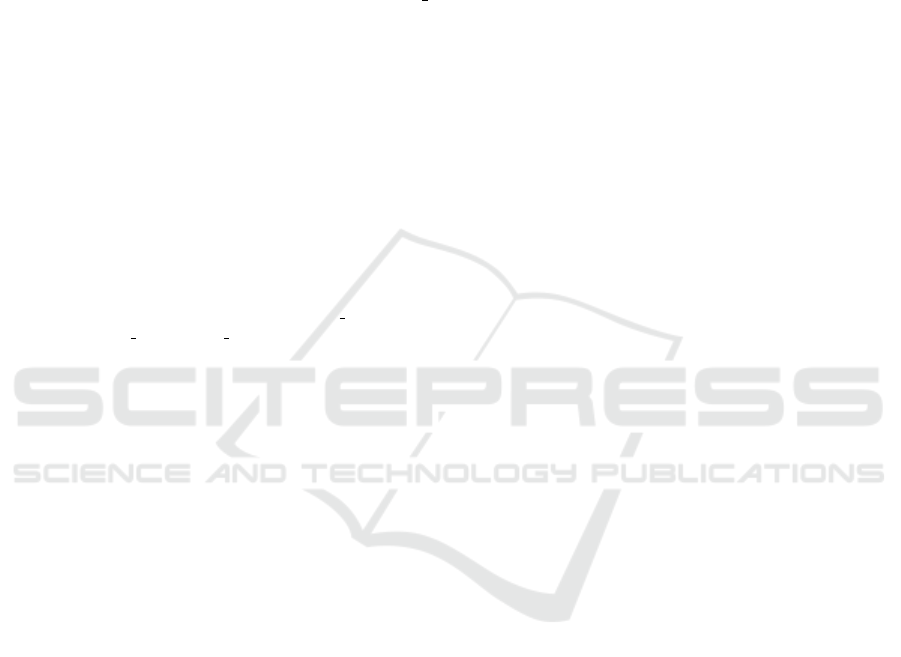
Balaniuk, R., Bessiere, P., Mazer, E., and Cobbe, P. (2012).
Risk based Government Audit Planning using Na
¨
ıve
Bayes Classifiers. In Advances in Knowledge-Based
and Intelligent Information and Engineering Systems,
Spain.
Balaprakash, P., Dongarra, J., Gamblin, T., Hall, M.,
Hollingsworth, J. K., Norris, B., and Vuduc, R.
(2018). Autotuning in high-performance computing
applications. Proceedings of the IEEE, 106(11):2068–
2083.
Brasil (1993). Lei 8666. http://www.planalto.gov.br/ccivil
03/leis/l8666cons.htm, visited 2020-05-16.
BRASIL (2020). Di
´
ario oficial da uni
˜
ao. http://www.in.gov.
br, visited 2020-05-16.
Carvalho, R. S. and Carvalho, R. N. (2016). Bayesian mod-
els to assess risk of corruption of federal management
units. In BMA@ UAI, pages 28–35.
Chung, M. T., Quang-Hung, N., Nguyen, M., and Thoai, N.
(2016). Using docker in high performance computing
applications. In 2016 IEEE Sixth International Con-
ference on Communications and Electronics (ICCE),
pages 52–57.
Connolly, S. and Matarazzo, J. (1998). Knowledge and Spe-
cial Libraries. Routledge.
de Bessa Lins, M. I. (1976). Di
´
arios oficiais dos estados
brasileiros. https://www.brapci.inf.br/ repositorio/
2010/02/pdf d7a5189ebe 0008270.pdf, visited 2020-
05-16.
de Sousa, S. R. (2014). Di
´
ario oficial do estado de
goi
´
as: uma proposta de requisitos de arquitetura da
informac¸
˜
ao para representac¸
˜
ao da informac¸
˜
ao oficial
digital.
Freund, G. E. (1982). Impactos da tecnologia da
informaC¸
˜
Ao.
Geist, A. and Reed, D. A. (2017). A survey of high-
performance computing scaling challenges. The In-
ternational Journal of High Performance Computing
Applications, 31(1):104–113.
Goel, S. and Uzuner, O. (2016). Do sentiments matter in
fraud detection? estimating semantic orientation of
annual reports. Intelligent Systems in Accounting, Fi-
nance and Management, 23(3):215–239.
Instituto Brasileiro de Geografia e Estat
´
ıstica
(2020). Estimativas da populac¸
˜
ao. https:
//www.ibge.gov.br/estatisticas/sociais/populacao/
9103-estimativas-de-populacao.html?=&t=
resultados, visited 2020-05-16.
Lima, M., Silva, R., Lopes de Souza Mendes, F., R. de
Carvalho, L., Araujo, A., and de Barros Vidal, F.
(2020). Inferring about fraudulent collusion risk on
Brazilian public works contracts in official texts us-
ing a Bi-LSTM approach. In Findings of the Associ-
ation for Computational Linguistics: EMNLP 2020,
pages 1580–1588, Online. Association for Computa-
tional Linguistics.
Luz de Araujo, P. H., de Campos, T. E., and Magalh
˜
aes
Silva de Sousa, M. (2020). Inferring the source of
official texts: Can svm beat ulmfit? In Quaresma,
P., Vieira, R., Alu
´
ısio, S., Moniz, H., Batista, F.,
and Gonc¸alves, T., editors, Computational Process-
ing of the Portuguese Language, pages 76–86, Cham.
Springer International Publishing.
Martano, A. M. R. (2015). Di
´
ario livre: co-criac¸
˜
ao
de uma ferramentapara publicac¸
˜
ao de um di
´
ario
oficial em formato aberto. Master’s thesis,
Escola de Artes, Ci
ˆ
encias e Humanidades.
https://teses.usp.br/teses/disponiveis/100/100131/
tde-21122015-091757/publico/dissertacao.pdf,
visited 2020-05-16.
Popowich, F. (2005). Using text mining and natural lan-
guage processing for health care claims processing.
ACM SIGKDD Explorations Newsletter, 7(1):59–66.
Rabuzin, K. and Modru
ˇ
san, N. (2019). Prediction of public
procurement corruption indices using machine learn-
ing methods.
Ralha, C. G. and Silva, C. V. S. (2012). A multi-agent data
mining system for cartel detection in brazilian govern-
ment procurement. Expert Systems with Applications,
39(14):11642 – 11656.
Rocha, J. P. L. (2011). Intelig
ˆ
encia de fontes abertas: um es-
tudo sobre descoberta de conhecimento no di
´
ario ofi-
cial da uni
˜
ao. https://bdtd.ucb.br:8443/jspui/handle/
123456789/1336, visited 2020-05-16.
Seemakurthi, P., Zhang, S., and Qi, Y. (2015). Detection
of fraudulent financial reports with machine learning
techniques. In 2015 Systems and Information Engi-
neering Design Symposium, pages 358–361. IEEE.
Signor, R., Love, P. E., Belarmino, A. T., and Al-
fred Olatunji, O. (2020a). Detection of collusive ten-
ders in infrastructure projects: Learning from opera-
tion car wash. Journal of Construction Engineering
and Management, 146(1):05019015.
Signor, R., Love, P. E., Oliveira Jr, A., Lopes, A. O.,
and Oliveira Jr, P. S. (2020b). Public infrastruc-
ture procurement: Detecting collusion in capped first-
priced auctions. Journal of Infrastructure Systems,
26(2):05020002.
Sun, T. and Sales, L. J. (2018). Predicting Public Pro-
curement Irregularity: An Application of Neural Net-
works. Journal of Emerging Technologies in Account-
ing, 15(1):141–154.
Vallim, J. J. d. C. B. (2020). Uso do Modelo de Racioc
´
ınio
Baseado em Casos Para Monitoramento de Conluio
em Licitac¸
˜
oes de Obras de Pavimentac¸
˜
ao Urbana.
Master’s thesis, Universidade Federal do Paran
´
a, Cu-
ritiba.
Van Arkel, J. H., Wagner, J. J., Schweyen, C. L., Mahone,
S. M., Curtis, T. J., HAGINS, S., et al. (2013). Pre-
dictive modeling processes for healthcare fraud detec-
tion. US Patent App. 13/536,414.
Xavier, B. M., da Silva, A. D., and Gomes, G. R. R. (2015).
Uma arquitetura hibrida para a indexac¸
˜
ao de docu-
mentos do di
´
ario oficial do munic
´
ıpio de cachoeiro de
itapemirim.
Deep-vacuity: A Proposal of a Machine Learning Platform based on High-performance Computing Architecture for Insights on Government
of Brazil Official Gazettes
143