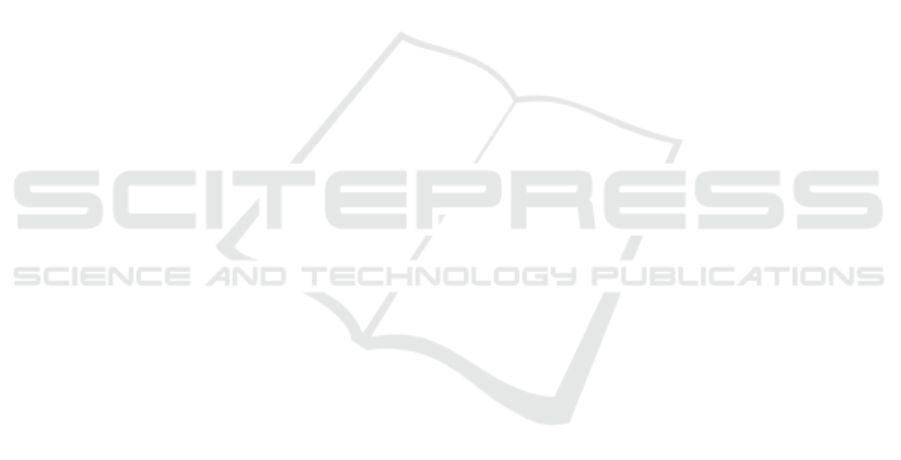
Chung, D., Choi, J., Jang, J.-H., Kim, T. Y., Byun, J., Park,
H., Lim, H.-S., Park, R. W., and Yoon, D. (2018).
Construction of an electrocardiogram database includ-
ing 12 lead waveforms. Healthcare informatics re-
search, 24(3):242–246.
Dau, H. A. and Keogh, E. (2017). Matrix profile V: A
generic technique to incorporate domain knowledge
into motif discovery. In Proceedings of the 23rd
ACM SIGKDD International Conference on Knowl-
edge Discovery and Data Mining, pages 125–134.
Deng, H., Guo, P., Zheng, M., Huang, J., Xue, Y., Zhan,
X., Wang, F., Liu, Y., Fang, X., Liao, H., et al.
(2018). Epidemiological characteristics of atrial fibril-
lation in southern china: results from the guangzhou
heart study. Scientific reports, 8(1):1–10.
Ebrahimi, Z., Loni, M., Daneshtalab, M., and Gharehbaghi,
A. (2020). A review on deep learning methods for ecg
arrhythmia classification. Expert Systems with Appli-
cations: X, 7:100033.
Fortune, J., Coppa, N., Haq, K. T., Patel, H., and
Tereshchenko, L. G. (2021). Digitizing ecg image:
new fully automated method and open-source soft-
ware code. medRxiv.
Furfaro, A., Groccia, M. C., and Rombo, S. E. (2017). 2d
motif basis applied to the classification of digital im-
ages. The Computer Journal, 60(7):1096–1109.
Gupta, V., Mittal, M., Mittal, V., and Saxena, N. K. (2021).
A critical review of feature extraction techniques for
ecg signal analysis. Journal of The Institution of En-
gineers (India): Series B, pages 1–12.
Houssein, E. H., Kilany, M., and Hassanien, A. E.
(2017). Ecg signals classification: a review. Interna-
tional Journal of Intelligent Engineering Informatics,
5(4):376–396.
Jayaraman, S., Swamy, P., Damodaran, V., and Venkatesh,
N. (2012). A novel technique for ecg morphology in-
terpretation and arrhythmia detection based on time
series signal extracted from scanned ecg record. Ad-
vances in Electrocardiograms-Methods and Analysis,
pages 127–140.
Kar, A. and Das, L. (2011). A technical review on statisti-
cal feature extraction of ecg signal. In IJCA Special
Issue on 2nd National Conference-Computing, Com-
munication and Sensor Network, CCSN, pages 35–40.
Khleaf, H. K., Ghazali, K. H., and Abdalla, A. N. (2013).
Features extraction technique for ecg recording paper.
In Proceeding of the International Conference on Ar-
tificial Intelligence in Computer Science and ICT.
Liu, X., Wang, H., Li, Z., and Qin, L. (2021). Deep learning
in ecg diagnosis: A review. Knowledge-Based Sys-
tems, 227:107187.
Loresco, P. J. M. and Africa, A. D. (2018). Ecg print-out
features extraction using spatial-oriented image pro-
cessing techniques. Journal of Telecommunication,
Electronic and Computer Engineering (JTEC), 10(1-
5):15–20.
Mir, H. Y. and Singh, O. (2021). Ecg denoising and feature
extraction techniques–a review. Journal of medical
engineering & technology, 45(8):672–684.
Mishra, S., Khatwani, G., Patil, R., Sapariya, D., Shah, V.,
Parmar, D., Dinesh, S., Daphal, P., and Mehendale, N.
(2021). Ecg paper record digitization and diagnosis
using deep learning. Journal of Medical and Biologi-
cal Engineering, pages 1–11.
Ravichandran, L., Harless, C., Shah, A. J., Wick, C. A.,
Mcclellan, J. H., and Tridandapani, S. (2013). Novel
tool for complete digitization of paper electrocardiog-
raphy data. IEEE journal of translational engineering
in health and medicine, 1:1800107–1800107.
Roth, G. A., Mensah, G. A., and et al., C. O. J. (2020).
Global burden of cardiovascular diseases and risk
factors, 1990–2019: Update from the gbd 2019
study. Journal of The American College of Cariology,
(25):2982–3201.
Seena, V. and Yomas, J. (2014). A review on feature extrac-
tion and denoising of ecg signal using wavelet trans-
form. In 2014 2nd international conference on de-
vices, circuits and systems (ICDCS), pages 1–6. IEEE.
Sm
´
ı
ˇ
sek, R. (2016). Ecg signal classification based on svm.
Biomedical Engineering, (1):365–369.
Thanapatay, D., Suwansaroj, C., and Thanawattano, C.
(2010). Ecg beat classification method for ecg printout
with principle components analysis and support vector
machines. In 2010 International Conference on Elec-
tronics and Information Engineering, volume 1, pages
V1–72. IEEE.
Torkamani, S. and Lohweg, V. (2017). Survey on time se-
ries motif discovery. Wiley Interdisciplinary Reviews:
Data Mining and Knowledge Discovery, 7(2):e1199.
Truong, C. D. and Anh, D. T. (2019). A survey on time
series motif discovery. International Journal of Busi-
ness Intelligence and Data Mining, 15(2):204–227.
Waits, G. S. and Soliman, E. Z. (2017). Digitizing paper
electrocardiograms: Status and challenges. Journal of
electrocardiology, 50(1):123–130.
Wankhedkar, R. and Jain, S. K. (2019). A brief survey on
techniques used in discovering time series motifs. In
Proceedings of the International Conference on Ad-
vances in Electronics, Electrical & Computational In-
telligence (ICAEEC).
KDIR 2022 - 14th International Conference on Knowledge Discovery and Information Retrieval
28