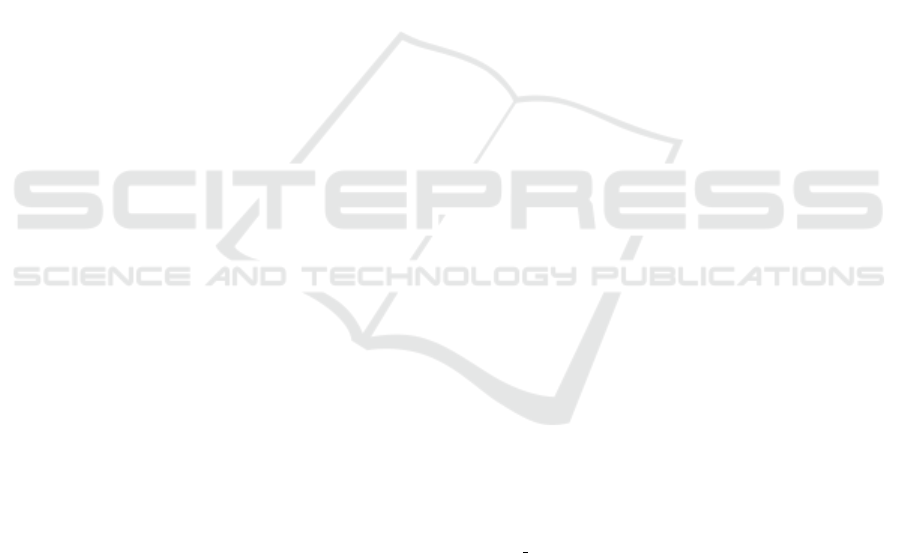
• Rarely discussed is the quality of the ground truth,
whether the set of skulls to train is cherry-picked
in some way, and how the Dice similarity coef-
ficient is exactly calculated (average or volume-
metric). While one paper calculates a statistical
error, systematic errors are never discussed. For
application use, it is much more important that
different groups with different methods all obtain
good enough results. That points to the necessary
maturity of the field. This is actually a problem
with a lot of medical research.
• Quality control will be necessary, by manual in-
spection or otherwise. This aspect has not been
considered yet.
• We did not encounter any problems in using skulls
with openings in training, probably because the
holes were never in the same location and there-
fore each hole was influencing only a small part
of the sample.
In this paper we tried to emphasize a few salient
points for transfer of academic research to industry.
First, how particular academic research can be used
in industry is not always very clear. Mesh manufac-
turing seemed originally more a problem in metal-
lurgy than a medical problem. Second, the transition
from papers in academic journals to reusing the work
elsewhere is more painful than academic researchers
seem to realize.
As a more general conclusion, we want to present
a more positive view by this example, given a general
pessimism that research is having diminishing returns
in boosting productivity, as for instance defended in
(Bloom et al., 2017). Bloom et. al. explicitly dismiss
a possible role of AI in growth of productivity. It is
true, as stated in that article, that most of IT efforts
have been spent on increasing choices (more choice
in streams instead of more time to listen, more fonts
instead of easier to understand documents, etc.), and
that AI has played a minor role in that. However,
the current example in this paper of technology trans-
fer provides a counter-example to that pessimism. It
shows that relatively low investments still can lead to
meaningful productivity improvements. AI can play
a significant role in that.
ACKNOWLEDGEMENTS
We thank our colleagues at Meticuly for their help and
contributions.
REFERENCES
Bloom, N., Jones, C., Van Reenen, J., and Webb, M. (2017).
Ideas aren’t running out, but they are getting more ex-
pensive to find. https://voxeu.org/article/ideas-aren-t-
running-out-theyare-getting-more-expensive-find.
Huttenlocher, P., Klanderman, G., and Rucklidge, W.
(1993). Comparing images using the Hausdorff dis-
tance. In IEEE Transactions on Pattern Analysis and
Machine Intelligence, volume 15(9), page 850. IEEE.
Jimenez, S., Gonzalez, F. A., and Gelbukh, A. (2016).
Mathematical properties of soft cardinality: Enhanc-
ing Jaccard, Dice and cosine similarity measures with
element-wise distance. Information Sciences, 367-
368:373–389.
Klein, A., Warszawski, J., Hillengaß, J., and Maier-Hein,
K. (2019). Automatic bone segmentation in whole-
body CT images. International journal of com-
puter assisted radiology and surgery, 14(1):21–29.
https://doi.org/10.1007/s11548-018-1883-7.
Kushner, D. S., Verano, J. W., and Titelbaum, A. R.
(2018). Trepanation procedures/outcomes:
Comparison of prehistoric Peru with other an-
cient, medieval, and American civil war cranial
surgery. World Neurochirurgy, 114:245–251.
doi:10.1016/j.wneu.2018.03.143.
Mader, K. (n.d.). 4Quant/Bone-Segmenter.
https://github.com/4Quant/Bone-Segmenter.
Mahinda, H. and Murty, O. (July-December 2009). Vari-
ability in thickness of human skull bones and sternum
– an autopsy experience. Journal of Forensic Medicine
& Toxicology, 26(2):26–31.
Minnema, J., van Eijnatten, M., Kouw, W., Diblen, F., Men-
drik, A., and Wolff, J. (2018). CT image segmentation
of bone for medical additive manufacturing using a
convolutional neural network. Computers in Biology
and Medicine, 103:130–139.
Rawat, W. and Wang, Z. (2017). Deep convolutional neural
networks for image classification: A comprehensive
review. Neural Computation, 29(9):2352–2449.
Ronneberger, O., Fischer, P., and Brox, T. (2015). U-
net:convolutional networks for biomedical image
segmentation. In Medical Image Computing and
Computer-Assisted Intervention – MICCAI 2015. Lec-
ture Notes in Computer Science, Volume 9351,
Springer. https://doi.org/10.1007/978-3-319-24574-
4
28.
Structseg2019 (2019). Structseg 2019 auto-
matic structure segmentation for radio ther-
apy challenge. https://structseg2019.grand-
challenge.org/Evaluation/.
van Eijnatten, M., van Dijk, R., Dobbe, J., Streekstra, G.,
Koivisto, J., and Wolff, J. (2018). CT image segmen-
tation methods for bone used in medical additive man-
ufacturing. Medical Engineering & Physics, 51:6–16.
Xia, D.-F., Xu, S.-L., and Qi, F. (1999). A proof of the arith-
metic mean – geometric mean – harmonic mean in-
equalities. RGMIA Research Report Collection, 2(1).
NCTA 2022 - 14th International Conference on Neural Computation Theory and Applications
380