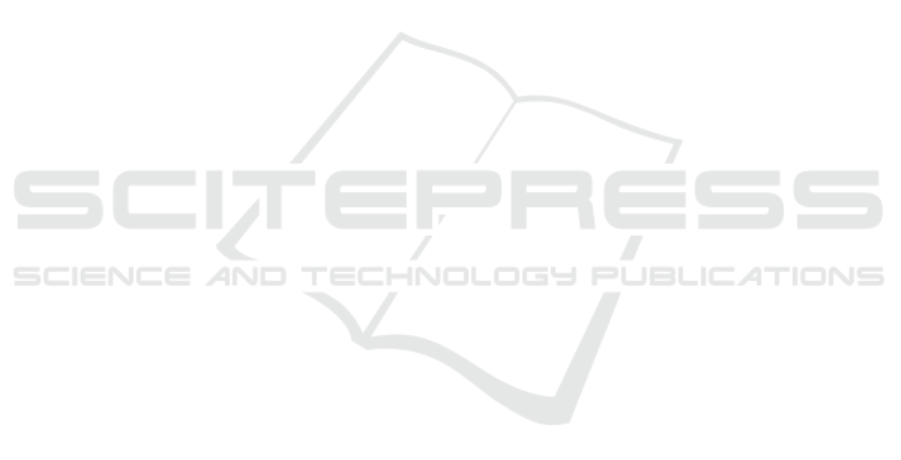
Ceccato, V. and Loukaitou-Sideris, A. (2022). Fear of sex-
ual harassment and its impact on safety perceptions in
transit environments: a global perspective. Violence
against women, 28(1):26–48.
Chu, C., Hsu, A.-L., Chou, K.-H., Bandettini, P., Lin, C.,
Initiative, A. D. N., et al. (2012). Does feature selec-
tion improve classification accuracy? impact of sam-
ple size and feature selection on classification using
anatomical magnetic resonance images. Neuroimage,
60(1):59–70.
Chun, S. A., Avinash Paturu, V., Yuan, S., Pathak, R.,
Atluri, V., and R. Adam, N. (2019). Crime predic-
tion model using deep neural networks. In Proc. of
the 20th Annual International Conf. on Digital Gov-
ernment Research, pages 512–514.
Guyon, I., Weston, J., Barnhill, S., and Vapnik, V. (2002).
Gene selection for cancer classification using support
vector machines. Machine learning, 46(1):389–422.
Hajela, G., Chawla, M., and Rasool, A. (2020). A clus-
tering based hotspot identification approach for crime
prediction. Procedia Computer Science, 167:1462–
1470.
Hilt, D. E. and Seegrist, D. W. (1977). Ridge, a computer
program for calculating ridge regression estimates,
volume 236. Department of Agriculture, Forest Ser-
vice, Northeastern Forest Experiment . . . .
Iqbal, R., Murad, M. A. A., Mustapha, A., Panahy, P. H. S.,
and Khanahmadliravi, N. (2013). An experimental
study of classification algorithms for crime prediction.
Indian J. Science and Technology, 6(3):4219–4225.
Isafiade, O. E. and Bagula, A. B. (2020). Series mining for
public safety advancement in emerging smart cities.
Future Generation Computer Systems, 108:777–802.
Islam, K. and Raza, A. (2020). Forecasting crime using
arima model. arXiv preprint arXiv:2003.08006.
Ivan, N., Ahishakiye, E., Omulo, E. O., and Taremwa, D.
(2017a). Crime prediction using decision tree (j48)
classification algorithm.
Ivan, N., Ahishakiye, E., Omulo, E. O., and Wario, R.
(2017b). A performance analysis of business intel-
ligence techniques on crime prediction.
Kadar, C., Iria, J., and Cvijikj, I. P. (2016). Exploring
foursquare-derived features for crime prediction in
new york city. In The 5th international workshop on
urban computing (UrbComp 2016). ACM.
Kim, S., Joshi, P., Kalsi, P. S., and Taheri, P. (2018). Crime
analysis through machine learning. In 2018 IEEE
9th Annual Information Technology, Electronics and
Mobile Communication Conf. (IEMCON), pages 415–
420. IEEE.
Kiran, J. and Kaishveen, K. (2018). Prediction analysis of
crime in india using a hybrid clustering approach. In
2018 2nd International Conf. on I-SMAC (IoT in So-
cial, Mobile, Analytics and Cloud), pages 520–523.
IEEE.
Kushner, M. G., Riggs, D. S., Foa, E. B., and Miller, S. M.
(1993). Perceived controllability and the development
of posttraumatic stress disorder (ptsd) in crime vic-
tims. Behaviour research and therapy, 31(1):105–
110.
Lin, Y.-L., Yen, M.-F., and Yu, L.-C. (2018). Grid-based
crime prediction using geographical features. ISPRS
International J. Geo-Information, 7(8):298.
Mart
´
ınez-Ram
´
on, M. and Christodoulou, C. (2005). Sup-
port vector machines for antenna array processing and
electromagnetics. Synthesis Lectures on Computa-
tional Electromagnetics, 1(1):1–120.
May, S., Isafiade, O., and Ajayi, O. (2021a). An enhanced
na
¨
ıve bayes model for crime prediction using recur-
sive feature elimination. New York, NY, USA. Asso-
ciation for Computing Machinery.
May, S., Isafiade, O., and Ajayi, O. (2021b). Hybridizing
extremely randomized trees with bootstrap aggrega-
tion for crime prediction. New York, NY, USA. Asso-
ciation for Computing Machinery.
Mohd, F., Noor, N. M. M., et al. (2017). A comparative
study to evaluate filtering methods for crime data fea-
ture selection. Procedia computer science, 116:113–
120.
Nitta, G. R., Rao, B. Y., Sravani, T., Ramakrishiah, N., and
Balaanand, M. (2019). Lasso-based feature selection
and na
¨
ıve bayes classifier for crime prediction and its
type. Service Oriented Computing and Applications,
13(3):187–197.
Rodriguez, C. D. R., Gomez, D. M., and Rey, M. A. M.
(2017). Forecasting time series from clustering by a
memetic differential fuzzy approach: An application
to crime prediction. In 2017 IEEE Symposium Se-
ries on Computational Intelligence (SSCI), pages 1–8.
IEEE.
Sivaranjani, S., Sivakumari, S., and Aasha, M. (2016).
Crime prediction and forecasting in tamilnadu using
clustering approaches. In 2016 International Conf. on
Emerging Technological Trends (ICETT), pages 1–6.
IEEE.
Steinwart, I. and Christmann, A. (2008). Support vector
machines. Springer Science & Business Media.
Sylvester, E. V. A., Bentzen, P., Bradbury, I. R., Cl
´
ement,
M., Pearce, J., Horne, J., and Beiko, R. G. (2018).
Applications of random forest feature selection for
fine-scale genetic population assignment. Evolution-
ary Applications, 11(2):153–165.
Vapnik, V. N. (1999). An overview of statistical learning
theory, volume 10. IEEE.
Wang, L. (2005). Support vector machines: theory and ap-
plications, volume 177. Springer Science & Business
Media.
Zaidi, N. A. S., Mustapha, A., Mostafa, S. A., and Razali,
M. N. (2019). A classification approach for crime pre-
diction. In International Conf. on Applied Comput-
ing to Support Industry: Innovation and Technology,
pages 68–78. Springer.
Zhu, Y. et al. (2018). Comparison of model perfor-
mance for basic and advanced modeling approaches
to crime prediction. Intelligent Information Manage-
ment, 10(06):123.
An Improved Support Vector Model with Recursive Feature Elimination for Crime Prediction
203