5-4.
Guidotti, R., Monreale, A., Ruggieri, S., Turini, F., Gian-
notti, F., and Pedreschi, D. (2019). A survey of meth-
ods for explaining black box models. ACM Comput.
Surv., 51(5):93:1–93:42.
Gunning, D. (2019). DARPA’s explainable artificial intelli-
gence (XAI) program. In Int. Conf. on Intelligent User
Interfaces. ACM.
Harel, D. (1987). Statecharts: A visual formalism for com-
plex systems. Sci. Comput. Program, 8(3):231–274.
Hassanibesheli, F., Boers, N., and Kurths, J. (2020). Recon-
structing complex system dynamics from time series:
a method comparison. New J. Phys, 22(7):073053.
Higgs, B. and Abbas, M. (2014). Segmentation and cluster-
ing of car-following behavior: Recognition of driving
patterns. IEEE Trans. Intell. Transp. Syst., 16(1):81–
90.
Le Saux, E., Lenca, P., and Picouet, P. (2002). Dynamic
adaptation of rules bases under cognitive constraints.
Eur. J. Oper. Res, 136(2):299–309.
Lenca, P. (2002). Human centered processes. Eur. J. Oper.
Res, 136(2):231–232.
Moshkovitz, M., Dasgupta, S., Rashtchian, C., and Frost,
N. (2020). Explainable k-means and k-medians clus-
tering. In ICML, pages 7055–7065.
Omlin, C. W. and Giles, C. L. (1996). Extraction of rules
from discrete-time recurrent neural networks. Neural
Networks, 9(1):41–52.
Pham, V., Nguyen, N., Li, J., Hass, J., Chen, Y., and Dang,
T. (2019). MTSAD: Multivariate time series abnor-
mality detection and visualization. In IEEE Big Data,
pages 3267–3276.
Rousseeuw, P. J. (1987). Silhouettes: a graphical aid to
the interpretation and validation of cluster analysis. J.
Computational Applied Mathematics, 20:53–65.
Shortliffe, E. H. (1974). A rule-based computer program
for advising physicians regarding antimicrobial ther-
apy selection. In ACM Annual Conference, page 739.
Soto, M. G., Henzinger, T. A., and Schilling, C. (2021).
Synthesis of hybrid automata with affine dynamics
from time-series data. In HSCC, pages 1–11.
Theissler, A. (2013). Detecting anomalies in multivari-
ate time series from automotive systems. PhD thesis,
Brunel Univ. School of Engineering and Design.
van Fraassen, B. (1988). The pragmatic theory of explana-
tion. Theories of Explanation, 8:136–155.
Weitz, K., Schiller, D., Schlagowski, R., Huber, T., and
Andr
´
e, E. (2021). “let me explain!”: exploring the
potential of virtual agents in explainable ai interaction
design. J. Multimodal User Interfaces, 15(2):87–98.
Weld, D. S. and Bansal, G. (2019). The challenge of crafting
intelligible intelligence. Commun. ACM, 62(6):70–79.
Yang, F., Huang, Z., Scholtz, J., and Arendt, D. L. (2020).
How do visual explanations foster end users’ appro-
priate trust in machine learning? In In. Conf. on Intel-
ligent User Interfaces, pages 189–201. ACM.
Yang, K. and Shahabi, C. (2004). A PCA-based similarity
measure for multivariate time series. In Int. workshop
on Multimedia databases, pages 65–74.
Zeng, Z., Goodman, R. M., and Smyth, P. (1993). Learn-
ing finite-state machines with self-clustering recurrent
networks. Neural Comput., 5(6):976–990.
Zhang, Y., Lin, Q., Wang, J., and Verwer, S. (2017). Car-
following behavior model learning using timed au-
tomata. IFAC-PapersOnLine, 50(1):2353–2358.
Automata-based Explainable Representation for a Complex System of Multivariate Times Series
179