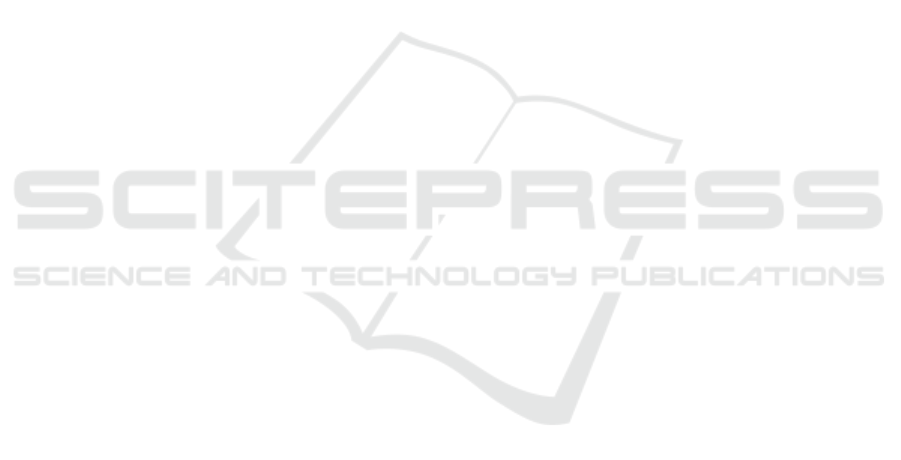
Godard, C., Mac Aodha, O., and Brostow, G. J. (2017). Un-
supervised monocular depth estimation with left-right
consistency. In Proceedings of the IEEE/CVF Con-
ference on Computer Vision and Pattern Recognition
(CVPR), pages 270–279.
J
¨
orgensen, E., Zach, C., and Kahl, F. (2019). Monocu-
lar 3d object detection and box fitting trained end-to-
end using intersection-over-union loss. arXiv preprint
arXiv:1906.08070.
Kalman, R. E. et al. (1960). A new approach to linear fil-
tering and prediction problems. Journal of Basic En-
gineering, 82(1):35–45.
Kim, Y. and Kum, D. (2019). Deep learning based vehi-
cle position and orientation estimation via inverse per-
spective mapping image. In IEEE Intelligent Vehicles
Symposium (IV), pages 317–323.
Kuhn, H. W. (1955). The hungarian method for the assign-
ment problem. Naval Research Logistics Quarterly,
2(1-2):83–97.
Law, H. and Deng, J. (2018). Cornernet: Detecting objects
as paired keypoints. In European Conference on Com-
puter Vision (ECCV), pages 734–750.
Liu, Y., Yixuan, Y., and Liu, M. (2021). Ground-
aware monocular 3d object detection for autonomous
driving. IEEE Robotics and Automation Letters,
6(2):919–926.
Liu, Z., Wu, Z., and T
´
oth, R. (2020). Smoke: Single-stage
monocular 3d object detection via keypoint estima-
tion. In IEEE/CVF Conference on Computer Vision
and Pattern Recognition Workshops (CVPRW), pages
4289–4298.
Luiten, J., Os Ep, A. A., Dendorfer, P., Torr, P., Geiger, A.,
Leal-Taix
´
e, L., and Leibe, B. (2021). Hota: A higher
order metric for evaluating multi-object tracking. In-
ternational Journal of Computer Vision, 129(2):548–
578.
Redmon, J., Divvala, S., Girshick, R., and Farhadi, A.
(2016). You only look once: Unified, real-time ob-
ject detection. In Proceedings of the IEEE/CVF Con-
ference on Computer Vision and Pattern Recognition
(CVPR), pages 779–788.
Redmon, J. and Farhadi, A. (2017). Yolo9000: better, faster,
stronger. In Proceedings of the IEEE conference on
computer vision and pattern recognition, pages 7263–
7271.
Redmon, J. and Farhadi, A. (2018). Yolov3: An incremental
improvement. arXiv preprint arXiv:1804.02767.
Ren, S., He, K., Girshick, R., and Sun, J. (2017). Faster r-
cnn: Towards real-time object detection with region
proposal networks. IEEE Transactions on Pattern
Analysis and Machine Intelligence, 39(6):1137–1149.
Roddick, T., Kendall, A., and Cipolla, R. (2018). Ortho-
graphic feature transform for monocular 3d object de-
tection. arXiv preprint arXiv:1811.08188.
Srivastava, S., Jurie, F., and Sharma, G. (2019). Learning 2d
to 3d lifting for object detection in 3d for autonomous
vehicles. In IEEE/RSJ International Conference on
Intelligent Robots and Systems (IROS), pages 4504–
4511.
Szeliski, R. (2022). Computer Vision. Springer Interna-
tional Publishing, Cham.
Vaswani, A., Shazeer, N., Parmar, N., Uszkoreit, J., Jones,
L., Gomez, A. N., Kaiser, Ł., and Polosukhin, I.
(2017). Attention is all you need. Advances in Neural
Information Processing Systems, 30.
Voigtlaender, P., Krause, M., Osep, A., Luiten, J., Sekar, B.
B. G., Geiger, A., and Leibe, B. (2019). Mots: Multi-
object tracking and segmentation. In Proceedings of
the IEEE/CVF Conference on Computer Vision and
Pattern Recognition (CVPR), pages 7942–7951.
Wang, Z., Zheng, L., Liu, Y., Li, Y., and Wang, S. (2020).
Towards real-time multi-object tracking. In European
Conference on Computer Vision (ECCV), pages 107–
122.
Weng, X. and Kitani, K. (2019). Monocular 3d object de-
tection with pseudo-lidar point cloud. In IEEE/CVF
International Conference on Computer Vision Work-
shop (ICCVW), pages 857–866.
Weng, X., Wang, J., Held, D., and Kitani, K. (2020).
Ab3dmot: A baseline for 3d multi-object track-
ing and new evaluation metrics. arXiv preprint
arXiv:2008.08063.
Yu, F., Wang, D., Shelhamer, E., and Darrell, T. (2018).
Deep layer aggregation. In Proceedings of the
IEEE/CVF Conference on Computer Vision and Pat-
tern Recognition (CVPR), pages 2403–2412.
Zhang, Y., Lu, J., and Zhou, J. (2021a). Objects are differ-
ent: Flexible monocular 3d object detection. In Pro-
ceedings of the IEEE/CVF Conference on Computer
Vision and Pattern Recognition (CVPR), pages 3289–
3298.
Zhang, Y., Ma, X., Yi, S., Hou, J., Wang, Z., Ouyang, W.,
and Xu, D. (2021b). Learning geometry-guided depth
via projective modeling for monocular 3d object de-
tection. arXiv preprint arXiv:2107.13931.
Zhang, Y., Wang, C., Wang, X., Zeng, W., and Liu, W.
(2021c). Fairmot: On the fairness of detection and
re-identification in multiple object tracking. Inter-
national Journal of Computer Vision, 129(11):3069–
3087.
Zhou, X., Wang, D., and Kr
¨
ahenb
¨
uhl, P. (2019). Objects as
points. arXiv preprint arXiv:1904.07850.
Zhu, X., Hu, H., Lin, S., and Dai, J. (2019). Deformable
convnets v2: More deformable, better results. In Pro-
ceedings of the IEEE/CVF Conference on Computer
Vision and Pattern Recognition (CVPR), pages 9308–
9316.
Zhu, X., Su, W., Lu, L., Li, B., Wang, X., and Dai, J.
(2020). Deformable detr: Deformable transform-
ers for end-to-end object detection. arXiv preprint
arXiv:2010.04159.
ROBOVIS 2022 - Workshop on Robotics, Computer Vision and Intelligent Systems
434