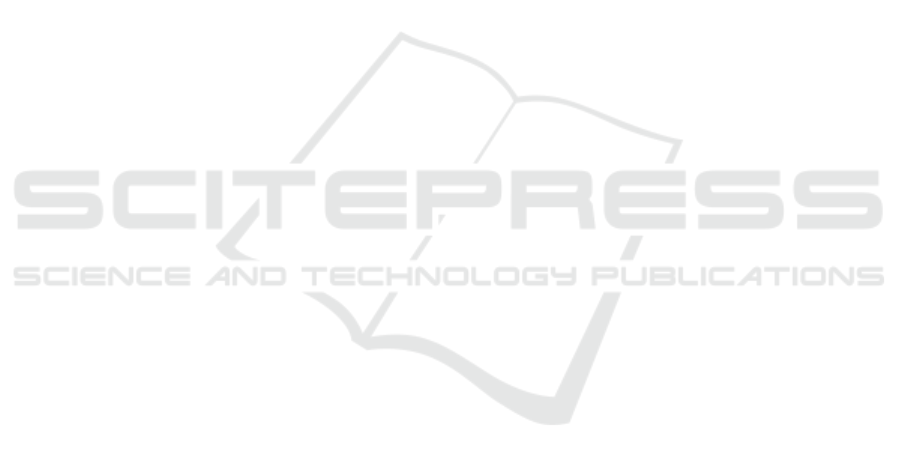
Dlova, N., Chateau, A., Khoza, N., Skenjane, A., Mkhize,
M., Katibi, O., Grobler, A., Tsoka-Gwegweni, J., and
Mosam, A. (2017). Prevalence of skin diseases treated
at public referral hospitals in KwaZulu-Natal, South
Africa. The British journal of dermatology, 178.
Genc, E. U., Ahuja, N., Ndiour, I. J., and Tickoo, O.
(2021). Energy-based anomaly detection and local-
ization. arXiv preprint arXiv:2105.03270.
Gonzalez-Jimenez, A., Lionetti, S., Amruthalingamnd, L.,
Gottfrois, P., Pouly, M., and Navarini, A. (2022). Jew-
elry segmentation masks for the 11k Hands dataset.
Groh, M., Harris, C., Soenksen, L., Lau, F., Han, R., Kim,
A., Koochek, A., and Badri, O. (2021). Evaluating
Deep Neural Networks Trained on Clinical Images in
Dermatology with the Fitzpatrick 17k Dataset.
Heer, M., Postels, J., Chen, X., Konukoglu, E., and Albar-
qouni, S. (2021). The OOD Blind Spot of Unsuper-
vised Anomaly Detection.
Ho, J., Jain, A., and Abbeel, P. (2020). Denoising Diffusion
Probabilistic Models.
Kamulegeya, L. H., Okello, M., Bwanika, J. M., Musin-
guzi, D., Lubega, W., Rusoke, D., Nassiwa, F., and
B
¨
orve, A. (2019). Using artificial intelligence on der-
matology conditions in Uganda: A case for diversity
in training data sets for machine learning.
Kingma, D. P. and Ba, J. (2017). Adam: A method for
stochastic optimization.
Kingma, D. P. and Welling, M. (2014). Auto-Encoding
Variational Bayes.
Kiprono, S. K., Muchunu, J. W., and Masenga, J. E. (2015).
Skin diseases in pediatric patients attending a tertiary
dermatology hospital in Northern Tanzania: A cross-
sectional study. BMC Dermatology, 15(1):16.
Liu, Q., Lee, J., and Jordan, M. (2016). A Kernelized Stein
Discrepancy for Goodness-of-fit Tests. In Proceed-
ings of The 33rd International Conference on Machine
Learning, pages 276–284. PMLR.
Liu, Y., Jain, A., Eng, C., Way, D. H., Lee, K., Bui,
P., Kanada, K., de Oliveira Marinho, G., Gallegos,
J., Gabriele, S., Gupta, V., Singh, N., Natarajan, V.,
Hofmann-Wellenhof, R., Corrado, G. S., Peng, L. H.,
Webster, D. R., Ai, D., Huang, S. J., Liu, Y., Dunn,
R. C., and Coz, D. (2020). A deep learning sys-
tem for differential diagnosis of skin diseases. Nature
Medicine, 26(6):900–908.
Razavi, A., van den Oord, A., and Vinyals, O. (2019). Gen-
erating Diverse High-Fidelity Images with VQ-VAE-
2.
Rezende, D. J., Mohamed, S., and Wierstra, D. (2014).
Stochastic backpropagation and approximate infer-
ence in deep generative models. In International
conference on machine learning, pages 1278–1286.
PMLR.
Ribeiro, V., Avila, S., and Valle, E. (2019). Handling Inter-
Annotator Agreement for Automated Skin Lesion Seg-
mentation.
Schlegl, T., Seeb
¨
ock, P., Waldstein, S., Langs, G., and
Schmidt-Erfurth, U. (2019). F-AnoGAN: Fast Unsu-
pervised Anomaly Detection withGenerative Adver-
sarial Networks. Medical Image Analysis, 54.
Song, Y., Durkan, C., Murray, I., and Ermon, S. (2021a).
Maximum Likelihood Training of Score-Based Diffu-
sion Models.
Song, Y., Sohl-Dickstein, J., Kingma, D. P., Kumar, A., Er-
mon, S., and Poole, B. (2021b). Score-Based Gener-
ative Modeling through Stochastic Differential Equa-
tions. In 9th International Conference on Learning
Representations,ICLR.
Stein, C. (1972). A bound for the error in the normal ap-
proximation to the distribution of a sum of dependent
random variables. In The Sixth Berkeley Symposium
on Mathematical Statistics and Probability, Volume 2:
Probability Theory, volume 6.2, pages 583–603. Uni-
versity of California Press.
Wolleb, J., Bieder, F., Sandk
¨
uhler, R., and Cattin, P. C.
(2022). Diffusion Models for Medical Anomaly De-
tection.
World Health Organization (2005). Epidemiology and man-
agement of common skin diseases in children in devel-
oping countries. (WHO/FCH/CAH/05.12).
Yi, J. and Yoon, S. (2020). Patch svdd: Patch-level svdd for
anomaly detection and segmentation. In Proceedings
of the Asian Conference on Computer Vision.
Zimmerer, D., Kohl, S. A. A., Petersen, J., Isensee, F., and
Maier-Hein, K. H. (2018). Context-encoding Varia-
tional Autoencoder for Unsupervised Anomaly Detec-
tion.
SDAIH 2022 - Scarce Data in Artificial Intelligence for Healthcare
58