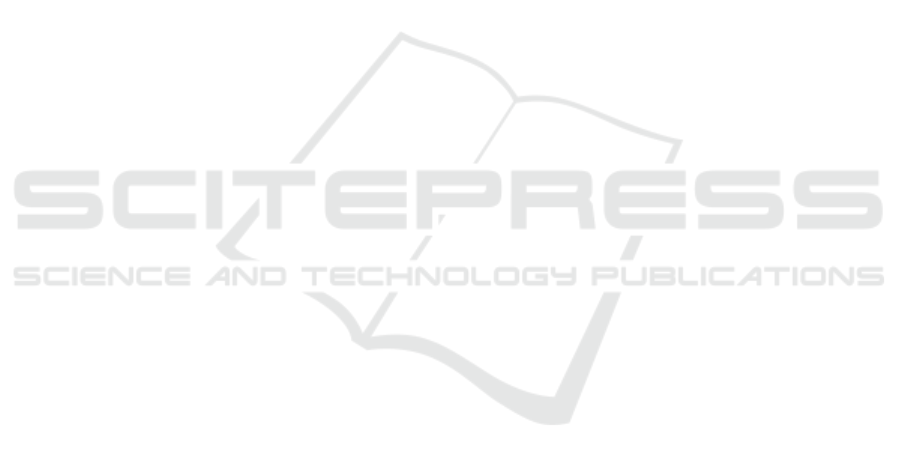
future date, thus indirectly predicting the community
structure in a significant subnetwork. This significant
subnetwork retains only the nodes with a strong com-
munity forming tendency over time, determined using
our novel entropy rate metric. Overall, our results in
the case study of the international refugee migration
network demonstrate that the effectiveness of our pro-
posed method depends strongly on the completeness
of the time series data.
There are limitations in our work stemming from
the data quality and availability, concerning the mi-
gration flow datasets. Finding datasets outside of mi-
gration flow is non-trivial, given the nuanced proper-
ties expected of the dataset, i.e., directed networks,
sparse, and with time series. Further research can be
pursued for migration flow data analysis itself in im-
proving the data quality using imputation and other
methods appropriate for the data.
ACKNOWLEDGEMENTS
The authors acknowledge the support of the IIIT Ban-
galore and the IIT Kharagpur summer internship pro-
gram for conducting this work. The authors are
grateful to the anonymous reviewers whose sugges-
tions have improved this paper. This publication
is supported by the grant by the Science and Engi-
neering Research Board (SERB), Government of In-
dia, under the Mathematical Research Impact Sup-
port (MATRICS). The authors are thankful to the help
provided by members of the Graphics-Visualization-
Computing Lab.
REFERENCES
Agreste, S., De Meo, P., Fiumara, G., and et al. (2016).
An empirical comparison of algorithms to find com-
munities in directed graphs and their application in
web data analytics. IEEE Transactions on Big Data,
3(3):289–306.
Bongiorno, C., Zino, L., and Rizzo, A. (2019). A novel
framework for community modeling and characteri-
zation in directed temporal networks. Appl. Net. Sc.,
4(1):1–25.
Box, G. and Jenkins, G. (1970). Time Series Analysis: Fore-
casting and Control. Holden-Day, San Francisco.
Cai, D., Campbell, T., and Broderick, T. (2016). Edge-
exchangeable graphs and sparsity. Advances in Neural
Info. Processing Sys., 29.
Cover, T. M. and Thomas, J. A. (2006). Elements of Infor-
mation Theory (Wiley Series in Telecommunications
and Signal Processing). Wiley-Intersc.
Fortunato, S. (2010). Community Detection in Graphs.
Phys. Reports, 486(3-5):75–174.
Krogstad, J. M. and Radford, J. (2017). Key facts about
refugees to the US. Pew Rsrch. Cntr., 30.
Krzakala, F., Moore, C., Mossel, E., Neeman, J., Sly, A.,
Zdeborov
´
a, L., and Zhang, P. (2013). Spectral re-
demption in clustering sparse networks. Proceedings
of the National Academy of Sciences, 110(52):20935–
20940.
Kvalseth, T. O. (1987). Entropy and correlation: Some
comments. IEEE Transactions on Systems, Man, and
Cybernetics, 17(3):517–519.
Leicht, E. A. and Newman, M. E. (2008). Community
structure in directed networks. Phys. Rev. Letters,
100(11):118703.
Malliaros, F. D. and Vazirgiannis, M. (2013). Clustering and
community detection in directed networks: A survey.
Phys. Reports, 533(4):95–142.
Martin, S., Mor
˘
arescu, I.-C., and Ne
ˇ
si
´
c, D. (2016). Time
scale modeling for consensus in sparse directed net-
works with time-varying topologies. In 2016 IEEE
55th Conference on Decision and Control (CDC),
pages 7–12. IEEE.
Mirshahvalad, A., Lindholm, J., Derlen, M., and Rosvall,
M. (2012). Significant communities in large sparse
networks. PloS one, 7(3):e33721.
Miyauchi, A. and Kawase, Y. (2016). Z-score-based modu-
larity for community detection in networks. PloS one,
11(1):e0147805.
Neumayer, E. (2005). Bogus refugees? The determinants
of asylum migration to Western Europe. International
studies quarterly, 49(3):389–409.
Nicosia, V., Mangioni, G., Carchiolo, V., and Malgeri,
M. (2009). Extending the definition of modular-
ity to directed graphs with overlapping communi-
ties. Jrnl. of Stat. Mech.: Theory and Experiment,
2009(03):P03024.
Pedregosa, F., Varoquaux, G., Gramfort, A., and et al.
(2011). Scikit-learn: Machine Learning in Python.
Journal of Machine Learning Research, 12:2825–
2830.
Pons, P. and Latapy, M. (2006). Computing communities in
large networks using random walks. Journal of Graph
Algorithms and Applications, 10(2):191–218.
Radicchi, F., Castellano, C., Cecconi, F., Loreto, V., and
Parisi, D. (2004). Defining and identifying communi-
ties in networks. PNAS, 101(9):2658–2663.
Rand, W. M. (1971). Objective criteria for the evaluation of
clustering methods. Journal of the American Statisti-
cal Association, 66(336):846–850.
Rossetti, G. and Cazabet, R. (2018). Community discov-
ery in dynamic networks: A survey. ACM Computing
Surveys (CSUR), 51(2):1–37.
Rossetti, G., Milli, L., and Cazabet, R. (2019). CDLIB:
A Python Library to Extract, Compare and Evaluate
Communities from Complex Networks. Applied Net-
work Science, 4(1):1–26.
Rosvall, M. and Bergstrom, C. T. (2008). Maps of random
walks on complex networks reveal community struc-
ture. PNAS, 105(4):1118–1123.
CAP-DSDN: Node Co-association Prediction in Communities in Dynamic Sparse Directed Networks and a Case Study of Migration Flow
73