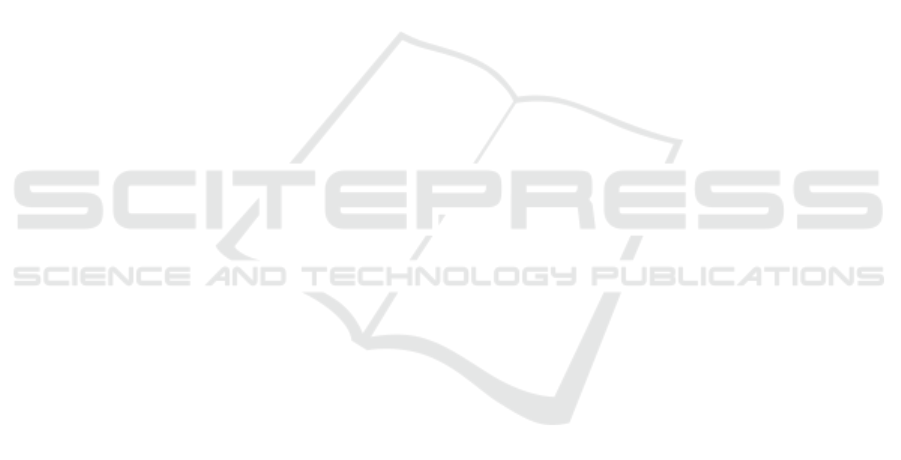
Battineni, G., Chintalapudi, N., and Amenta, F. (2020).
Forecasting of covid-19 epidemic size in four high
hitting nations (usa, brazil, india and russia) by fb-
prophet machine learning model. Applied Computing
and Informatics.
Carrara, F., Amato, G., Brombin, L., Falchi, F., and Gen-
naro, C. (2021). Combining gans and autoencoders
for efficient anomaly detection. In 2020 25th Inter-
national Conference on Pattern Recognition (ICPR),
pages 3939–3946. IEEE.
Curia, F. (2020). Unsupervised hybrid algorithm to detect
anomalies for predicting terrorists attacks. Interna-
tional Journal of Computer Applications, 975:8887.
Diab, S. (2019). Optimizing stochastic gradient de-
scent in text classification based on fine-tuning hyper-
parameters approach. a case study on automatic clas-
sification of global terrorist attacks. arXiv preprint
arXiv:1902.06542.
Jackson, R. (2018). Writing the war on terrorism: Lan-
guage, politics and counter-terrorism. Manchester
University Press.
Jha, B. K. and Pande, S. (2021). Time series forecasting
model for supermarket sales using fb-prophet. In 2021
5th International Conference on Computing Method-
ologies and Communication (ICCMC), pages 547–
554. IEEE.
Khorshid, M., Abou-El-Enien, T., and Soliman, G.
(2015a). A comparison among support vector ma-
chine and other machine learning classification algo-
rithms. IPASJ International Journal of Computer Sci-
ence (IIJCS).
Khorshid, M. M., Abou-El-Enien, T. H., and Soliman,
G. M. (2015b). Hybrid classification algorithms for
terrorism prediction in middle east and north africa.
International Journal of Emerging Trends & Technol-
ogy in Computer Science, 4(3):23–29.
Laite, R., Lozano, M., and Sankaranarayanan, K. (2019).
Terrorist group classification of historic terrorist at-
tacks from the global terrorism database. In 2019 14th
International Conference on Computer Science & Ed-
ucation (ICCSE), pages 237–242. IEEE.
Li, S., Zhuang, J., and Shen, S. (2017). Dynamic forecast-
ing conditional probability of bombing attacks based
on time-series and intervention analysis. Risk analy-
sis, 37(7):1287–1297.
Mathivanan, N. M. N., Ghani, N. A. M., and Janor, R. M.
(2018). Improving classification accuracy using clus-
tering technique. Bulletin of Electrical Engineering
and Informatics, 7(3):465–470.
Meng, X., Mo, H., Zhao, S., and Li, J. (2017). Application
of anomaly detection for detecting anomalous records
of terroris attacks. In 2017 IEEE 2nd International
Conference on Cloud Computing and Big Data Anal-
ysis (ICCCBDA), pages 70–75. IEEE.
Mohammed, D. Y. and Karabatak, M. (2018). Terrorist at-
tacks in turkey: An evaluate of terrorist acts that oc-
curred in 2016. In 2018 6th International Symposium
on Digital Forensic and Security (ISDFS), pages 1–3.
IEEE.
National Consortium for the Study of Terrorism and Re-
sponses to Terrorism (START) (2019). Global terror-
ism database. https://www.start.umd.edu/gtd.
Pag
´
an, J. V. (2010). Improving the classification of terrorist
attacks a study on data pre-processing for mining the
global terrorism database. In 2010 2nd International
Conference on Software Technology and Engineering,
volume 1, pages V1–104. IEEE.
Pan, X. (2021). Quantitative analysis and prediction of
global terrorist attacks based on machine learning.
Scientific Programming, 2021.
Pedregosa, F., Varoquaux, G., Gramfort, A., Michel, V.,
Thirion, B., Grisel, O., Blondel, M., Prettenhofer,
P., Weiss, R., Dubourg, V., Vanderplas, J., Passos,
A., Cournapeau, D., Brucher, M., Perrot, M., and
Duchesnay, E. (2011). Scikit-learn: Machine learning
in Python. Journal of Machine Learning Research,
12:2825–2830.
Peng, A. (2018). An integrated machine learning approach
to studying terrorism. In Undergraduate Thesis. Yale
University.
Pfeiffer, C. P. (2012). Causalities and casualties: Media
attention and terrorism, 1970–2010. Working Paper
127/2012, Helmut Schmidt University, Hamburg.
Rajan, S. (2021). Anomaly detection using autoen-
coders: A walk-through in python. https://tinyurl.
com/2p92wm4p. Analytics Vidhja. Accessed June
2022.
Sahin, Y. (2018). Forecasting the Monthly Occurrence of
Terrorist Incidents Based on the GPI indicators and
the GDP. PhD thesis, Tilburg University.
Singer, G. and Golan, M. (2019). Identification of sub-
groups of terror attacks with shared characteristics for
the purpose of preventing mass-casualty attacks: A
data-mining approach. Crime Science, 8(1):1–11.
Smith, T. G. et al. (2017). pmdarima: Arima estimators for
Python. http://www.alkaline-ml.com/pmdarima. Ac-
cessed June 2022.
Spinosa, E. J. and Carvalho, A. (2005). Support vector
machines for novel class detection in bioinformatics.
Genet Mol Res, 4(3):608–15.
Talreja, D., Nagaraj, J., Varsha, N., and Mahesh, K. (2017).
Terrorism analytics: Learning to predict the perpe-
trator. In 2017 International Conference on Ad-
vances in Computing, Communications and Informat-
ics (ICACCI), pages 1723–1726. IEEE.
Taylor, S. J. and Letham, B. (2017). Prophet: Forecast-
ing at scale. https://research.facebook.com/blog/2017/
02/prophet-forecasting-at-scale/. Meta Research. Ac-
cessed June 2022.
Tolan, G. M. and Soliman, O. S. (2015). An experi-
mental study of classification algorithms for terror-
ism prediction. International Journal of Knowledge
Engineering-IACSIT, 1(2):107–112.
Zenati, H., Foo, C. S., Lecouat, B., Manek, G., and Chan-
drasekhar, V. R. (2018). Efficient gan-based anomaly
detection. arXiv preprint arXiv:1802.06222.
KDIR 2022 - 14th International Conference on Knowledge Discovery and Information Retrieval
228