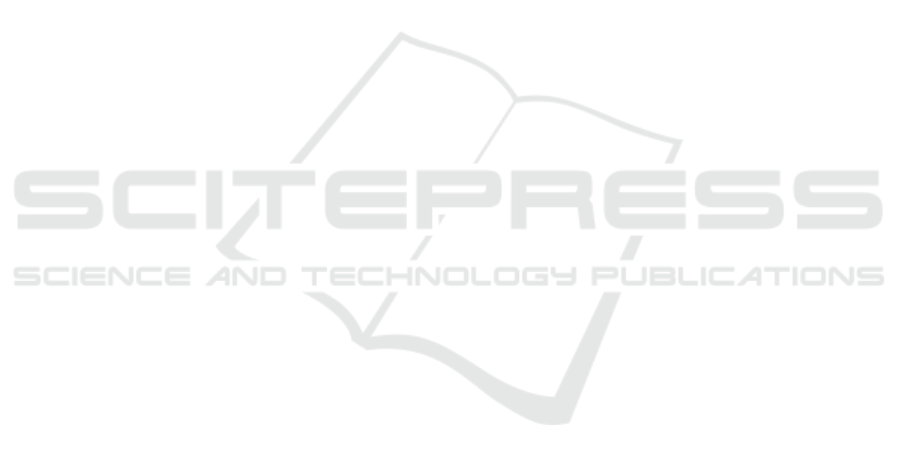
ditional random fields: Probabilistic models for seg-
menting and labeling sequence data.
Lewis, M., Liu, Y., Goyal, N., Ghazvininejad, M., Mo-
hamed, A., Levy, O., Stoyanov, V., and Zettlemoyer,
L. (2020). Bart: Denoising sequence-to-sequence pre-
training for natural language generation, translation,
and comprehension. In Proceedings of the 58th An-
nual Meeting of the Association for Computational
Linguistics, pages 7871–7880.
Liu, Y., Zhang, T., Liang, Z., Ji, H., and McGuinness, D. L.
(2018). Seq2rdf: An end-to-end application for de-
riving triples from natural language text. In CEUR
Workshop Proceedings, volume 2180. CEUR-WS.
M
`
arquez, L., Carreras, X., Litkowski, K. C., and Stevenson,
S. (2008). Semantic role labeling: an introduction to
the special issue.
Martinez-Rodriguez, J. L., Lopez-Arevalo, I., Rios-
Alvarado, A. B., Hernandez, J., and Aldana-
Bobadilla, E. (2019). Extraction of rdf statements
from text. In Iberoamerican Knowledge Graphs and
Semantic Web Conference, pages 87–101. Springer.
Radford, A., Wu, J., Child, R., Luan, D., Amodei, D.,
Sutskever, I., et al. (2019). Language models are un-
supervised multitask learners.
Raffel, C., Shazeer, N., Roberts, A., Lee, K., Narang, S.,
Matena, M., Zhou, Y., Li, W., and Liu, P. J. (2020).
Exploring the limits of transfer learning with a unified
text-to-text transformer. Journal of Machine Learning
Research, 21:1–67.
Rossanez, A. and dos Reis, J. C. (2019). Generating knowl-
edge graphs from scientific literature of degenerative
diseases. In SEPDA@ ISWC, pages 12–23.
Rossanez, A., Reis, J., and Torres, R. D. S. (2020). Rep-
resenting scientific literature evolution via temporal
knowledge graphs. CEUR Workshop Proceedings.
Shaikh, S., Rathi, S., and Janrao, P. (2017). Recom-
mendation system in e-commerce websites: A graph
based approached. In 2017 IEEE 7th International
Advance Computing Conference (IACC), pages 931–
934. IEEE.
Sharma, R. K. and Joshi, M. (2020). An analytical study
and review of open source chatbot framework, rasa.
International Journal of Engineering Research, 9.
Shi, B. and Weninger, T. (2018). Open-world knowledge
graph completion. In Proceedings of the AAAI Con-
ference on Artificial Intelligence, volume 32.
Vaswani, A., Shazeer, N., Parmar, N., Uszkoreit, J., Jones,
L., Gomez, A. N., Kaiser, Ł., and Polosukhin, I.
(2017). Attention is all you need. Advances in neural
information processing systems, 30.
Vegesna, A., Jain, P., and Porwal, D. (2018). Ontology
based chatbot (for e-commerce website). Interna-
tional Journal of Computer Applications, 179(14):51–
55.
Wong, W., Bartels, M., and Chrobot, N. (2014). Practical
eye tracking of the ecommerce website user experi-
ence. In International Conference on Universal Ac-
cess in Human-Computer Interaction, pages 109–118.
Springer.
Xue, L., Constant, N., Roberts, A., Kale, M., Al-Rfou, R.,
Siddhant, A., Barua, A., and Raffel, C. (2021). mt5: A
massively multilingual pre-trained text-to-text trans-
former. In Proceedings of the 2021 Conference of the
North American Chapter of the Association for Com-
putational Linguistics: Human Language Technolo-
gies, pages 483–498.
Zhang, J., Zhao, Y., Saleh, M., and Liu, P. (2020). Pegasus:
Pre-training with extracted gap-sentences for abstrac-
tive summarization. In International Conference on
Machine Learning, pages 11328–11339. PMLR.
QART: A Framework to Transform Natural Language Questions and Answers into RDF Triples
65