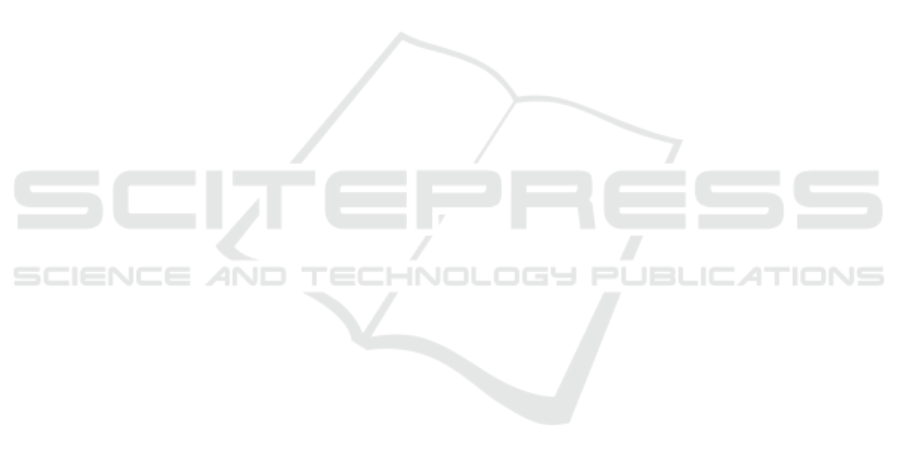
Bouktif, S., Fiaz, A., Ouni, A., and Serhani, M. A.
(2018). Optimal deep learning lstm model for elec-
tric load forecasting using feature selection and ge-
netic algorithm: Comparison with machine learning
approaches. Energies, 11(7):1636.
Chen, T. and Guestrin, C. (2016). Xgboost: A scalable
tree boosting system. In Proceedings of the 22nd
ACM SIGKDD International Conference on Knowl-
edge Discovery and Data Mining, KDD ’16, page
785–794, New York, NY, USA. Association for Com-
puting Machinery.
Deschepper, M., Eeckloo, K., Malfait, S., Benoit, D., Cal-
lens, S., and Vansteelandt, S. (2021). Prediction of
hospital bed capacity during the covid- 19 pandemic.
BMC health services research, 21(1):1–10.
Dineva, A., Csom
´
os, B., Sz, S. K., and Vajda, I. (2021).
Investigation of the performance of direct forecasting
strategy using machine learning in state-of-charge pre-
diction of li-ion batteries exposed to dynamic loads.
Journal of Energy Storage, 36:102351.
Dossani, A. (2022). Inference in direct multi-step and long
horizon forecasting regressions. Available at SSRN
4020488.
Du, P., Jin, Y., and Zhang, K. (2016). A hybrid multi-step
rolling forecasting model based on ssa and simulated
annealing—adaptive particle swarm optimization for
wind speed. Sustainability, 8(8):754.
Feng, B., Xu, J., Zhang, Y., and Lin, Y. (2021). Multi-
step traffic speed prediction based on ensemble learn-
ing on an urban road network. Applied Sciences,
11(10):4423.
Friedman, J. H. (2001). Greedy function approximation: a
gradient boosting machine. Annals of statistics, pages
1189–1232.
Geurts, P., Ernst, D., and Wehenkel, L. (2006). Extremely
randomized trees. Machine learning, 63(1):3–42.
Goic, M., Bozanic-Leal, M. S., Badal, M., and Basso, L. J.
(2021). Covid-19: Short-term forecast of icu beds in
times of crisis. Plos one, 16(1):e0245272.
Herrer
´
ıa-Alonso, S., Su
´
arez-Gonz
´
alez, A., Rodr
´
ıguez-
P
´
erez, M., Rodr
´
ıguez-Rubio, R. F., and L
´
opez-
Garc
´
ıa, C. (2021). Efficient wind speed forecast-
ing for resource-constrained sensor devices. Sensors,
21(3):983.
Hornik, K., Stinchcombe, M., and White, H. (1989). Multi-
layer feedforward networks are universal approxima-
tors. Neural networks, 2(5):359–366.
James, G., Witten, D., Hastie, T., and Tibshirani, R. (2021).
Statistical Learning, pages 15–57. Springer US, New
York, NY.
Ke, G., Meng, Q., Finley, T., Wang, T., Chen, W., Ma, W.,
Ye, Q., and Liu, T.-Y. (2017). Lightgbm: A highly
efficient gradient boosting decision tree. Advances in
neural information processing systems, 30.
Khalid, M. (2019). Wind power economic dispatch–impact
of radial basis functional networks and battery energy
storage. IEEE Access, 7:36819–36832.
Lee, M. (2018). Modeling and forecasting hotel room de-
mand based on advance booking information. Tourism
Management, 66:62–71.
Liu, D., Lu, J., and Ma, W. (2021a). Real-time return de-
mand prediction based on multisource data of one-
way carsharing systems. Journal of Advanced Trans-
portation, 2021.
Liu, Y., Wang, H., Feng, W., and Huang, H. (2021b). Short
term real-time rolling forecast of urban river water
levels based on lstm: A case study in fuzhou city,
china. International Journal of Environmental Re-
search and Public Health, 18(17):9287.
Mu, B., Peng, C., Yuan, S., and Chen, L. (2019). Enso
forecasting over multiple time horizons using convl-
stm network and rolling mechanism. In 2019 inter-
national joint conference on neural networks (ijcnn),
pages 1–8. IEEE.
M
¨
uller, J. and Bogenberger, K. (2015). Time series analysis
of booking data of a free-floating carsharing system
in berlin. Transportation Research Procedia, 10:345–
354.
Shi, X. and Yeung, D.-Y. (2018). Machine learning for spa-
tiotemporal sequence forecasting: A survey. arXiv
preprint arXiv:1808.06865.
Sun, S., Wu, H., and Xiang, L. (2020). City-wide traf-
fic flow forecasting using a deep convolutional neural
network. Sensors, 20(2):421.
Surakhi, O., Zaidan, M. A., Fung, P. L., Hossein Motlagh,
N., Serhan, S., AlKhanafseh, M., Ghoniem, R. M.,
and Hussein, T. (2021). Time-lag selection for time-
series forecasting using neural network and heuristic
algorithm. Electronics, 10(20):2518.
Taieb, S. B., Bontempi, G., Atiya, A. F., and Sorjamaa, A.
(2012). A review and comparison of strategies for
multi-step ahead time series forecasting based on the
nn5 forecasting competition. Expert systems with ap-
plications, 39(8):7067–7083.
Vassallo, D., Krishnamurthy, R., Sherman, T., and Fer-
nando, H. J. (2020). Analysis of random forest mod-
eling strategies for multi-step wind speed forecasting.
Energies, 13(20):5488.
Wang, C.-C., Chien, C.-H., and Trappey, A. J. (2021a). On
the application of arima and lstm to predict order de-
mand based on short lead time and on-time delivery
requirements. Processes, 9(7):1157.
Wang, Y., Zhang, N., and Chen, X. (2021b). A short-term
residential load forecasting model based on lstm re-
current neural network considering weather features.
Energies, 14(10):2737.
Yu, D., Li, Z., Zhong, Q., Ai, Y., and Chen, W. (2020). De-
mand management of station-based car sharing sys-
tem based on deep learning forecasting. Journal of
Advanced Transportation, 2020.
Yuan, S., Luo, X., Mu, B., Li, J., and Dai, G. (2019). Pre-
diction of north atlantic oscillation index with convo-
lutional lstm based on ensemble empirical mode de-
composition. Atmosphere, 10(5):252.
Yuan, S., Wang, C., Mu, B., Zhou, F., and Duan, W. (2021).
Typhoon intensity forecasting based on lstm using the
rolling forecast method. Algorithms, 14(3):83.
Zou, H. and Hastie, T. (2003). Regression shrinkage and
selection via the elastic net, with applications to mi-
croarrays. JR Stat Soc Ser B, 67:301–20.
NCTA 2022 - 14th International Conference on Neural Computation Theory and Applications
366