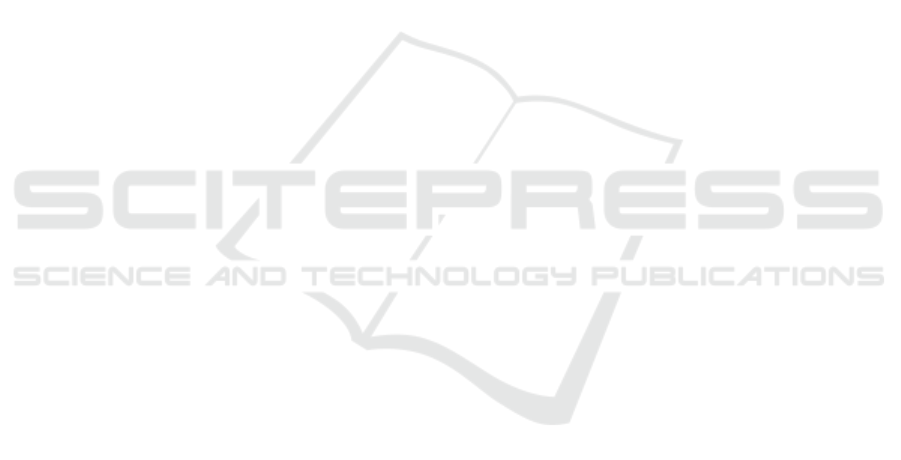
Houreh, Y., Mahdinejad, M., Naredo, E., Dias, D. M., and
Ryan, C. (2021). Hnas: Hyper neural architecture
search for image segmentation. In ICAART (2), pages
246–256.
Kamran, S. A., Hossain, K. F., Tavakkoli, A., Zuckerbrod,
S. L., Sanders, K. M., and Baker, S. A. (2021). Rv-
gan: segmenting retinal vascular structure in fundus
photographs using a novel multi-scale generative ad-
versarial network. In International Conference on
Medical Image Computing and Computer-Assisted In-
tervention, pages 34–44. Springer.
LeCun, Y., Bengio, Y., et al. (1995). Convolutional net-
works for images, speech, and time series. The
handbook of brain theory and neural networks,
3361(10):1995.
Li, L., Verma, M., Nakashima, Y., Nagahara, H., and
Kawasaki, R. (2020). Iternet: Retinal image segmen-
tation utilizing structural redundancy in vessel net-
works. In Proceedings of the IEEE/CVF Winter Con-
ference on Applications of Computer Vision, pages
3656–3665.
Ogurtsova, K., da Rocha Fernandes, J., Huang, Y., Lin-
nenkamp, U., Guariguata, L., Cho, N. H., Cavan, D.,
Shaw, J., and Makaroff, L. (2017). Idf diabetes at-
las: Global estimates for the prevalence of diabetes
for 2015 and 2040. Diabetes research and clinical
practice, 128:40–50.
Popat, V., Mahdinejad, M., Cede
˜
no, O. D., Naredo, E., and
Ryan, C. (2020). Ga-based u-net architecture opti-
mization applied to retina blood vessel segmentation.
In IJCCI, pages 192–199.
Ronneberger, O., Fischer, P., and Brox, T. (2015). U-net:
Convolutional networks for biomedical image seg-
mentation. CoRR, abs/1505.04597.
Shin, S. Y., Lee, S., Yun, I. D., and Lee, K. M. (2019). Deep
vessel segmentation by learning graphical connectiv-
ity. Medical image analysis, 58:101556.
Staal, J. (2018). DRIVE: Digital retinal images for vessel
extraction.
Turing, A. M. (1990). The chemical basis of morphogene-
sis. Bulletin of mathematical biology, 52(1):153–197.
Uysal, E. S., Bilici, M. S¸., Zaza, B. S.,
¨
Ozgenc¸, M. Y.,
and Boyar, O. (2021). Exploring the limits of data
augmentation for retinal vessel segmentation. arXiv
preprint arXiv:2105.09365.
Vaswani, A., Shazeer, N., Parmar, N., Uszkoreit, J., Jones,
L., Gomez, A. N., Kaiser, Ł., and Polosukhin, I.
(2017). Attention is all you need. Advances in neural
information processing systems, 30.
Wang, B., Qiu, S., and He, H. (2019). Dual encoding u-net
for retinal vessel segmentation. In International con-
ference on medical image computing and computer-
assisted intervention, pages 84–92. Springer.
Winder, R. J., Morrow, P. J., McRitchie, I. N., Bailie, J., and
Hart, P. M. (2009). Algorithms for digital image pro-
cessing in diabetic retinopathy. Computerized medical
imaging and graphics, 33(8):608–622.
Woo, S., Park, J., Lee, J.-Y., and Kweon, I. S. (2018). Cbam:
Convolutional block attention module. In Proceed-
ings of the European conference on computer vision
(ECCV), pages 3–19.
Zhou, Y., Yu, H., and Shi, H. (2021). Study group learning:
Improving retinal vessel segmentation trained with
noisy labels. In International Conference on Medi-
cal Image Computing and Computer-Assisted Inter-
vention, pages 57–67. Springer.
Zhou, Z., Rahman Siddiquee, M. M., Tajbakhsh, N., and
Liang, J. (2018). Unet++: A nested u-net architecture
for medical image segmentation. In Deep learning in
medical image analysis and multimodal learning for
clinical decision support, pages 3–11. Springer.
ECTA 2022 - 14th International Conference on Evolutionary Computation Theory and Applications
104