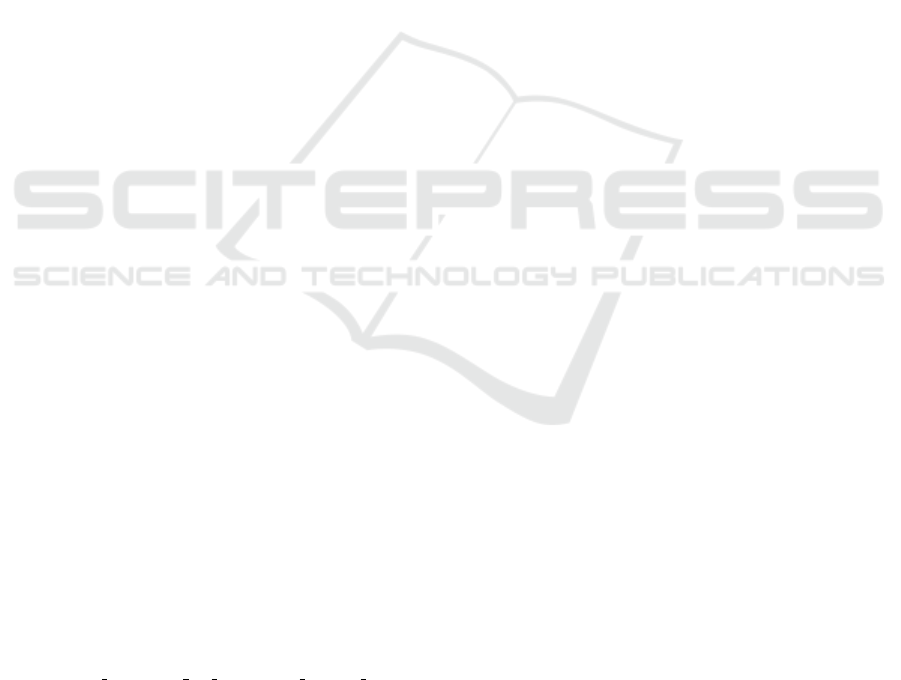
DEXPI (n.d.). Data exchange in the process industry. URL
https://dexpi.org [Visited 28 Jun 2022].
EIF (2017). New European interoperability framework.
European Commission. URL http://doi.org/10.2799/
78681.
Epple, U., Mertens, M., Palm, F., and Azarmipour, M.
(2017). Using properties as a semantic base for inter-
operability. IEEE Trans. Ind. Informat., 13(6):3411–
3419.
Fillinger, S., Esche, E., Tolksdorf, G., Welscher, W., Wozny,
G., and Repke, J.-U. (2019). Data exchange for
process engineering – challenges and opportunities.
Chem. Ing. Tech., 91(3):256–267.
Hendler, J. and Pardo, T. A. (2012). A primer on machine
readability for online documents and data. Data.gov.
URL https://www.data.gov/developers/blog/primer-
machine-readability-online-documents-and-data
[Visited 21 Jun 2022].
H
¨
astbacka, D. and M
¨
at
¨
asniemi, T. (2009). Unifying pro-
cess design with automation and control application
development - an approach based on information inte-
gration and model-driven methods. IFAC Proceedings
Volumes, 42(4):1227–1232. 13th IFAC Symposium
on Information Control Problems in Manufacturing.
IEC 61987-10 (2009). Industrial-process measurement and
control — data structures and elements in process
equipment catalogues — part 10: Lists of properties
(LOPs) for industrial-process measurement and con-
trol for electronic data exchange. IEC.
ISO 15926 (2004). Industrial automation systems and in-
tegration – integration of life-cycle data for process
plants including oil and gas production facilities – part
1: Overview and fundamental principles. ISO.
Jacoby, M., Volz, F., Weißenbacher, C., and M
¨
uller, J.
(2022). FA
3
ST service – an open source implemen-
tation of the reactive Asset Administration Shell. In
First Workshop on Implementing Asset Administration
Shells (ImplAAS). In press.
Kannisto, P., H
¨
astbacka, D., Guti
´
errez, T., Suominen, O.,
Vilkko, M., and Craamer, P. (2022). Plant-wide inter-
operability and decoupled, data-driven process control
with message bus communication. J. Ind. Inf. Integr.,
26:100253.
Kannisto, P., H
¨
astbacka, D., and Marttinen, A. (2020). In-
formation exchange architecture for collaborative in-
dustrial ecosystem. Inf. Syst. Front., 22(3):655–670.
Kim, B. C., Kim, B., Park, S., Teijgeler, H., and Mun, D.
(2020). ISO 15926–based integration of process plant
life-cycle information including maintenance activity.
Concurr. Eng., 28(1):58–71.
Koch, B. (2019). The e-invoicing journey 2019-2025.
Billentis. URL https://www.comarch.com/files-
com/file
441/report the e-invoicing journey 2019-
2025.pdf [Visited 27 Jun 2022].
Lenkenhoff, K., Wilkens, U., Zheng, M., S
¨
uße, T., Kuh-
lenk
¨
otter, B., and Ming, X. (2018). Key challenges
of digital business ecosystem development and how
to cope with them. Procedia CIRP, 73:167–172.
Magas, M. and Kiritsis, D. (2022). Industry commons: an
ecosystem approach to horizontal enablers for sustain-
able cross-domain industrial innovation (a positioning
paper). Int. J. Prod. Res., 60(2):479–492.
Nachira, F., Nicolai, A., and Dini, P. (2007). The digital
business ecosystems: Roots, processes and perspec-
tives. In Nachira, F., Nicolai, A., Dini, P., Le Louarn,
M., and Rivera Leon, L., editors, Digital Business
Ecosystems, pages 1–20. European Commission.
Nast, M., Rother, B., Golatowski, F., Timmermann, D.,
Leveling, J., Olms, C., and Nissen, C. (2020). Work-
in-progress: Towards an International Data Spaces
connector for the Internet of Things. In 2020 16th
IEEE International Conference on Factory Communi-
cation Systems (WFCS), pages 1–4.
OPC UA (2017). OPC unified architecture part 1, overview
and concepts, release 1.04. OPC Foundation.
Panetto, H., Iung, B., Ivanov, D., Weichhart, G., and Wang,
X. (2019). Challenges for the cyber-physical manu-
facturing enterprises of the future. Annu. Rev. Control,
47:200–213.
Papakonstantinou, N., Karttunen, J., Sierla, S., and Vyatkin,
V. (2019). Design to automation continuum for indus-
trial processes: ISO 15926 – IEC 61131 versus an in-
dustrial case. In 2019 24th IEEE International Confer-
ence on Emerging Technologies and Factory Automa-
tion (ETFA), pages 1207–1212.
Pidun, U., Reeves, M., and Sch
¨
ussler, M. (2019). Do you
need a business ecosystem. Boston Consulting Group.
Reif, V. and Meeus, L. (2020). Getting our act together on
the EU interoperability acts. Policy Briefs, Florence
School of Regulation, Energy, 2020/30.
Rulebook for a Fair Data Economy (2021). Rulebook tem-
plate for data networks, version 1.3 en. Sitra.
Scaria, E., Berghmans, A., Pont, M., Arnaut, C., and
Leconte, S. (2018). Study on data sharing between
companies in Europe. European Commission.
SEIIA (2022). P
˚
ag
˚
aende och framtida projekt. URL https:
//seiia.se/projekt/ [Visited 14 Sep 2022].
SGAM (2012). Smart grid reference architecture 3.0. CEN-
CENELEC-ETSI Smart Grid Coordination Group.
Sierla, S., Azangoo, M., Fay, A., Vyatkin, V., and Papakon-
stantinou, N. (2020). Integrating 2D and 3D digi-
tal plant information towards automatic generation of
digital twins. In 2020 IEEE 29th International Sympo-
sium on Industrial Electronics (ISIE), pages 460–467.
Usl
¨
ander, T., Baumann, M., Boschert, S., Rosen, R., Sauer,
O., Stojanovic, L., and Wehrstedt, J. C. (2022). Sym-
biotic evolution of digital twin systems and datas-
paces. Automation, 3(3):378–399.
Vernadat, F. B. (2010). Technical, semantic and organi-
zational issues of enterprise interoperability and net-
working. Annu. Rev. Control, 34(1):139–144.
Weichhart, G., Molina, A., Chen, D., Whitman, L. E., and
Vernadat, F. (2016). Challenges and current devel-
opments for sensing, smart and sustainable enterprise
systems. Comput. Ind., 79:34–46.
Weichhart, G., Stary, C., and Vernadat, F. (2018). Enterprise
modelling for interoperable and knowledge-based en-
terprises. Int. J. Prod. Res., 56(8):2818–2840.
Digitalized Cross-organizational Interoperability in Industrial Business Ecosystems: Implications and Models for Process Industry
241