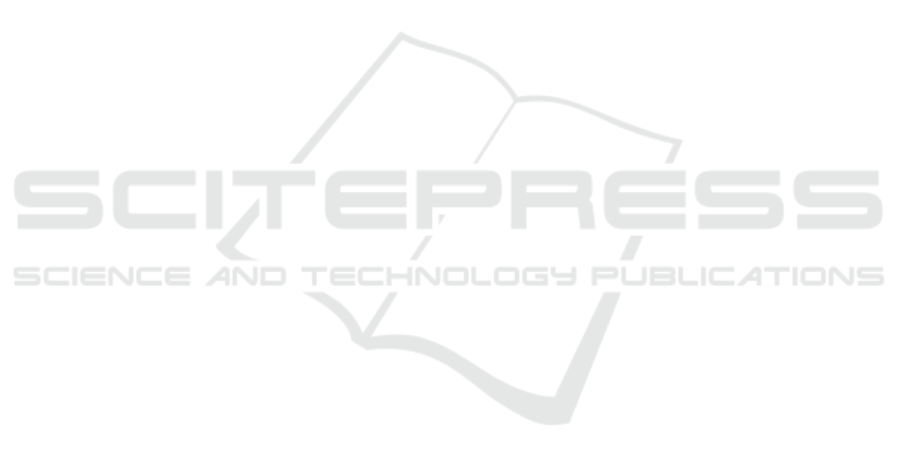
framework for handwritten character recognition. In
2015 3rd IAPR Asian Conference on Pattern Recogni-
tion (ACPR), pages 695–699. IEEE.
Chu, W. and Cai, D. (2018). Deep feature based con-
textual model for object detection. Neurocomputing,
275:1035–1042.
Engstrom, L., Tran, B., Tsipras, D., Schmidt, L., and
Madry, A. (2019). Exploring the landscape of spatial
robustness. In International Conference on Machine
Learning, pages 1802–1811. PMLR.
Eykholt, K., Evtimov, I., Fernandes, E., Li, B., Rahmati, A.,
Xiao, C., Prakash, A., Kohno, T., and Song, D. (2018).
Robust physical-world attacks on deep learning visual
classification. In Proceedings of the IEEE Conference
on Computer Vision and Pattern Recognition (CVPR).
Finlayson, S. G., Bowers, J. D., Ito, J., Zittrain, J. L., Beam,
A. L., and Kohane, I. S. (2019). Adversarial attacks on
medical machine learning. Science, 363(6433):1287–
1289.
He, K., Zhang, X., Ren, S., and Sun, J. (2016). Deep resid-
ual learning for image recognition. In Proceedings of
the IEEE conference on computer vision and pattern
recognition, pages 770–778.
Heaven, D. (2019). Why deep-learning ais are so easy to
fool.
Hendrycks, D., Zhao, K., Basart, S., Steinhardt, J.,
and Song, D. (2019). Natural adversarial exam-
ples.(2019). arXiv preprint cs.LG/1907.07174.
Komkov, S. and Petiushko, A. (2021). Advhat: Real-world
adversarial attack on arcface face id system. In 2020
25th International Conference on Pattern Recognition
(ICPR), pages 819–826. IEEE.
Maron, R. C., Haggenm
¨
uller, S., von Kalle, C., Utikal,
J. S., Meier, F., Gellrich, F. F., Hauschild, A., French,
L. E., Schlaak, M., Ghoreschi, K., Kutzner, H., Heppt,
M. V., Haferkamp, S., Sondermann, W., Schadendorf,
D., Schilling, B., Hekler, A., Krieghoff-Henning, E.,
Kather, J. N., Fr
¨
ohling, S., Lipka, D. B., and Brinker,
T. J. (2021a). Robustness of convolutional neural net-
works in recognition of pigmented skin lesions. Euro-
pean Journal of Cancer, 145:81–91.
Maron, R. C., Schlager, J. G., Haggenm
¨
uller, S., von Kalle,
C., Utikal, J. S., Meier, F., Gellrich, F. F., Hobels-
berger, S., Hauschild, A., French, L., Heinzerling, L.,
Schlaak, M., Ghoreschi, K., Hilke, F. J., Poch, G.,
Heppt, M. V., Berking, C., Haferkamp, S., Sonder-
mann, W., Schadendorf, D., Schilling, B., Goebeler,
M., Krieghoff-Henning, E., Hekler, A., Fr
¨
ohling, S.,
Lipka, D. B., Kather, J. N., and Brinker, T. J. (2021b).
A benchmark for neural network robustness in skin
cancer classification. European Journal of Cancer,
155:191–199.
Nguyen, A., Yosinski, J., and Clune, J. (2015). Deep neural
networks are easily fooled: High confidence predic-
tions for unrecognizable images. In Proceedings of
the IEEE conference on computer vision and pattern
recognition, pages 427–436.
Papernot, N., McDaniel, P., Goodfellow, I., Jha, S., Celik,
Z. B., and Swami, A. (2017). Practical black-box at-
tacks against machine learning. In Proceedings of the
2017 ACM on Asia conference on computer and com-
munications security, pages 506–519.
Szegedy, C., Vanhoucke, V., Ioffe, S., Shlens, J., and Wo-
jna, Z. (2016). Rethinking the inception architecture
for computer vision. In Proceedings of the IEEE con-
ference on computer vision and pattern recognition,
pages 2818–2826.
Szegedy, C., Zaremba, W., Sutskever, I., Bruna, J., Er-
han, D., Goodfellow, I., and Fergus, R. (2013). In-
triguing properties of neural networks. arXiv preprint
arXiv:1312.6199.
Tan, M. and Le, Q. (2019). Efficientnet: Rethinking model
scaling for convolutional neural networks. In Interna-
tional Conference on Machine Learning, pages 6105–
6114. PMLR.
Thys, S., Ranst, W. V., and Goedem
´
e, T. (2019). Fooling au-
tomated surveillance cameras: Adversarial patches to
attack person detection. 2019 IEEE/CVF Conference
on Computer Vision and Pattern Recognition Work-
shops (CVPRW), pages 49–55.
Uesato, J., O’donoghue, B., Kohli, P., and Oord, A. (2018).
Adversarial risk and the dangers of evaluating against
weak attacks. In International Conference on Machine
Learning, pages 5025–5034. PMLR.
NCTA 2022 - 14th International Conference on Neural Computation Theory and Applications
272