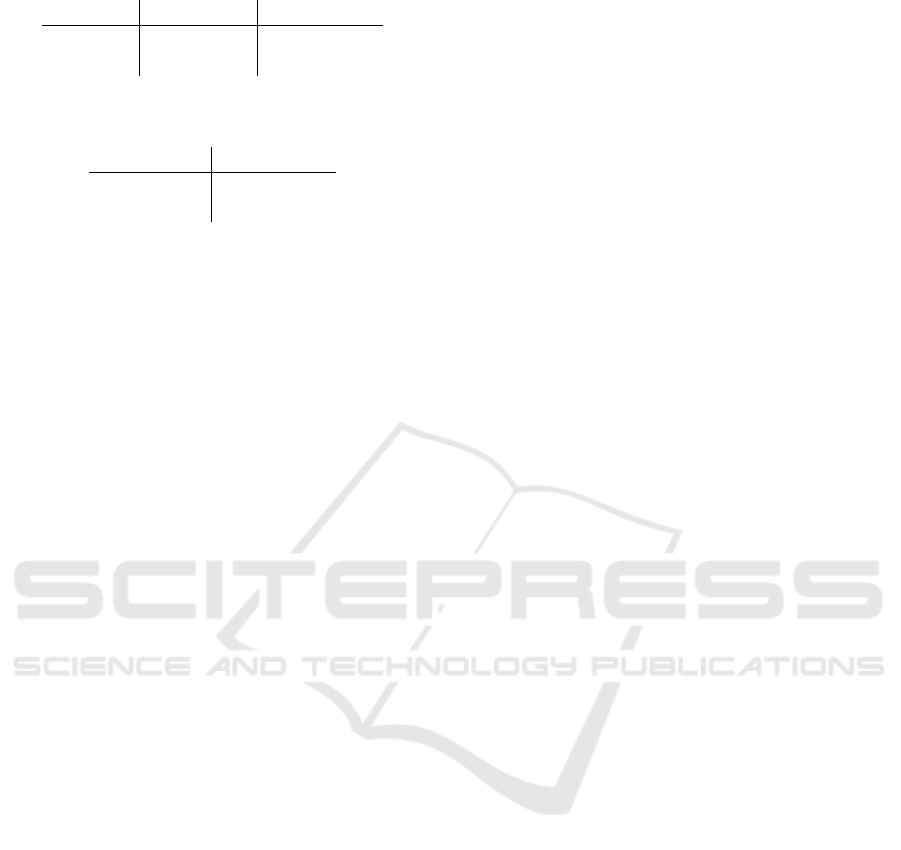
Table 2: CMJ results.
Cluster Cluster size Winner
Cluster 1 33 Bovine meat
Cluster 2
30 Tuna
Table 3: Top 2 of single-winner Majority Judgement ap-
plied to voters.
Ranking MJ Candidate
1 Bovine meat
2 Chicken
We can compare CMJ results with single-winner
MJ ranking, comparing Table 3 3 and Table 2 2.
Expressed judgements are very polarizing and the
two formed cluster seems in opposition between each
other, since the most preferred dishes for one are the
most negatively judged by the other. For this rea-
son, we notice both for Majority Judgement and Clus-
tered Majority Judgement the tendency to avoid the
favourite dishes, focusing on the moderate ones.
In case of Majority Judgement, the solution is
Bovine meat and Chicken, where both alternatives
are considered not acceptable for cluster 2 and for
this reason, 29 dishes would be discarded. With clus-
tered approach, the solution takes into account cluster
2’s preferences, and provides a lower number of dis-
carded dishes.
4 CONCLUSIONS
In section 1, we dealt with logical issues involved in
voting rules and judgement aggregation, highlighting
majority rule’s resistance to strategical vote.
In section 2, a more fined model of majority rule,
Majority Judgement, has been presented as an option
to better estimate the most shared candidate.
In section 3, the related works have been shown
and in section 4, all possible categories of cluster-
ing approach has been reported in order to choose the
fittest one for our generalization of Majority Judge-
ment as a multi-winner strategy. After that, a case
study is reported, with a particular attention to the
comparison between MJ and CMJ results.
The CMJ, as shown, represents the optimal com-
promise in case of polarized groups (clusters), so, in
this situations, this method could be the preferable
choice in order to take into account minorities judge-
ments.
In spite of non-deterministic nature of K-Medoids,
Clustered Majority Judgement is thought to be used
in high populated disputes. For these reasons, we
feel confident about clustering’s role of taking into
account all different perspectives could be shown in
such situation.
Moreover, our implementation is not strictly
linked to political field, as is clearly shown in the
case studies (except the first one), mostly because it
requires only some fixed parameters: number of win-
ners, number of grades and grades themselves.
An important future challenge could be speeding
up the algorithm or making a more flexible structure,
even though all the constraints already explained in
previous sections need to be satisfied.
REFERENCES
Andrea Loreggia, Nicholas Mattei, S. Q. (2020). Artificial
intelligence research for fighting. In Political Polari-
sation: A Research Agenda.
Arrow, K. J. (2012). Social choice and individual values.
Yale university press.
Balinski, M. (2006). Fair majority voting (or how to elimi-
nate gerrymandering). In The American Mathematical
Monthly, Vol. 115, No. 2. Mathematical Association of
America.
Balinski, M. and Laraki, R. (2007). A theory of measuring,
electing, and ranking. National Acad Sciences.
Balinski, M. and Laraki, R. (2011). Election by major-
ity judgment: experimental evidence. In In Situ and
Laboratory Experiments on Electoral Law Reform.
Springer.
Balinski, M. and Laraki, R. (2014). Judge: Don’t vote!
volume 62, pages 483–511. INFORMS.
Brandt, F., Conitzer, V., Endriss, U., Lang, J., and Procac-
cia, A. D. (2016). Handbook of computational social
choice. Cambridge University Press.
Caroprese, L. and Zumpano, E. (2020). Declarative seman-
tics for P2P data management system. J. Data Se-
mant., 9(4):101–122.
Ceci, M., Corizzo, R., Fumarola, F., Ianni, M., Malerba,
D., Maria, G., Masciari, E., Oliverio, M., and
Rashkovska, A. (2015). Big data techniques for
supporting accurate predictions of energy production
from renewable sources. In Desai, B. C. and Toyama,
M., editors, Proceedings of the 19th International
Database Engineering & Applications Symposium,
Yokohama, Japan, July 13-15, 2015, pages 62–71.
ACM.
Fabre, A. (2018). Tie-breaking the highest median: Alter-
natives to the majority judgment. In Paris School of
Economics.
Fazzinga, B., Flesca, S., Furfaro, F., and Masciari, E.
(2013). Rfid-data compression for supporting aggre-
gate queries. ACM Trans. Database Syst., 38(2):11.
G. Bellec, F. Scherr, A. S. (2020). A solution to the learning
dilemma for recurrent networks of spiking neurons. In
Nat Commun.
Hae-Sang Park, C.-H. J. (2008). A simple and fast algorithm
for k-medoids clustering. In POSTECH. Elsevier.
Decision Making with Clustered Majority Judgment
139