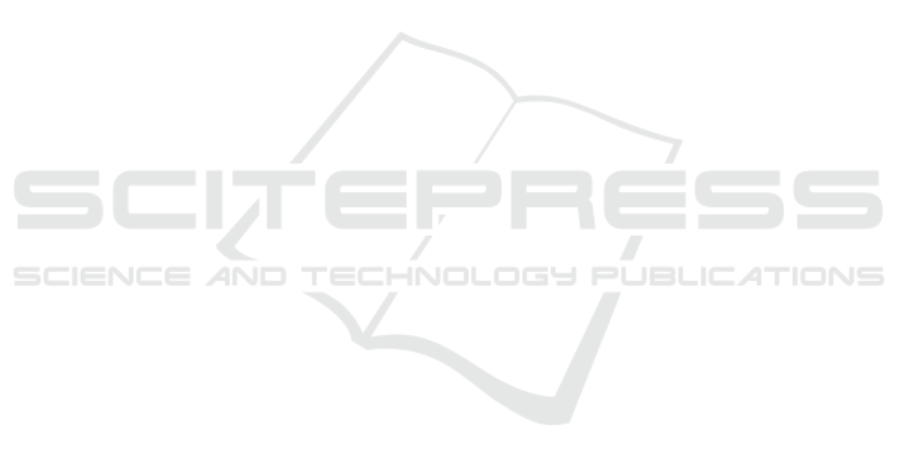
works (Lindsay, 2021). Those two approaches com-
plement the limitations of each other and broaden the
understanding of the phenomenon. We suppose both
the structure of visual system and the function of spa-
tial perception contribute to the appearance of the op-
tical illusions, however we are yet to understand the
interaction of those components while working with
various stimuli. A possible route we can take is to
study the inner states of the model: for example, the
filter weights and the feature maps of a neural model
are much easier to access than the internal states of
living neurons.
5 CONCLUSIONS
We have successfully recreated the M
¨
uller-Lyer illu-
sion in a convolutional neural network that was pre-
trained to estimate heights of the 3D object in a spatial
simulation. Transfer learning was successful and the
model was substantially biased when estimating the
illusion stimuli. We used Bayesian statistics to calcu-
late the impact of the image properties on the estima-
tions of the neural network and tested the model on
unconventional versions of the illusion.
Still, it is necessary to cover additional aspects of
the illusion in the next studies, such as the compar-
ison between the estimations of the neural network
and the mean estimations provided by humans for the
same images. Moreover, convolutional models may
be successfully used with other optical illusions, and
the study of the models’ inner states, as mentioned in
the previous section, can also be fruitful.
REFERENCES
Burns, B. D. and Pritchard, R. (1971). Geometrical illu-
sions and the response of neurones in the cat’s visual
cortex to angle patterns. The Journal of Physiology,
213(3):599–616.
Garc
´
ıa-Garibay, O. B. and de Lafuente, V. (2015). The
M
¨
uller-Lyer illusion as seen by an artificial neural net-
work. Frontiers in Computational Neuroscience, 9.
Gomez-Villa, A., Martin, A., Vazquez-Corral, J., and
Bertalmio, M. (2019). Convolutional Neural Net-
works Can Be Deceived by Visual Illusions. In
Proceedings of the IEEE/CVF Conference on Com-
puter Vision and Pattern Recognition (CVPR), pages
12309–12317.
Gorbunov, I., Pershin, I., Zainutdinov, M., and Koval, V.
(2019). Using the neural model of the person’s se-
mantic space to reveal the occurred with him events.
In 10th International Multi-Conference on Complex-
ity, Informatics and Cybernetics, IMCIC 2019, pages
164–165.
Gregory, R. L. (1963). Distortion of Visual Space as Inap-
propriate Constancy Scaling. Nature, 199(4894):678–
680. Number: 4894 Publisher: Nature Publishing
Group.
Hatwell, Y. (1960). Etude de quelques illusions
g
´
eom
´
etriques tactiles chez les aveugles. L’Ann
´
ee psy-
chologique, 60(1):11–27.
Kubota, Y., Hiyama, A., and Inami, M. (2021). A Ma-
chine Learning Model Perceiving Brightness Optical
Illusions: Quantitative Evaluation with Psychophys-
ical Data. In Augmented Humans Conference 2021,
AHs’21, pages 174–182, New York, NY, USA. As-
sociation for Computing Machinery. event-place:
Rovaniemi, Finland.
Lindsay, G. W. (2021). Convolutional Neural Networks
as a Model of the Visual System: Past, Present,
and Future. Journal of Cognitive Neuroscience,
33(10):2017–2031.
Mamaev, A. N. (2022). MLˆ2: the M
¨
uller-Lyer illusion
Machine Learning model.
Mamaev, A. N. and Gorbunov, I. A. (2021). The M
¨
uller-
Lyer illusion in CNN trained for 3D object height esti-
mation. In Neurotechnologies, pages 159–167. VVM,
St. Petersburg.
Muller-Lyer, F. (1889). Optische urteilstauschungen.
Archiv fur Anatomie und Physiologie, Physiologische
Abteilung, 2:263–270.
Parker, N. I. and Newbigging, P. L. (1963). Magnitude and
decrement of the M
¨
uller-Lyer illusion as a function of
pre-training. Canadian Journal of Psychology/Revue
canadienne de psychologie, 17(1):134–140.
Segall, M. H., Campbell, D. T., and Herskovits, M. J.
(1963). Cultural differences in the perception of ge-
ometric illusions. Science, 139(3556):769–771. Pub-
lisher: American Association for the Advancement of
Science.
Zeman, A., Obst, O., Brooks, K. R., and Rich, A. N.
(2013). The M
¨
uller-Lyer Illusion in a Computational
Model of Biological Object Recognition. PLoS ONE,
8(2):e56126.
Spatial Simulation of the Müller-Lyer Illusion Genesis with Convolutional Neural Networks
291