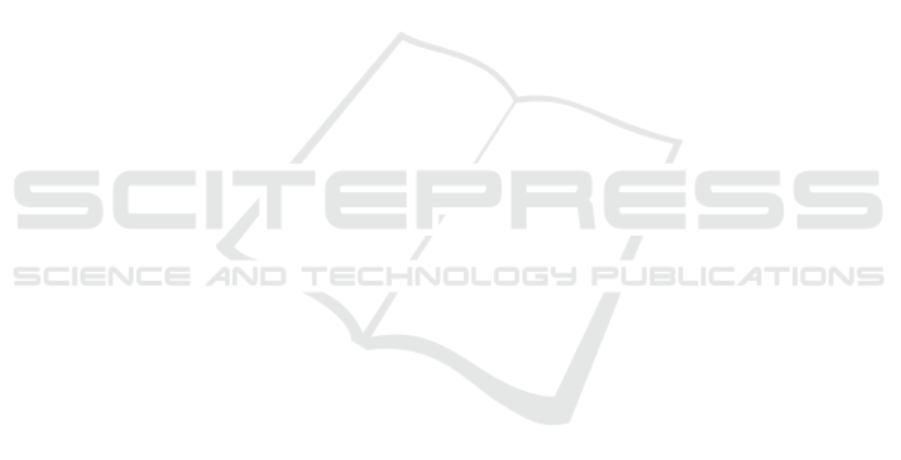
a database created for this paper with tweets from
2022. The results show that is possible to identify
relevant topics for companies and how these topics
change over time.
We would like to explore more tweets with a big-
ger time range to have a better view of emerging top-
ics on Twitter and for how long they stay relevant.
Also, a technique that can analyze tweets in real-time
would be interesting to provide information to the
company that is being analyzed at the same moment
that the users are talking about a topic. These could
improve the actions that a company could take about
what is being said about it.
As future work we plan to reproduce the method-
ology during the Brazilian election period to under-
stand what Twitter users are discussing about the can-
didates. It is a great opportunity since elections are a
subject widely discussed, providing us a big quantity
of tweets.
REFERENCES
Agrawal, R. and Srikant, R. (1994). Fast algorithms for
mining association rules in large databases. In Pro-
ceedings of the 20th International Conference on Very
Large Data Bases, VLDB ’94, pages 487–499, San
Francisco, CA, USA. Morgan Kaufmann Publishers
Inc.
Amig
´
o, E., Carrillo-de Albornoz, J., Chugur, I., Corujo, A.,
Gonzalo, J., Mart
´
ın, T., Meij, E., Rijke, M., and Spina,
D. (2013). Overview of replab 2013: Evaluating on-
line reputation monitoring systems. volume 1179.
Arca, A., Carta, S., Giuliani, A., Stanciu, M., and Refor-
giato Recupero, D. (2020). Automated tag enrichment
by semantically related trends. pages 183–193.
Castellanos, A., Cigarr
´
an, J., and Garc
´
ıa-Serrano, A.
(2017). Formal concept analysis for topic detection:
A clustering quality experimental analysis. Informa-
tion Systems, 66:24–42.
Cataldi, M., Di Caro, L., and Schifanella, C. (2010). Emerg-
ing topic detection on twitter based on temporal and
social terms evaluation. In Proceedings of the Tenth
International Workshop on Multimedia Data Mining,
MDMKDD ’10, New York, NY, USA. Association for
Computing Machinery.
Cigarr
´
an, J.,
´
Angel Castellanos, and Garc
´
ıa-Serrano, A.
(2016). A step forward for topic detection in twitter:
An fca-based approach. Expert Systems with Applica-
tions, 57:21–36.
Contreras, J. O., Hilles, S., and Abubakar, Z. B. (2018).
Automated essay scoring with ontology based on text
mining and nltk tools. In 2018 International Confer-
ence on Smart Computing and Electronic Enterprise
(ICSCEE), pages 1–6.
Ganter, B., Stumme, G., and Wille, R., editors (2005). For-
mal Concept Analysis: Foundations and Applications.
Springer.
Ganter, B. and Wille, R. (1999). Formal concept analy-
sis: mathematical foundations. Springer, Berlin; New
York.
Kwuida, L., Missaoui, R., Amor, B. B., Boumedjout, L.,
and Vaillancourt, J. (2010). Restrictions on concept
lattices for pattern management. In Kryszkiewicz,
M. and Obiedkov, S. A., editors, Proceedings of the
7th International Conference on Concept Lattices and
Their Applications, Sevilla, Spain, October 19-21,
2010, volume 672 of CEUR Workshop Proceedings,
pages 235–246. CEUR-WS.org.
Miao, Z., Chen, K., Fang, Y., He, J., Zhou, Y., Zhang,
W., and Zha, H. (2016). Cost-effective online trend-
ing topic detection and popularity prediction in mi-
croblogging. ACM Trans. Inf. Syst., 35(3).
Roark, B., Saraclar, M., and Collins, M. (2007). Discrimi-
native n-gram language modeling. Computer Speech
Language, 21(2):373–392.
SM., D., C., S., and S¸otropa DF. (2017). An investigation of
user behavior in educational platforms using temporal
concept analysis. In ICFCA 2017.
Zhang, J., Liu, D., Ong, K.-L., Li, Z., and Li, M. (2012).
Detecting topic labels for tweets by matching features
from pseudo-relevance feedback. In Proceedings of
the Tenth Australasian Data Mining Conference - Vol-
ume 134, AusDM ’12, page 9–19, AUS. Australian
Computer Society, Inc.
Temporal Evolution of Topics on Twitter
119