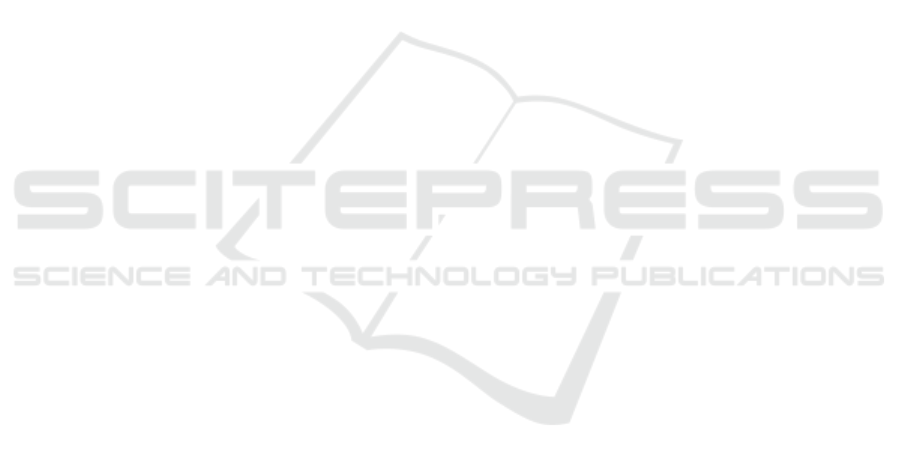
to automatically detect and address correlated events
during its building time, similarly to the concept of
predictive bi-clustering trees used in multi-label clas-
sification (Zamith et al., 2020). Finally, we would
also like to further validate our method by performing
more experiments, specially regarding datasets with
competing risks, as seen in (Ishwaran et al., 2014).
AUTHORS CONTRIBUTIONS
Conceptualization, M.V.; methodology, M.V. and
F.K.N.; software, M.V.; writing—original draft prepa-
ration, M.V. and F.K.N.; writing—review and editing,
C.V.; supervision, funding acquisition, C.V. All au-
thors have read and agreed to the published version of
the manuscript
FUNDING
This research was funded by the Research Fund
Flanders (through research project G080118N and
G0A2120N). The authors also acknowledge the
Flemish Government (AI Research Program).
REFERENCES
Dong, G., Mao, L., Huang, B., Gamalo-Siebers, M.,
Wang, J., Yu, G., and Hoaglin, D. C. (2020). The
inverse-probability-of-censoring weighting (ipcw) ad-
justed win ratio statistic: an unbiased estimator in the
presence of independent censoring. Journal of bio-
pharmaceutical statistics, 30(5):882–899.
Harrell, F., Califf, R., Pryor, D., Lee, K., and Rosati, R.
(1982). Evaluating the yield of medical tests. JAMA,
247(18):2543—2546.
Ishwaran, H., Gerds, T. A., Kogalur, U. B., Moore, R. D.,
Gange, S. J., and Lau, B. M. (2014). Random survival
forests for competing risks. Biostatistics, 15(4):757–
773.
Ishwaran, H., Kogalur, U. B., Blackstone, E. H., and Lauer,
M. S. (2008). Random survival forests. The annals of
applied statistics, 2(3):841–860.
Johnson, A. E., Pollard, T. J., Shen, L., Lehman, L.-w. H.,
Feng, M., Ghassemi, M., Moody, B., Szolovits, P.,
Anthony Celi, L., and Mark, R. G. (2016). Mimic-
iii, a freely accessible critical care database. Scientific
Data, 3(1):160035.
Kaplan, E. L. and Meier, P. (1958). Nonparametric esti-
mation from incomplete observations. Journal of the
American Statistical Association, 53(282):457–481.
Kocev, D., Vens, C., Struyf, J., and D
ˇ
zeroski, S. (2013).
Tree ensembles for predicting structured outputs. Pat-
tern Recognition, 46(3):817–833.
Ping Wang, Yan Li, C. K. R. (2019). Machine Learning for
Survival Analysis: A Survey. ACM Comput. Surv.,
51(6).
Tjandra, D., He, Y., and Wiens, J. (2021). A hierarchical
approach to multi-event survival analysis. Proceed-
ings of the AAAI Conference on Artificial Intelligence,
35(1):591–599.
Vock, D. M., Wolfson, J., Bandyopadhyay, S., Adomavi-
cius, G., Johnson, P. E., Vazquez-Benitez, G., and
O’Connor, P. J. (2016). Adapting machine learn-
ing techniques to censored time-to-event health record
data: A general-purpose approach using inverse prob-
ability of censoring weighting. J Biomed Inform,
61:119–131.
Xu, D., Shi, Y., Tsang, I. W., Ong, Y.-S., Gong, C., and
Shen, X. (2020). Survey on multi-output learning.
IEEE Transactions on Neural Networks and Learning
Systems, 31(7):2409–2429.
Zamith, B., Nakano, F. K., Cerri, R., and Vens, C. (2020).
Predictive bi-clustering trees for hierarchical multi-
label classification. ECML PKDD 2020.
SDAIH 2022 - Scarce Data in Artificial Intelligence for Healthcare
34