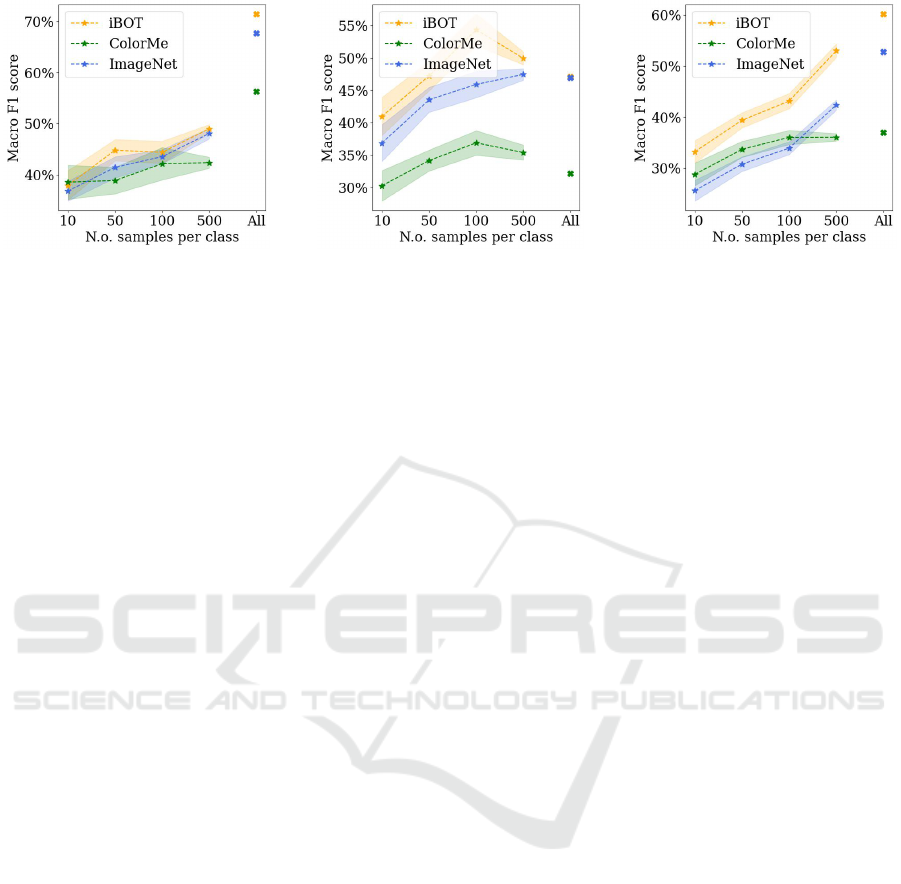
(a) Fitzpatrick17k (b) PAD-UFES-20 (c) HAM10000
Figure 1: Results of a kNN classifier on pre-trained representations when varying the number of samples per class for all three
downstream tasks.
respect to other methods. This suggests that the inher-
ent structure of the dataset labels was already learned
in the pre-training phase, and only minor adaptions to
the existing features could be done.
Finally, figure 1 shows the results of adding a kNN
classifier to the pre-trained ColorMe, iBOT and Im-
ageNet features upon changing the training dataset
size. The results achieved by iBOT outperform, on
average, the ones from ImageNet over all three down-
stream tasks, indicating that its features are very com-
petitive also in low data regimes.
5 CONCLUSION
In this paper, we set out to investigate whether
features from domain-specific self-supervised pre-
training yield a benefit over general-purpose ones
such as ImageNet weights, which are currently the
de facto standard in the medical domain. The re-
sults achieved so far indicate that there might be an
advantage in SSL initialization, especially when us-
ing iBOT. However, we currently cannot conclude
whether this benefit can be traced back to the pre-
training strategy or the difference in model architec-
ture. An indication in favor of the former is that
DINO, which is also based on ViTs, did not outper-
form ImageNet initialization. In the future, we plan
ablation experiments to determine if the performance
gain is really due to the pre-training task or influenced
by the different architecture.
REFERENCES
Brinker, T. J., Hekler, A., Enk, A. H., Berking, C., Hafer-
kamp, S., Hauschild, A., Weichenthal, M., Klode,
J., Schadendorf, D., Holland-Letz, T., von Kalle, C.,
Fr
¨
ohling, S., Schilling, B., and Utikal, J. S. (2019).
Deep neural networks are superior to dermatologists
in melanoma image classification. European Journal
of Cancer, 119:11–17.
Caron, M., Touvron, H., Misra, I., J
´
egou, H., Mairal, J., Bo-
janowski, P., and Joulin, A. (2021). Emerging Prop-
erties in Self-Supervised Vision Transformers. pages
9650–9660.
Chen, T., Kornblith, S., Norouzi, M., and Hinton, G. (2020).
A Simple Framework for Contrastive Learning of Vi-
sual Representations. In Proceedings of the 37th In-
ternational Conference on Machine Learning, pages
1597–1607. PMLR.
Deng, J., Dong, W., Socher, R., Li, L.-J., Li, K., and Fei-
Fei, L. (2009). Imagenet: A large-scale hierarchical
image database. In 2009 IEEE Conference on Com-
puter Vision and Pattern Recognition, pages 248–255.
Dosovitskiy, A., Beyer, L., Kolesnikov, A., Weissenborn,
D., Zhai, X., Unterthiner, T., Dehghani, M., Minderer,
M., Heigold, G., Gelly, S., Uszkoreit, J., and Houlsby,
N. (2020). An Image is Worth 16x16 Words: Trans-
formers for Image Recognition at Scale.
Giotis, I., Molders, N., Land, S., Biehl, M., Jonkman,
M., and Petkov, N. Med-node: A computer-
assisted melanoma diagnosis system using non-
dermoscopic images”. Expert Systems with Applica-
tions, 42:6578–6585.
Grill, J.-B., Strub, F., Altch
´
e, F., Tallec, C., Richemond, P.,
Buchatskaya, E., Doersch, C., Avila Pires, B., Guo,
Z., Gheshlaghi Azar, M., Piot, B., kavukcuoglu, k.,
Munos, R., and Valko, M. (2020). Bootstrap Your
Own Latent - A New Approach to Self-Supervised
Learning. In Advances in Neural Information Process-
ing Systems, volume 33, pages 21271–21284. Curran
Associates, Inc.
Groh, M., Harris, C., Soenksen, L., Lau, F., Han, R., Kim,
A., Koochek, A., and Badri, O. (2021). Evaluating
Deep Neural Networks Trained on Clinical Images in
Dermatology with the Fitzpatrick 17k Dataset. pages
1820–1828. IEEE Computer Society.
ISIC (2016). ISIC Archive. https://www.isic-archive.com/.
Accessed: 2022-05-20.
Jacob, J., Ciccarelli, O., Barkhof, F., and Alexander, D. C.
(2021). Disentangling human error from the ground
truth in segmentation of medical images. ACL.
Towards Reducing the Need for Annotations in Digital Dermatology with Self-supervised Learning
45