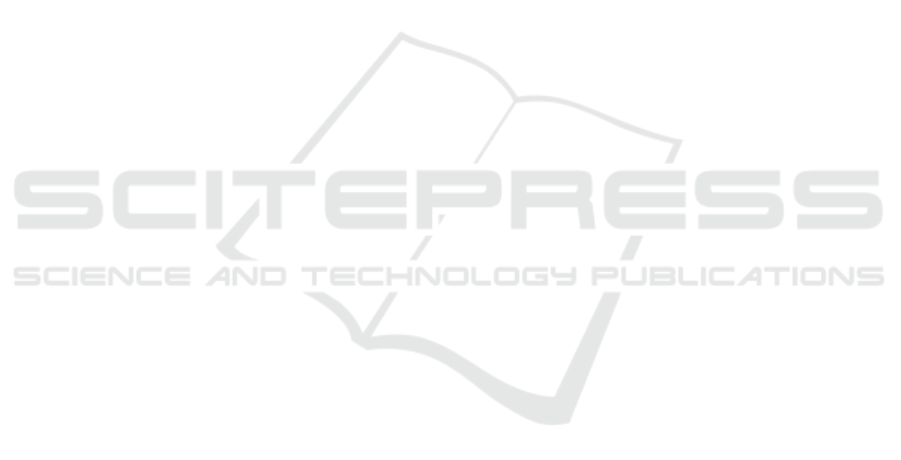
Dautov, R. and Distefano, S. (2017). Three-level hierarchi-
cal data fusion through the IoT, edge, and cloud com-
puting. In Proceedings of the 1st International Con-
ference on Internet of Things and Machine Learning,
pages 1–5.
Dautov, R. and Distefano, S. (2019). Automating IoT data-
intensive application allocation in clustered edge com-
puting. IEEE Transactions on Knowledge and Data
Engineering, 33(1):55–69.
Dautov, R., Distefano, S., Bruneo, D., Longo, F., Mer-
lino, G., and Puliafito, A. (2017). Pushing intelli-
gence to the edge with a stream processing architec-
ture. In 2017 IEEE International Conference on Inter-
net of Things (iThings) and IEEE Green Computing
and Communications (GreenCom) and IEEE Cyber,
Physical and Social Computing (CPSCom) and IEEE
Smart Data (SmartData), pages 792–799. IEEE.
Dautov, R., Distefano, S., and Buyya, R. (2019). Hierarchi-
cal data fusion for Smart Healthcare. Journal of Big
Data, 6(1):1–23.
De Vries, J., Michielsen, H. J., and Van Heck, G. L. (2003).
Assessment of fatigue among working people: a com-
parison of six questionnaires. Occupational and envi-
ronmental medicine, 60(suppl 1):i10–i15.
Gander, P., Hartley, L., Powell, D., Cabon, P., Hitchcock,
E., Mills, A., and Popkin, S. (2011). Fatigue risk man-
agement: Organizational factors at the regulatory and
industry/company level. Accident Analysis & Preven-
tion, 43(2):573–590.
Ganster, D. C. and Rosen, C. C. (2013). Work stress and
employee health: A multidisciplinary review. Journal
of management, 39(5):1085–1122.
Gordon, R. P. (1998). The contribution of human factors
to accidents in the offshore oil industry. Reliability
Engineering & System Safety, 61(1-2):95–108.
Hallman, D. M., Gupta, N., Mathiassen, S. E., and Holter-
mann, A. (2015). Association between objectively
measured sitting time and neck–shoulder pain among
blue-collar workers. International Archives of Oc-
cupational and Environmental Health, 88(8):1031–
1042.
Hamy, V., Garcia-Gancedo, L., Pollard, A., Myatt, A.,
Liu, J., Howland, A., Beineke, P., Quattrocchi, E.,
Williams, R., and Crouthamel, M. (2020). Develop-
ing smartphone-based objective assessments of phys-
ical function in rheumatoid arthritis patients: the PA-
RADE study. Digital biomarkers, 4(1):26–44.
Hellesøy, O. H. (1985). Work Environment, Statfjord Field:
Work Environment, Health, and Safety on a North Sea
Oil Platform. Universitetsforlaget.
Hochreiter, S. and Schmidhuber, J. (1997). Long short-term
memory. Neural computation, 9(8):1735–1780.
Hockey, R. (2013). The psychology of fatigue: Work, effort
and control. Cambridge University Press.
Hofman, M., Ryan, J. L., Figueroa-Moseley, C. D., Jean-
Pierre, P., and Morrow, G. R. (2007). Cancer-related
fatigue: the scale of the problem. The oncologist,
12(S1):4–10.
Husom, E. J. B. G., Bernab
´
e, P., and Sen, S. (2022). Deep
learning to predict power output from respiratory in-
ductive plethysmography data. Applied AI Letters,
3(2):e65.
Jacobson, N. C., Summers, B., and Wilhelm, S. (2020).
Digital biomarkers of social anxiety severity: digital
phenotyping using passive smartphone sensors. Jour-
nal of medical Internet research, 22(5):e16875.
Kaewkannate, K. and Kim, S. (2016). A comparison of
wearable fitness devices. BMC public health, 16(1):1–
16.
Karuppusamy, N. S. and Kang, B.-Y. (2020). Multi-
modal System to Detect Driver Fatigue Using EEG,
Gyroscope, and Image Processing. IEEE Access,
8:129645–129667.
Kim, D. H., Nam, K. H., Choi, B. K., Han, I. H., Jeon,
T. J., and Park, S. Y. (2019). The Usefulness of a
Wearable Device in Daily Physical Activity Monitor-
ing for the Hospitalized Patients Undergoing Lumbar
Surgery. Journal of Korean Neurosurgical Society,
62(5):561.
Kudo, Y., Arai, M., and Miki, N. (2017). Fatigue assess-
ment by electroencephalogram measured with candle-
like dry microneedle electrodes. Micro & Nano Let-
ters, 12(8):545–549.
Lavidor, M., Weller, A., and Babkoff, H. (2003). How sleep
is related to fatigue. British journal of health psychol-
ogy, 8(1):95–105.
Lee, K. A., Hicks, G., and Nino-Murcia, G. (1991). Validity
and reliability of a scale to assess fatigue. Psychiatry
research, 36(3):291–298.
Leroux, A., Rzasa-Lynn, R., Crainiceanu, C., and Sharma,
T. (2021). Wearable devices: current status and oppor-
tunities in pain assessment and management. Digital
Biomarkers, 5(1):89–102.
Liu, B. (2017). Lifelong machine learning: a paradigm for
continuous learning. Frontiers of Computer Science,
11(3):359–361.
Low, C. A., Li, M., Vega, J., Durica, K. C., Ferreira, D.,
Tam, V., Hogg, M., Zeh III, H., Doryab, A., and Dey,
A. K. (2021). Digital biomarkers of symptom bur-
den self-reported by perioperative patients undergo-
ing pancreatic surgery: prospective longitudinal study.
JMIR cancer, 7(2).
Lu, H., Frauendorfer, D., Rabbi, M., Mast, M. S., Chit-
taranjan, G. T., Campbell, A. T., Gatica-Perez, D., and
Choudhury, T. (2012). StressSense: Detecting stress
in unconstrained acoustic environments using smart-
phones. In Proceedings of the 2012 ACM conference
on ubiquitous computing, pages 351–360.
Luo, H., Lee, P.-A., Clay, I., Jaggi, M., and De Luca, V.
(2020). Assessment of fatigue using wearable sensors:
a pilot study. Digital biomarkers, 4(1):59–72.
Maman, Z. S., Yazdi, M. A. A., Cavuoto, L. A., and Mega-
hed, F. M. (2017). A data-driven approach to model-
ing physical fatigue in the workplace using wearable
sensors. Applied ergonomics, 65:515–529.
Marino, F. E. (2019). Human Fatigue: Evolution, Health
and Performance. Routledge.
Matuz, A., van der Linden, D., Zsolt, K., Hernadi, I.,
Kazmer, K., and Csatho, A. (2021). Enhanced cardiac
vagal tone in mental fatigue: Analysis of heart rate
Machine Learning for Fatigue Detection using Fitbit Fitness Trackers
51