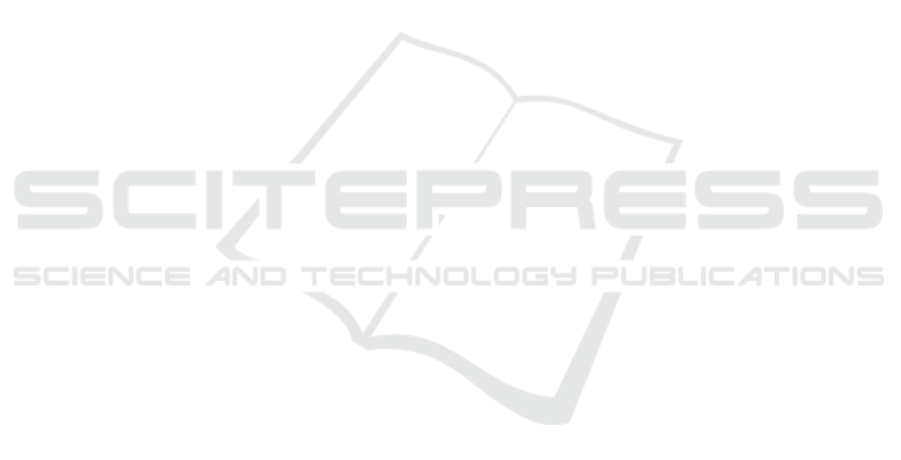
given the feature vectors representing samples. The
centers of the hidden neurons capture similar clusters
of the training data from a given class. The com-
plex phenomenon of thought patterns is handled ef-
ficiently using the proposed algorithm. Our algorithm
outperforms those with an accuracy of 71.45% for
subject-independent motor imagery task classification
for the dataset IIa. The mean kappa value for subject-
dependent task classification is obtained as 0.59 for
the same dataset. The algorithm also performed well
for dataset IIb. In the future, we will explore the
potential of this algorithm to work with more com-
plex thought classes such as music and mathematic
problem solving. We plan to use the transfer learning
methods for other mental activity recognition.
REFERENCES
Agarwal, V. (2019). Adaptive radial basis functions neu-
ral network for motor imagery task classification. In
2019 Twelfth International Conference on Contempo-
rary Computing (IC3), pages 1–6. IEEE.
Alam, M. N., Ibrahimy, M. I., and Motakabber, S. (2021).
Feature extraction of eeg signal by power spectral den-
sity for motor imagery based bci. In 2021 8th Interna-
tional Conference on Computer and Communication
Engineering (ICCCE), pages 234–237. IEEE.
Ang, K. K., Chin, Z. Y., Wang, C., Guan, C., and Zhang, H.
(2012). Filter bank common spatial pattern algorithm
on bci competition iv datasets 2a and 2b. Frontiers in
neuroscience, 6:39.
Baig, M. Z., Aslam, N., and Shum, H. P. (2020). Filtering
techniques for channel selection in motor imagery eeg
applications: a survey. Artificial intelligence review,
53(2):1207–1232.
Bhatti, M. H., Khan, J., Khan, M. U. G., Iqbal, R., Alo-
qaily, M., Jararweh, Y., and Gupta, B. (2019). Soft
computing-based eeg classification by optimal feature
selection and neural networks. IEEE Transactions on
Industrial Informatics, 15(10):5747–5754.
Das, A. and Suresh, S. (2015). An effect-size based chan-
nel selection algorithm for mental task classification
in brain computer interface. In 2015 IEEE Interna-
tional Conference on Systems, Man, and Cybernetics,
pages 3140–3145. IEEE.
Davoudi, A., Ghidary, S. S., and Sadatnejad, K. (2017). Di-
mensionality reduction based on distance preservation
to local mean for symmetric positive definite matrices
and its application in brain–computer interfaces. Jour-
nal of neural engineering, 14(3):036019.
Haykin, S. (2005). Neural Networks. PEARSON.
Kim, J.-Y., Park, S.-M., Ko, K.-E., and Sim, K.-B. (2012).
A binary pso-based optimal eeg channel selection
method for a motor imagery based bci system. In In-
ternational Conference on Hybrid Information Tech-
nology, pages 245–252. Springer.
Ko, W., Yoon, J., Kang, E., Jun, E., Choi, J.-S., and Suk,
H.-I. (2018). Deep recurrent spatio-temporal neural
network for motor imagery based bci. In 2018 6th In-
ternational Conference on Brain-Computer Interface
(BCI), pages 1–3. IEEE.
Miao, M., Zeng, H., Wang, A., Zhao, C., and Liu, F.
(2017). Discriminative spatial-frequency-temporal
feature extraction and classification of motor im-
agery eeg: An sparse regression and weighted na
¨
ıve
bayesian classifier-based approach. Journal of neuro-
science methods, 278:13–24.
Nicolas-Alonso, L. F. and Gomez-Gil, J. (2012). Brain
computer interfaces, a review. sensors, 12(2):1211–
1279.
Qiu, Z., Jin, J., Lam, H.-K., Zhang, Y., Wang, X., and Ci-
chocki, A. (2016). Improved sffs method for channel
selection in motor imagery based bci. Neurocomput-
ing, 207:519–527.
Sheoran, P. and Saini, J. (2022). Optimizing channel selec-
tion using multi-objective fodpso for bci applications.
Brain-Computer Interfaces, 9(1):7–22.
Xie, X., Yu, Z. L., Lu, H., Gu, Z., and Li, Y. (2016).
Motor imagery classification based on bilinear sub-
manifold learning of symmetric positive-definite ma-
trices. IEEE Transactions on Neural Systems and Re-
habilitation Engineering, 25(6):504–516.
Zhang, C. and Eskandarian, A. (2020). A computation-
ally efficient multiclass time-frequency common spa-
tial pattern analysis on eeg motor imagery. In 2020
42nd Annual International Conference of the IEEE
Engineering in Medicine & Biology Society (EMBC),
pages 514–518. IEEE.
Zhang, L. and Wei, Q. (2019). Channel selection in motor
imaginary-based brain-computer interfaces: a particle
swarm optimization algorithm. Journal of integrative
neuroscience, 18(2):141–152.
Zhao, H., Zheng, Q., Ma, K., Li, H., and Zheng, Y.
(2020). Deep representation-based domain adapta-
tion for nonstationary eeg classification. IEEE Trans-
actions on Neural Networks and Learning Systems,
32(2):535–545.
Zhou, B., Wu, X., Ruan, J., Zhao, L., and Zhang, L. (2019).
How many channels are suitable for independent com-
ponent analysis in motor imagery brain-computer in-
terface. Biomedical Signal Processing and Control,
50:103–120.
NCTA 2022 - 14th International Conference on Neural Computation Theory and Applications
358