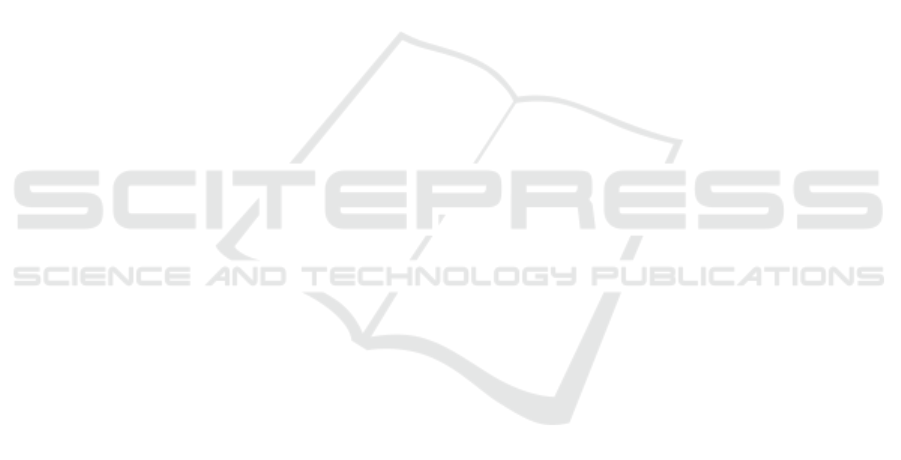
Ib
´
a
˜
nez, M., Malan, K. M., Moore, J. H., Naujoks, B.,
Orzechowski, P., Volz, V., Wagner, M., and Weise, T.
(2020). Benchmarking in Optimization: Best Practice
and Open Issues. arXiv, pages 1–50.
Braam, F. and van den Berg, D. (2022). Which rectangle
sets have perfect packings? Operations Research Per-
spectives, page 100211.
Brouwer, N., Dijkzeul, D., Koppenhol, L., Pijning, I., and
Van den Berg, D. (2022). Survivor selection in a
crossoverless evolutionary algorithm. In Proceedings
of the 2022 Genetic and Evolutionary Computation
Conference Companion. (in press).
Brownlee, J. et al. (2007). A note on research methodol-
ogy and benchmarking optimization algorithms. Com-
plex Intelligent Systems Laboratory (CIS), Centre for
Information Technology Research (CITR), Faculty of
Information and Communication Technologies (ICT),
Swinburne University of Technology, Victoria, Aus-
tralia, Technical Report ID, 70125.
Dahmani, R., Boogmans, S., Meijs, A., and Van den Berg,
D. (2020). Paintings-from-polygons: Simulated an-
nealing. In ICCC.
De Jonge, M. and van den Berg, D. (2020). Parameter sen-
sitivity patterns in the plant propagation algorithm. In
IJCCI, page 92–99.
de Jonge, M. and van den Berg, D. (2020). Plant propa-
gation parameterization: Offspring & population size.
Evo* 2020, page 19.
Dijkzeul, D., Brouwer, N., Pijning, I., Koppenhol, L., and
Van den Berg, D. (2022). Painting with evolutionary
algorithms. In International Conference on Compu-
tational Intelligence in Music, Sound, Art and Design
(Part of EvoStar), pages 52–67. Springer.
Doerr, C., Ye, F., Horesh, N., Wang, H., Shir, O. M., and
B
¨
ack, T. (2020). Benchmarking discrete optimization
heuristics with iohprofiler. Applied Soft Computing,
88:106027.
Fraga, E. S. (2019). An example of multi-objective opti-
mization for dynamic processes. Chemical Engineer-
ing Transactions, 74:601–606.
Gallagher, M. and Yuan, B. (2006). A general-purpose tun-
able landscape generator. IEEE transactions on evo-
lutionary computation, 10(5):590–603.
Geleijn, R., van der Meer, M., van der Post, Q., and van den
Berg, D. (2019). The plant propagation algorithm on
timetables: First results. EVO* 2019, page 2.
Haddadi, S. (2020). Plant propagation algorithm for nurse
rostering. International Journal of Innovative Com-
puting and Applications, 11(4):204–215.
Iori, M., De Lima, V., Martello, S., and M., M. (2021a).
2dpacklib: a two-dimensional cutting and packing li-
brary (in press). Optimization Letters.
Iori, M., de Lima, V. L., Martello, S., Miyazawa, F. K., and
Monaci, M. (2021b). Exact solution techniques for
two-dimensional cutting and packing. European Jour-
nal of Operational Research, 289(2):399–415.
Iori, M., de Lima, V. L., Martello, S., and Monaci, M.
(2021c). 2dpacklib.
Kerschke, P. and Trautmann, H. (2019). Comprehen-
sive feature-based landscape analysis of continuous
and constrained optimization problems using the r-
package flacco. In Applications in Statistical Com-
puting, pages 93–123. Springer.
Koppenhol, L., Brouwer, N., Dijkzeul, D., Pijning, I.,
Sleegers, J., and Van den Berg, D. (2022). Survivor
selection in a crossoverless evolutionary algorithm.
In Proceedings of the 2022 Genetic and Evolutionary
Computation Conference Companion. (in press).
Laguna, M. and Marti, R. (2005). Experimental testing of
advanced scatter search designs for global optimiza-
tion of multimodal functions. Journal of Global Opti-
mization, 33(2):235–255.
Lou, Y., Yuen, S. Y., and Chen, G. (2018). Evolving bench-
mark functions using kruskal-wallis test. In Proceed-
ings of the Genetic and Evolutionary Computation
Conference Companion, pages 1337–1341.
Malan, K. M. and Engelbrecht, A. P. (2013). A survey of
techniques for characterising fitness landscapes and
some possible ways forward. Information Sciences,
241:148–163.
Paauw, M. and Van den Berg, D. (2019). Paintings,
polygons and plant propagation. In International
Conference on Computational Intelligence in Music,
Sound, Art and Design (Part of EvoStar), pages 84–
97. Springer.
Pohlheim, H. (2007). Examples of objective functions. Re-
trieved, 4(10):2012.
Reinelt, G. (1991). Tsplib—a traveling salesman problem
library. ORSA journal on computing, 3(4):376–384.
Rice, J. R. (1976). The algorithm selection problem. In Ad-
vances in computers, volume 15, pages 65–118. Else-
vier.
Rodman, A. D., Fraga, E. S., and Gerogiorgis, D. (2018).
On the application of a nature-inspired stochastic
evolutionary algorithm to constrained multi-objective
beer fermentation optimisation. Computers & Chemi-
cal Engineering, 108:448–459.
Salhi, A. and Fraga, E. S. (2011). Nature-inspired optimi-
sation approaches and the new plant propagation algo-
rithm.
Selamo
˘
glu, B.
˙
I. and Salhi, A. (2016). The plant propaga-
tion algorithm for discrete optimisation: The case of
the travelling salesman problem. In Nature-inspired
computation in engineering, pages 43–61. Springer.
Sleegers, J. and Berg, D. v. d. (2021). Backtracking (the)
algorithms on the hamiltonian cycle problem. arXiv
preprint arXiv:2107.00314.
Sleegers, J. and van den Berg, D. (2020a). Looking for
the hardest hamiltonian cycle problem instances. In
IJCCI, pages 40–48.
Sleegers, J. and van den Berg, D. (2020b). Plant propaga-
tion & hard hamiltonian graphs. Evo* 2020, page 10.
Smith-Miles, K., Hemert, J. v., and Lim, X. Y. (2010). Un-
derstanding tsp difficulty by learning from evolved in-
stances. In International conference on learning and
intelligent optimization, pages 266–280. Springer.
Socha, K. and Dorigo, M. (2008). Ant colony optimization
for continuous domains. European journal of opera-
tional research, 185(3):1155–1173.
Making Hard(Er) Bechmark Test Functions
37