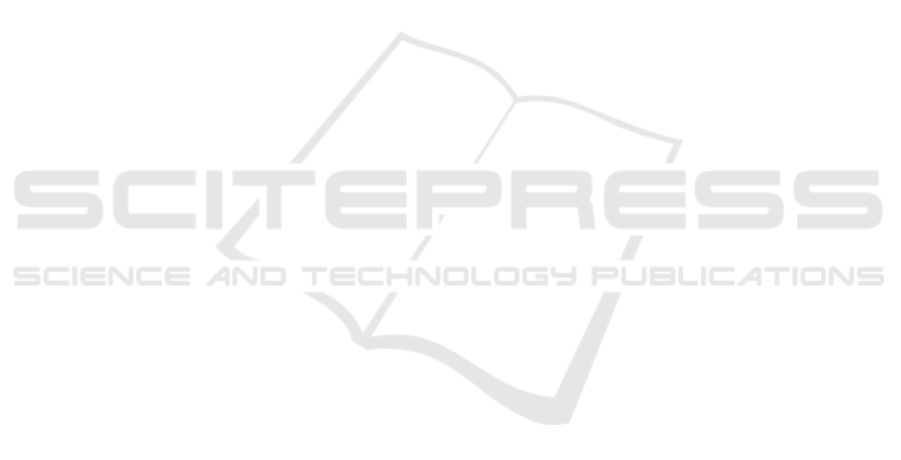
6 CONCLUSION
Having alternatives regarding products in e-
commerce plays a key role for the purchase.
However, finding and filtering adequate and compat-
ible products based on customer’s natural language
questions remains a very difficult task. In this article,
we proposed a novel methodology to recommend
e-commerce products based on RDF KGs. The rec-
ommendation uses questions asked by e-commerce
customers interested if their consumer item is com-
patible with the sales product. Our recommendation
technique queries a KG filled with compatibility
between consumer items and products. Our proposal
was implemented and deployed in a real-world e-
commerce platform in Latin America. We found that
our solution is suitable for recommending relevant
products in the e-commerce platform. Future work
involves the study of novel methods to compare
available products on e-commerce platforms. This
work used textual properties, such as categories,
store, and domain. The recommendation algorithm
could further benefit from comparing NL texts and
images, for instance. In addition, based on the
evaluation results, we plan to further assess the use
of products that are universally compatible within the
recommendation feature.
ACKNOWLEDGEMENTS
This study was financed by the National Council for
Scientific and Technological Development - Brazil
(CNPq) process number 140213/2021-0.
REFERENCES
Covington, P., Adams, J., and Sargin, E. (2016). Deep
neural networks for youtube recommendations. In
Proceedings of the 10th ACM Conference on Rec-
ommender Systems, RecSys ’16, page 191–198, New
York, NY, USA. Association for Computing Machin-
ery.
Dwivedi, R., Anand, A., Johri, P., Banerji, A., and Gaur,
N. (2020). Product based recommendation system on
amazon data. International Journal of Creative Re-
search Thoughts - IJCRT.
Guo, Q., Zhuang, F., Qin, C., Zhu, H., Xie, X., Xiong, H.,
and He, Q. (2020). A survey on knowledge graph-
based recommender systems. IEEE Transactions on
Knowledge & Data Engineering, (01):1–1.
Isinkaye, F. O., Folajimi, Y., and Ojokoh, B. A. (2015). Rec-
ommendation systems: Principles, methods and eval-
uation. Egyptian informatics journal, 16(3):261–273.
Lee, D. and Hosanagar, K. (2014). Impact of recommender
systems on sales volume and diversity. In Myers,
M. D. and Straub, D. W., editors, Proceedings of the
International Conference on Information Systems -
Building a Better World through Information Systems,
ICIS 2014, Auckland, New Zealand, December 14-17,
2014. Association for Information Systems.
Linden, G., Smith, B., and York, J. (2003). Amazon. com
recommendations: Item-to-item collaborative filter-
ing. IEEE Internet computing, 7(1):76–80.
Luo, X., Liu, L., Yang, Y., Bo, L., Cao, Y., Wu, J., Li, Q.,
Yang, K., and Zhu, K. Q. (2020). Alicoco: Alibaba
e-commerce cognitive concept net. In Proceedings of
the 2020 ACM SIGMOD International Conference on
Management of Data, pages 313–327.
Sant’Anna, D. T., Caus, R. O., dos Santos Ramos, L.,
Hochgreb, V., and dos Reis, J. C. (2020). Generat-
ing knowledge graphs from unstructured texts: Expe-
riences in the e-commerce field for question answer-
ing. In Advances in Semantics and Linked Data: Joint
Workshop Proceedings from ISWC 2020, pages 56–
71.
Shao, B., Li, X., and Bian, G. (2021). A survey of research
hotspots and frontier trends of recommendation sys-
tems from the perspective of knowledge graph. Expert
Systems with Applications, 165:113764.
Wu, P., Zhang, X., and Feng, Z. (2019). A survey of ques-
tion answering over knowledge base. In Zhu, X., Qin,
B., Zhu, X., Liu, M., and Qian, L., editors, Knowledge
Graph and Semantic Computing: Knowledge Com-
puting and Language Understanding, pages 86–97,
Singapore. Springer Singapore.
KEOD 2022 - 14th International Conference on Knowledge Engineering and Ontology Development
42