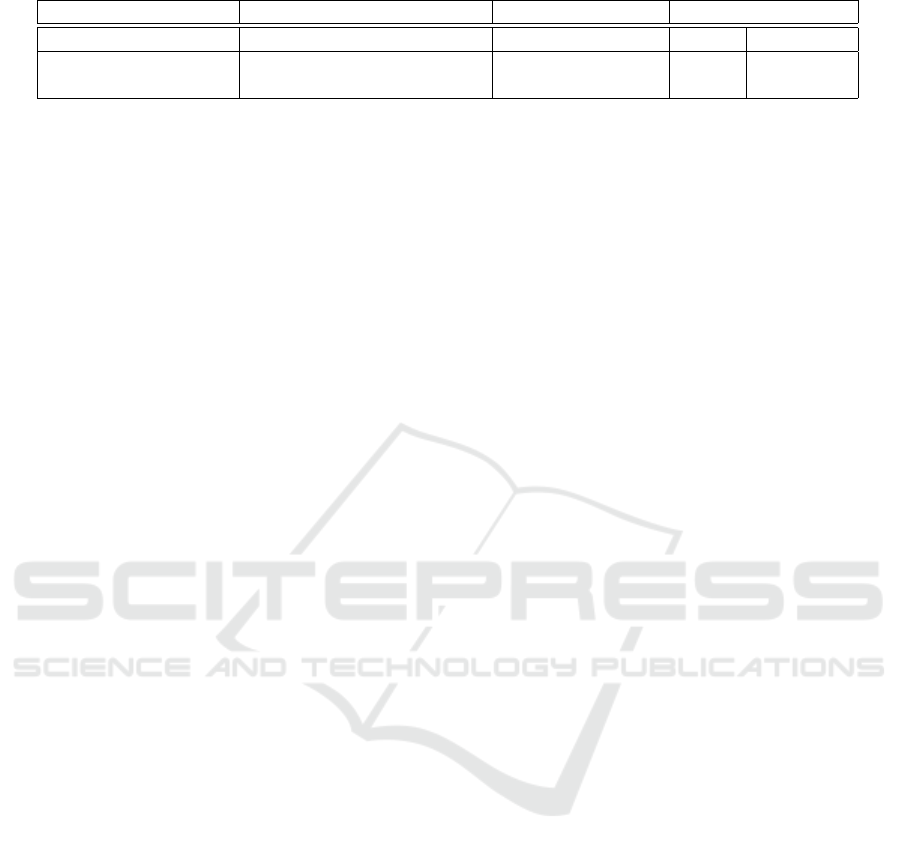
Table 3: Tube 1 Dryer 1: Fisher-Snedecor result test between ”T1 NN1 3” and ”T1 NNC 13”.
Freedom degrees: 149 Risk of error: 5% Lower bound: 0.72 Upper bound: 1.38
NN Name and conf. RMSE: test dataset Dryer 1 Ratio ”T” Pvalue Fisher Test
T1 NN1 3 0.35
2.47 0.00 Reject
T1 NNC 13 0.87
Dataset Dryer 2. The same work performed for
dryer 1 Tube 2 (ref:4.2.2.1 ) is applied on Dryer 2
Tube 2. RMSE of ”T2 NNC 9” on this dataset is 2.02.
The parameters are: degree of freedom: 158, risk of
error: 5%, lower bound: 0.73 and upper bound: 1.37.
The results are a ratio of 2.07 and a Pvalue close to
0. One more time, the conclusions are the same. The
specialized model ’T2 NN2 4” is statistically more ac-
curate than the combined one ”T2 NNC 9”. However,
the performances of the combined model remains ac-
ceptable.
5 CONCLUSIONS
In order to model dryers steam consumption be-
haviours, several MLP have been trained on different
datasets. (single or combination dryer). The goal is
to reduce the number of models, because maintain-
ing one model instead of several is easier and cheaper
in changing industrial environment. To conclude this
paper, the construction of a global model represent-
ing the operation of the two dryers is less effective
than the construction of a dedicated model for each
dryer, due to the use of different raw-material (recy-
cled wood vs. fresh wood) and the different modifica-
tions made during their life leading to a drift between
the behaviors of these two dryers. However, even if
dedicated models are more accurate than combina-
tion one, the performances of combined models re-
main acceptable. Also, if a change in the management
policy of the two dryers were to take place (switch
to recycled material or fresh wood on both dryers),
the construction of a global model would make sense.
Moreover, the behaviors of dryers evolve during the
time due to, as example: fouling, wear and tear and
continual improvement actions. To ensure the perfor-
mance and confidence during the time of these model,
it will be interesting to add statistical process control
tools such as control charts to detect when to update
the models (Thomas et al., 2018). In this work, both
dryers were split in two tubes, and tubes was treated
alone. In futur work, it would be interesting to group
together tube from the same dryer, in order to create a
single dataset for each dryer. Then, same work as pre-
sented in this paper could be done, to compare the per-
formances of a specialized neural network trained on
single dryer with a global neural network trained on
combination dryer, which could be easier for mainte-
nance and retraining, and could be used indifferently
for both dryer.
REFERENCES
Azadbakht, M., Aghili, H., Ziaratban, A., and Torshizi,
M. (2016). Application of artificial neural network
method to exergy and energy analyses of fluidized bed
dryer for potato cubes. Energy.
Buehlmann, U., Ragsdale, C. T., and Gfeller, B. (2000). A
spreadsheet-based decision support system for wood
panel manufacturing. ISPRS Journal of Photogram-
metry and Remote Sensing, 29:21.
Huang, M. and Mujumdar, A. (1993). Use of neural net-
work to predict industrial dryer performance. Dry-
ing Technology: An International Journal, 11(3):525–
541.
Jin, Y., Wong, K. W., Yang, D., Zhang, Z., Wu, W., and Yin,
J. (2021). A neural network model used in continuous
grain dryer control system. Drying Technology: An
International Journal.
Lasi, H., Fettke, P., Kemper, H.-G., Feld, T., and Hoffmann,
M. (2014). Industry 4.0. Business & Information Sys-
tems Engineering, 6(4):239–242.
Martineau, V., Morin, M., Gaudreault, J., Thomas, P., and
Bril El-Haouzi, H. (2021). Neural network architec-
tures and feature extraction for lumber production pre-
diction. In The 34th Canadian Conference on Artifi-
cial Intelligence. Springer.
Nguyen, D. and Widrow, B. (1990). Improving the learning
speed of 2-layer neural networks by choosing initial
values of the adaptive weights. 1990 IJCNN Interna-
tional Joint Conference on Neural Networks, 3:21–26.
Sapna, S. (2012). Backpropagation learning algorithm
based on levenberg marquardt algorithm. Computer
Science & Information Technology ( CS & IT ), pages
393–398.
Thomas, P., El Haouzi, H. B., Suhner, M.-C., Thomas, A.,
Zimmermann, E., and Noyel, M. (2018). Using a clas-
sifier ensemble for proactive quality monitoring and
control: The impact of the choice of classifiers types,
selection criterion, and fusion process. Computers in
Industry, 99:193–204.
Thomas, P. and Thomas, A. (2011). Multilayer percep-
tron for simulation models reduction: Application to
a sawmill workshop. Engineering Applications of Ar-
tificial Intelligence, 24(4):646–657.
NCTA 2022 - 14th International Conference on Neural Computation Theory and Applications
388