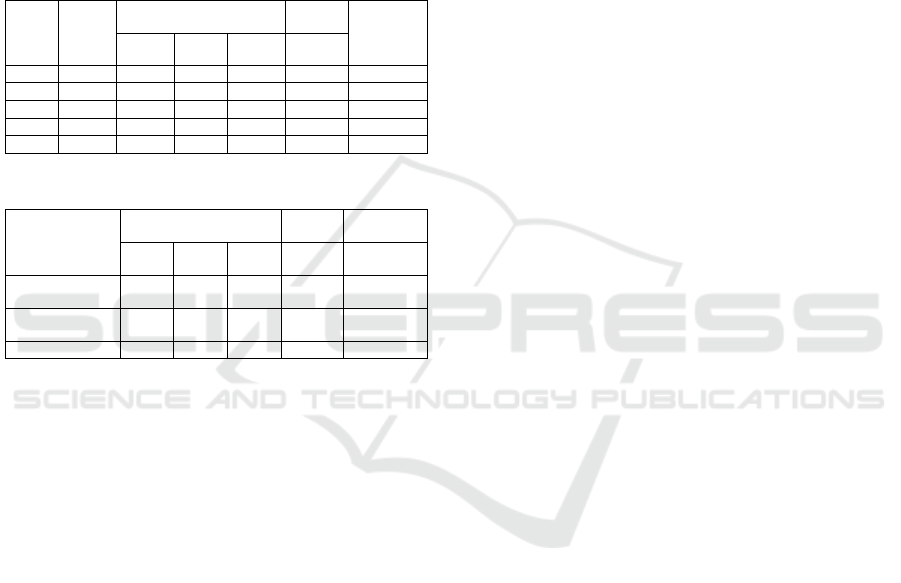
2 uses the semantic similarity method only, Scenario
3 uses a hybrid of syntactic and semantic similar-
ities methods with equal contribution (both have a
weight of 0.5).We present the evaluation of the pro-
posed similarity measure compared with the other
measure. Similarity measures are computed as shown
in 1. When we combine cosine and semantic similar-
ity, we obtain optimal results and it is more accurate
than a single measurement. We evaluate recommen-
dation accuracy by using Precision as shown in table
2.
Table 1: Similarity measurement results.
Syntactic Similarity Semantic
Similarity
Hybrid Simi-
larity
Profile
ID
Job ID Pearson Cosine Jaccard Spacy
NLP
Cosine and
Spacy NLP
1 1 0.34 0.44 0.41 0.51 0.47
2 2 0.45 0.38 0.21 0.32 0.35
3 3 0.74 0.92 0.67 0.99 0.9
4 4 0.56 0.58 0.45 0.62 0.6
5 5 0.33 0.41 0.12 0.38 0.35
Table 2: Evaluation recommendation.
Syntactic similarity Semantic
similarity
Hybrid simi-
larity
Jaccard Pearson Cosine Spacy
NLP
Cosine &
Spacy NLP
Total of recommenda-
tions
180 166 208 195 248
Pertinent recommen-
dations
77 68 92 84 139
Precision Value 0.42 0.4 0.44 0.43 0.56
6 CONCLUSION AND
PERSPECTIVES
“eRReBIS” is data-driven strategic decision system
and differs from the existing approach in that it re-
lies mainly on data management based BI architec-
ture. We propose Dynamic Multiviews dashboard-
ing (strategic, tactical, operational) based on prescrip-
tive analysis in order to assist the recruiter to iden-
tify trends, to create new business opportunities. Our
prototype is a content-based recommendation engines
that deploy a combination of similarity measures in
order to classify the candidates. We show that by
combining the similarity measures between the jobs
and skills, our model provides better recommenda-
tion for both recruiter and candidate. Additionally,
we also show some case studies which validate our
claims. Our system is scalable and can be gradually
enhanced by other massive data sources from social
networks and Business platforms. Furthermore, the
system architecture can be improved by other intel-
ligent features and components of deep learning al-
lowing the inference of strategic recommendations.
Future research will focus more on deep and predic-
tion analysis with machine learning techniques to im-
prove the performance of recommenders and linked
data profiling in the knowledge representation.
REFERENCES
Al-Otaibi, S. T. and Ykhlef, M. (2012). A survey of job rec-
ommender systems. International Journal of Physical
Sciences, 7(29):5127–5142.
Casagrande, A., Gotti, F., and Lapalme, G. (2017). Cerebra,
un syst
`
eme de recommandation de candidats pour l’e-
recrutement. In AISR2017.
F
¨
arber, F., Weitzel, T., and Keim, T. (2003). An automated
recommendation approach to selection in personnel
recruitment.
Jiechieu, K. F. F. and Tsopze, N. (2021). Skills prediction
based on multi-label resume classification using CNN
with model predictions explanation. Neural Comput-
ing and Applications, 33(10):5069–5087. Publisher:
Springer.
Karimi, M., Jannach, D., and Jugovac, M. (2018). News
recommender systems–Survey and roads ahead. Infor-
mation Processing & Management, 54(6):1203–1227.
Publisher: Elsevier.
Lang, S., Laumer, S., Maier, C., and Eckhardt, A. (2011).
Drivers, challenges and consequences of e-recruiting:
a literature review. In Proceedings of the 49th SIGMIS
annual conference on Computer personnel research,
pages 26–35.
Paquette, G. (2014). Technology-based instructional de-
sign: Evolution and major trends. Handbook of re-
search on educational communications and technol-
ogy, pages 661–671. Publisher: Springer.
Ramanath, R., Inan, H., Polatkan, G., Hu, B., Guo, Q.,
Ozcaglar, C., Wu, X., Kenthapadi, K., and Geyik,
S. C. (2018). Towards deep and representation learn-
ing for talent search at LinkedIn. In Proceedings of
the 27th ACM International Conference on Informa-
tion and Knowledge Management, pages 2253–2261.
Stohr, E. A. and Viswanathan, S. (1998). Recommendation
systems: Decision support for the information econ-
omy.
Wang, J., Zhang, Y., Posse, C., and Bhasin, A. (2013). Is it
time for a career switch? In Proceedings of the 22nd
international conference on World Wide Web, pages
1377–1388.
Xu, H., Yu, Z., Yang, J., Xiong, H., and Zhu, H. (2016).
Talent circle detection in job transition networks. In
Proceedings of the 22nd ACM SIGKDD International
Conference on Knowledge Discovery and Data Min-
ing, pages 655–664.
Zhao, M., Javed, F., Jacob, F., and McNair, M. (2015). Skill:
A system for skill identification and normalization. In
Twenty-Seventh IAAI Conference.
WEBIST 2022 - 18th International Conference on Web Information Systems and Technologies
380