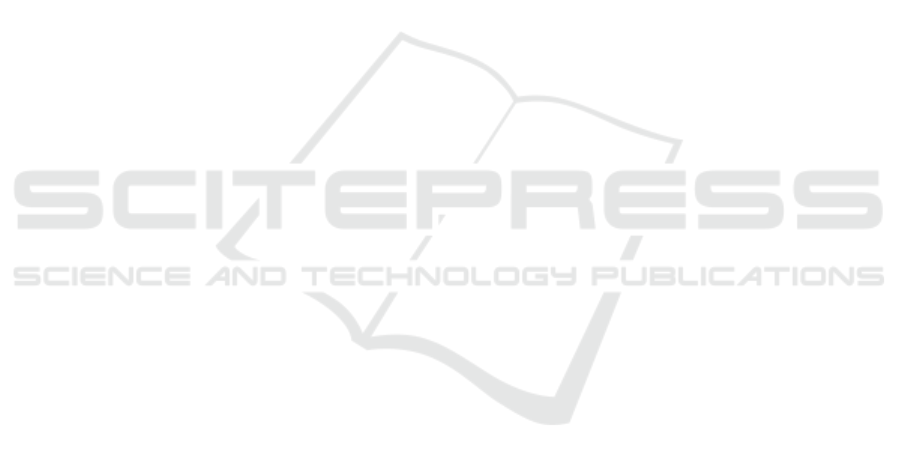
cial intelligence (XAI) research both in terms of con-
ceptual formalism and evaluation metrics and propose
some XAI methods that could be incorporated into
specific state-of-the-art EDM approaches.
In the long horizon, achieving explainable EDM
would have a great impact on education and should
be among significant goals for a healthier and trust-
worthy EDM practice. Our work is a foundational
research and does not lead to any direct applications.
ACKNOWLEDGEMENTS
This work is part of the Digital Mentoring project,
which is funded by the Stiftung Innovation in der
Hochschullehre under FBM2020-VA-219-2-05750.
REFERENCES
Al-Shedivat, M., Dubey, A., and Xing, E. P. (2020). Con-
textual explanation networks. J. Mach. Learn. Res.,
21:194–1.
Alvarez Melis, D. and Jaakkola, T. (2018). Towards robust
interpretability with self-explaining neural networks.
Advances in neural information processing systems,
31.
Asif, R., Merceron, A., Ali, S. A., and Haider, N. G. (2017).
Analyzing undergraduate students’ performance using
educational data mining. Computers & Education,
113:177–194.
Bhopal, K. and Myers, M. (2020). The impact of covid-19
on a level students in england.
Burgos, C., Campanario, M. L., de la Pe
˜
na, D., Lara, J. A.,
Lizcano, D., and Mart
´
ınez, M. A. (2018). Data min-
ing for modeling students’ performance: A tutoring
action plan to prevent academic dropout. Computers
& Electrical Engineering, 66:541–556.
Burkart, N. and Huber, M. F. (2021). A survey on the ex-
plainability of supervised machine learning. Journal
of Artificial Intelligence Research, 70:245–317.
Calvet Li
˜
n
´
an, L. and Juan P
´
erez,
´
A. A. (2015). Educa-
tional data mining and learning analytics: differences,
similarities, and time evolution. International Jour-
nal of Educational Technology in Higher Education,
12(3):98–112.
Cruz-Jesus, F., Castelli, M., Oliveira, T., Mendes, R.,
Nunes, C., Sa-Velho, M., and Rosa-Louro, A. (2020).
Using artificial intelligence methods to assess aca-
demic achievement in public high schools of a euro-
pean union country. Heliyon, 6(6):e04081.
Fernandes, E., Holanda, M., Victorino, M., Borges, V., Car-
valho, R., and Van Erven, G. (2019). Educational data
mining: Predictive analysis of academic performance
of public school students in the capital of brazil. Jour-
nal of Business Research, 94:335–343.
Gardner, J., Brooks, C., and Baker, R. (2019). Evaluating
the fairness of predictive student models through slic-
ing analysis. In Proceedings of the 9th international
conference on learning analytics & knowledge, pages
225–234.
Gaur, M., Faldu, K., and Sheth, A. (2020). Semantics of
the black-box: Can knowledge graphs help make deep
learning systems more interpretable and explainable?
Hasib, K. M., Rahman, F., Hasnat, R., and Alam, M. G. R.
(2022). A machine learning and explainable ai ap-
proach for predicting secondary school student per-
formance. In 2022 IEEE 12th Annual Computing and
Communication Workshop and Conference (CCWC),
pages 0399–0405.
Hoffait, A.-S. and Schyns, M. (2017). Early detection of
university students with potential difficulties. Deci-
sion Support Systems, 101:1–11.
Hutt, S., Gardner, M., Duckworth, A. L., and D’Mello,
S. K. (2019). Evaluating fairness and generalizability
in models predicting on-time graduation from college
applications. International Educational Data Mining
Society.
Islam, S. R., Eberle, W., and Ghafoor, S. K. (2019).
Towards quantification of explainability in explain-
able artificial intelligence methods. arXiv preprint
arXiv:1911.10104.
Kaur, P., Singh, M., and Josan, G. S. (2015). Classification
and prediction based data mining algorithms to predict
slow learners in education sector. Procedia Computer
Science, 57:500–508. 3rd International Conference on
Recent Trends in Computing 2015 (ICRTC-2015).
Kim, B., Wattenberg, M., Gilmer, J., Cai, C., Wexler, J.,
Viegas, F., et al. (2018). Interpretability beyond fea-
ture attribution: Quantitative testing with concept ac-
tivation vectors (tcav). In International conference on
machine learning, pages 2668–2677. PMLR.
Lee, G.-H., Jin, W., Alvarez-Melis, D., and Jaakkola, T.
(2019). Functional transparency for structured data: a
game-theoretic approach. In International Conference
on Machine Learning, pages 3723–3733. PMLR.
Lombrozo, T. (2006). The structure and function of ex-
planations. Trends in cognitive sciences, 10(10):464–
470.
Lundberg, S. M. and Lee, S.-I. (2017). A unified approach
to interpreting model predictions. Advances in neural
information processing systems, 30.
Marcinkowski, F., Kieslich, K., Starke, C., and L
¨
unich, M.
(2020). Implications of ai (un-) fairness in higher ed-
ucation admissions: the effects of perceived ai (un-)
fairness on exit, voice and organizational reputation.
In Proceedings of the 2020 conference on fairness, ac-
countability, and transparency, pages 122–130.
Miller, T. (2019). Explanation in artificial intelligence: In-
sights from the social sciences. Artificial intelligence,
267:1–38.
Plumb, G., Al-Shedivat, M., Cabrera, A. A., Perer, A.,
Xing, E., and Talwalkar, A. (2019). Regularizing
black-box models for improved interpretability. arXiv
preprint arXiv:1902.06787.
Towards Explainability in Modern Educational Data Mining: A Survey
219