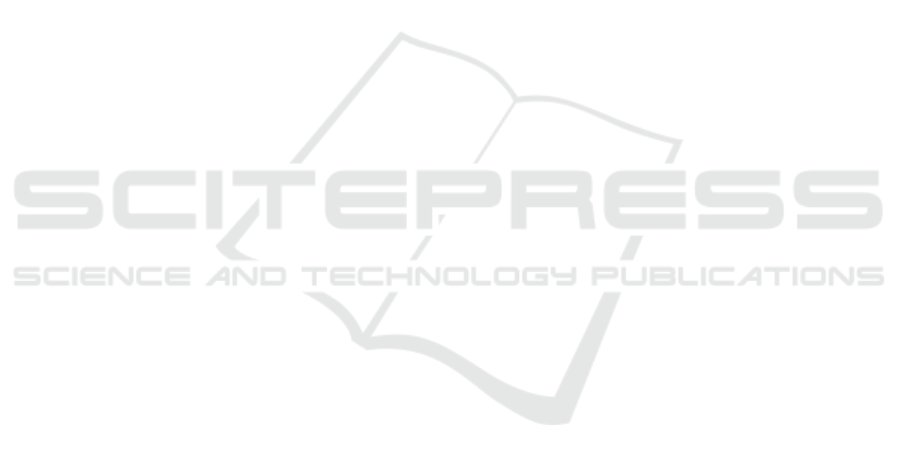
ACKNOWLEDGEMENTS
This work was supported by the Brazilian Na-
tional Council for Scientific and Technological De-
velopment (CNPq) under grants 304456/2020-9,
04274/2019-4 and 302467/2019-0, by the Ripple Im-
pact Fund, a donor advised fund of the Silicon Valley
Community Foundation, grant 2018-196450(5855) as
part of the University Blockchain Research Initiative,
UBRI, and by the Research Foundation of the State of
S
˜
ao Paulo (FAPESP), grant 2020/09838-0.
REFERENCES
Atsalakis, G. S., Atsalaki, I. G., Pasiouras, F., and Zopouni-
dis, C. (2019). Bitcoin price forecasting with neuro-
fuzzy techniques. European Journal of Operational
Research, 276(2):770–780.
Balcilar, M., Bouri, E., Gupta, R., and Roubaud, D. (2017).
Can volume predict bitcoin returns and volatility?
a quantiles-based approach. Economic Modelling,
62:74–81.
Box, G., Jenkins, G., Reinsel, G., and Jung, G. (2016). Time
Series Analysis, Forecasting and Control. John Wiley,
Hoboken.
Burns, K. and Moosa, I. (2015). Enhancing the forecasting
power of exchange rate models by introducing nonlin-
earity: Does it work? Economic Modelling, 50:27–39.
Chowdhury, R., Rahman, M. A., Rahman, M. S., and
Mahdy, M. (2020). An approach to predict and fore-
cast the price of constituents and index of cryptocur-
rency using machine learning. Physica A: Statistical
Mechanics and its Applications, 551:124569.
Fung, K., Jeong, J., and Pereira, J. (2021). More to cryp-
tos than bitcoin: A garch modelling of heterogeneous
cryptocurrencies. Finance Research Letters, page
102544.
Garcia, C., Esmin, A., Leite, D., and
ˇ
Skrjanc, I. (2019).
Evolvable fuzzy systems from data streams with miss-
ing values: With application to temporal pattern
recognition and cryptocurrency prediction. Pattern
Recognition Letters, 128:278–282.
Haykin, S. (2009). Neural Networks and Learning Ma-
chines. Pearson Education, Upper Saddle River.
Hitam, N. A., Ismail, A. R., and Saeed, F. (2019). An opti-
mized support vector machine (svm) based on particle
swarm optimization (pso) for cryptocurrency forecast-
ing. Procedia Computer Science, 163:427–433. 16th
Learning and Technology Conference 2019Artificial
Intelligence and Machine Learning: Embedding the
Intelligence.
Hyndman, R., Koehler, A., Ord, J., and Snyder, R. (2008).
Forecasting with exponential smoothing: the state
space approach. Springer-Verlag.
Lahmiri, S. and Bekiros, S. (2019). Cryptocurrency fore-
casting with deep learning chaotic neural networks.
Chaos, Solitons & Fractals, 118:35–40.
Leite, D., Gomide, F., and Yager, R. (2022). Data driven
fuzzy modeling using level sets. In 2022 IEEE World
Congress on Computational Intelligence, pages 1–10.
Maciel, L., Ballini, R., and Gomide, F. (2022). Data driven
level set method in fuzzy modeling and forecasting. In
2022 Annual Conference of the North America Fuzzy
Information Processing Society, pages 1–10.
Meese, R. and Rogoff, K. (1983). Empirical exchange rate
models of the seventies: Do they fit out of sample?
Journal of International Economics, 14(1–2):3–24.
Moosa, I. and Burns, K. (2014). The unbeatable random
walk in exchange rate forecasting: Reality or myth?
Journal of Macroeconomics, 40:69–81.
Mukhopadhyay, U., Skjellum, A., Hambolu, O., Oakley, J.,
Yu, L., and Brooks, R. (2016). A brief survey of cryp-
tocurrency systems. In 14th IEEE Annual Conference
on Privacy, Security and Trust (PST), pages 745–752.
Nakamoto, S. (2008). Bitcoin: A peer-to-peer electronic
cash system. Decentralized Business Review, 21260.
Parfenov, D. (2022). Efficiency linkages between cryp-
tocurrencies, equities and commodities at different
time frames. Procedia Computer Science, 199:182–
189.
Philippas, D., Rjiba, H., Guesmi, K., and Goutte, S. (2019).
Media attention and bitcoin prices. Finance Research
Letters, 30:37–43.
Selmi, R., Mensi, W., Hammoudeh, S., and Bouoiyour, J.
(2018). Is bitcoin a hedge, a safe haven or a diversi-
fier for oil price movements? a comparison with gold.
Energy Economics, 74:787–801.
Serre, D. (2010). Matrices: Theory and Applications.
Springer, New York.
Sun, X., Liu, M., and Sima, Z. (2020). A novel cryptocur-
rency price trend forecasting model based on light-
gbm. Finance Research Letters, 32:101084.
Tandon, C., Revankar, S., Palivela, H., and Parihar, S. S.
(2021). How can we predict the impact of the
social media messages on the value of cryptocur-
rency? insights from big data analytics. International
Journal of Information Management Data Insights,
1(2):100035.
Yager, R. (1991). An alternative procedure for the cal-
culation of fuzzy logic controller values. Journal of
the Japanese Society for Fuzzy Theory and Systems,
4:736–746.
Zhang, Z., Dai, H.-N., Zhou, J., Mondal, S. K., Garc
´
ıa,
M. M., and Wang, H. (2021). Forecasting cryptocur-
rency price using convolutional neural networks with
weighted and attentive memory channels. Expert Sys-
tems with Applications, 183:115378.
FCTA 2022 - 14th International Conference on Fuzzy Computation Theory and Applications
198