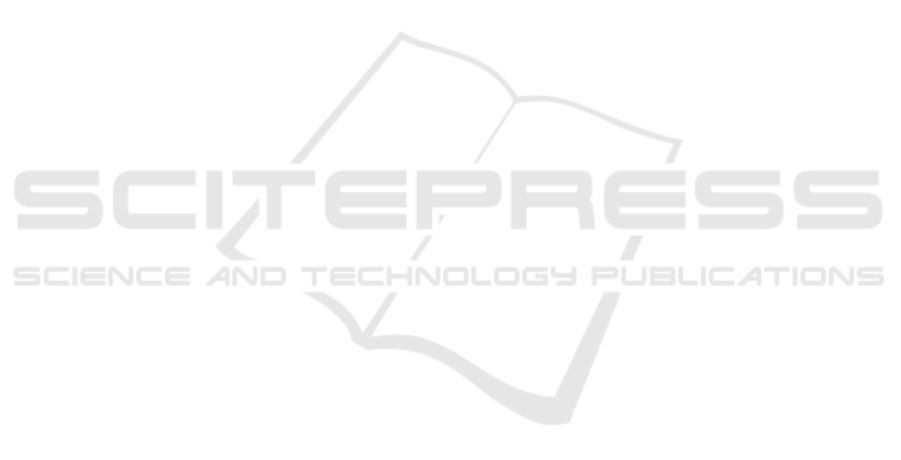
ered, it is shown that using (C2) coverage rates of over
95% can be achieved while the false positive rate is
significantly reduced compared to the FPR
RC
of the
role concept. These methods could therefore be used,
for example, by consultants when optimizing existing
role concepts and permission-to-user assignments.
8 CONCLUSION AND FUTURE
WORKS
In this paper, different methods were presented, which
aim at enhancing the assignment of permissions to
users based on trace data available in enterprise re-
source planning systems. These are based on the clus-
tering of users and the subsequent exchange of per-
missions within the users of the resulting clusters.
A special feature of the presented methods is that,
even though additional permissions are assigned to
users, the emergence of SoD conflicts can be avoided.
In order to be able to use the presented methods in
the framework of SAP ERP, the corresponding au-
thorization management data model was explained
in detail. Furthermore, trace and role concept data
derived from real-world use cases were analyzed as
basis for the evaluation of the presented methods.
The strengths and weaknesses of the various methods
could be shown and the potential of trace data for the
enhancement of permission-to-user assignment could
be demonstrated. In addition, it was shown that ex-
ploiting the knowledge about the relationship between
transactions and components or the pre-processing of
trace data, significantly improved the results of the
methods. Due to permission exchange, the structure
of the UPA is changed as users become more simi-
lar. It seems plausible that this facilitates the process
of finding good role concepts using EAs and needs to
be investigated in more detail in the future. Another
promising approach which could further improve the
quality of the presented methods could be the integra-
tion of user attributes into the clustering procedures.
REFERENCES
Anderer, S., Kreppein, D., Scheuermann, B., and
Mostaghim, S. (2020). The addRole-EA: A new
evolutionary algorithm for the role mining prob-
lem. In Proceedings of IJCCI 2020, pages 155–166.
SCITEPRESS.
Anderer, S., Scheuermann, B., Mostaghim, S., Bauerle, P.,
and Beil, M. (2021). RMPlib: A library of bench-
marks for the role mining problem. In Proceedings of
SACMAT ’21, page 3–13, New York, NY, USA. Asso-
ciation for Computing Machinery.
Anderer, S., Schrader, F., Scheuermann, B., and
Mostaghim, S. (2022). Evolutionary algorithms for
the constrained two-level role mining problem. In
Proceedings of EvoCOP 2022, page 79–94, Berlin,
Heidelberg. Springer-Verlag.
Du, X. and Chang, X. (2014). Performance of AI algo-
rithms for mining meaningful roles. In 2014 IEEE
Congress on Evolutionary Computation (CEC), pages
2070–2076. IEEE.
Eiben, A. E., Smith, J. E., et al. (2003). Introduction to
evolutionary computing, volume 53. Springer.
Frank, M., Basin, D., and Buhmann, J. M. (2008). A class
of probabilistic models for role engineering. In Pro-
ceedings of ACM CSS ’08, pages 299–310.
Frank, M., Buhmann, J. M., and Basin, D. (2010). On the
definition of role mining. In Proceedings of SACMAT
’10, page 35–44, New York, NY, USA. Association
for Computing Machinery.
Frank, M., Streich, A. P., Basin, D., and Buhmann, J. M.
(2009). A probabilistic approach to hybrid role min-
ing. In Proceedings of ACM CSS ’09, pages 101–111.
Fukunaga, K. and Hostetler, L. (1975). The estimation of
the gradient of a density function, with applications in
pattern recognition. IEEE Transactions on informa-
tion theory, 21(1):32–40.
Jaccard, P. (1901).
´
Etude comparative de la distribution flo-
rale dans une portion des alpes et des jura. Bull Soc
Vaudoise Sci Nat, 37:547–579.
Landau, S., Leese, M., Stahl, D., and Everitt, B. S. (2011).
Cluster analysis. John Wiley & Sons.
Lehnert, V., Stelzner, K., John, P., and Otto, A. (2016). SAP-
Berechtigungswesen: Konzeption und Realisierung.
Rheinwerk Publishing.
Lu, H., Vaidya, J., and Atluri, V. (2008). Optimal boolean
matrix decomposition: Application to role engineer-
ing. In 2008 IEEE 24th International Conference on
Data Engineering, pages 297–306. IEEE.
Mitra, B., Sural, S., Vaidya, J., and Atluri, V. (2016). A
survey of role mining. ACM Computing Surveys,
48(4):1–37.
Molloy, I., Li, N., Qi, Y., Lobo, J., and Dickens, L. (2010).
Mining roles with noisy data. In Proceedings of SAC-
MAT ’10, pages 45–54.
Roeckle, H., Schimpf, G., and Weidinger, R. (2000).
Process-oriented approach for role-finding to imple-
ment role-based security administration in a large in-
dustrial organization. In Proceedings of RBAC ’00,
pages 103–110, New York, USA. ACM Press.
Saenko, I. and Kotenko, I. (2011). Genetic algorithms
for role mining problem. In Proceedings of PDP’11,
pages 646–650. IEEE.
Vaidya, J., Atluri, V., and Guo, Q. (2007). The role mining
problem. In Proceedings of SACMAT ’07, pages 175–
184, New York, New York, USA. ACM Press.
Vaidya, J., Atluri, V., Guo, Q., and Lu, H. (2010). Role
mining in the presence of noise. In Proceedings of
DBSEC 2010, pages 97–112. Springer.
Verizon (2019). Data breach investigations report 2019.
Computer Fraud & Security, 2019(6):4.
ECTA 2022 - 14th International Conference on Evolutionary Computation Theory and Applications
132