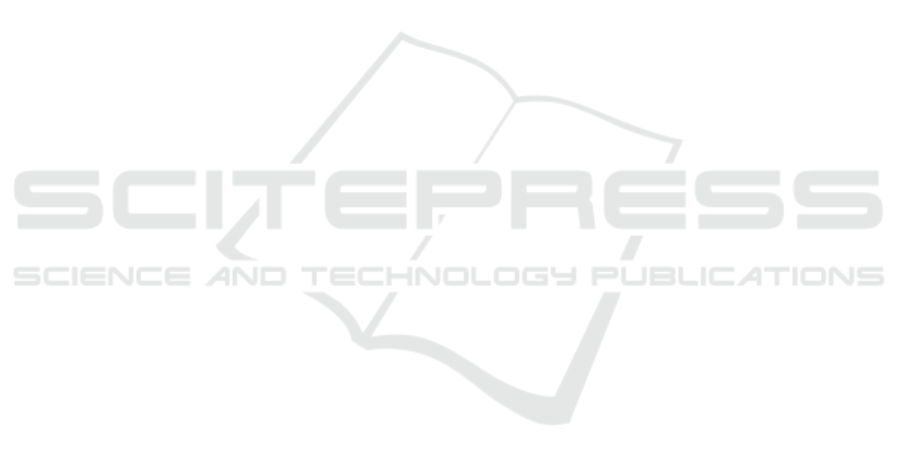
achieves better results in terms of accuracy than other
state-of-the-art methods. Furthermore, considering
the results obtained by measuring competence rein-
forcement, the proposed scheme is less affected by
classification noise due to non-competent classifiers
as it produces better CR results than state-of-the-art
approaches.
Future developments of the architecture will in-
clude testing on real-world data sets, to prove its ef-
fectiveness also in real-world applications. Especially
in this context, it would be useful to have an ex-
plainable artificial intelligence architecture, and since
the proposed approach leverages a number of the bi-
nary base classifiers that are specialized on the deci-
sion boundary between a pair of classes, those can be
fruitfully employed to generate counterfactual expla-
nations, i.e. motivate why a given prediction belongs
to a given class rather than the other one.
ACKNOWLEDGMENT
Work partially supported by (i) the Tuscany Region
in the framework of the ”SecureB2C” project, POR
FESR 2014-2020, Law Decree 7429 31.05.2017;
(ii) the Italian Ministry of University and Research
(MUR), in the framework of the ”Reasoning” project,
PRIN 2020 LS Programme, Law Decree 2493 04-
11-2021; and (iii) the Italian Ministry of Education
and Research (MIUR) in the framework of the Cross-
Lab project (Departments of Excellence). The authors
thank Mirco Quintavalla for his work on the subject
during his master thesis.
REFERENCES
Alfeo, A. L., Barsocchi, P., Cimino, M. G., La Rosa,
D., Palumbo, F., and Vaglini, G. (2018). Sleep be-
havior assessment via smartwatch and stigmergic re-
ceptive fields. Personal and ubiquitous computing,
22(2):227–243.
Alfeo, A. L., Catrambone, V., Cimino, M. G., Vaglini, G.,
and Valenza, G. (2021). Recognizing motor imagery
tasks from eeg oscillations through a novel ensemble-
based neural network architecture. In 2021 43rd An-
nual International Conference of the IEEE Engineer-
ing in Medicine & Biology Society (EMBC), pages
5983–5986. IEEE.
Alfeo, A. L., Cimino, M. G. C., Egidi, S., Lepri, B., Pent-
land, A., and Vaglini, G. (2017a). Stigmergy-based
modeling to discover urban activity patterns from po-
sitioning data. In International Conference on Social
Computing, Behavioral-Cultural Modeling and Pre-
diction and Behavior Representation in Modeling and
Simulation, pages 292–301. Springer.
Alfeo, A. L., Cimino, M. G. C. A., and Vaglini, G. (2017b).
Measuring physical activity of older adults via smart-
watch and stigmergic receptive fields. In The 6th
International Conference on Pattern Recognition Ap-
plications and Methods (ICPRAM 2017), pages 724–
730. PRT.
Bay, S. D., Kibler, D., Pazzani, M. J., and Smyth, P. (2000).
The uci kdd archive of large data sets for data mining
research and experimentation. ACM SIGKDD explo-
rations newsletter, 2(2):81–85.
Belkin, M., Hsu, D., Ma, S., and Mandal, S. (2019). Recon-
ciling modern machine-learning practice and the clas-
sical bias–variance trade-off. Proceedings of the Na-
tional Academy of Sciences, 116(32):15849–15854.
Cevikalp, H. and Polikar, R. (2008). Local classifier weight-
ing by quadratic programming. IEEE Transactions on
Neural Networks, 19(10):1832–1838.
Cimino, M. G., Pedrycz, W., Lazzerini, B., and Marcelloni,
F. (2009). Using multilayer perceptrons as receptive
fields in the design of neural networks. Neurocomput-
ing, 72(10-12):2536–2548.
Costa, V. S., Farias, A. D. S., Bedregal, B., Santiago, R. H.,
and Canuto, A. M. d. P. (2018). Combining multiple
algorithms in classifier ensembles using generalized
mixture functions. Neurocomputing, 313:402–414.
Cruz, R. M., Cavalcanti, G. D., and Ren, T. I. (2010). An
ensemble classifier for offline cursive character recog-
nition using multiple feature extraction techniques. In
The 2010 International Joint Conference on Neural
Networks (IJCNN), pages 1–8. Ieee.
Cruz, R. M., Sabourin, R., and Cavalcanti, G. D. (2018).
Dynamic classifier selection: Recent advances and
perspectives. Information Fusion, 41:195–216.
Duin, R. P. (2002). The combining classifier: to train or
not to train? In Object recognition supported by user
interaction for service robots, volume 2, pages 765–
770. IEEE.
Feng, P., Ma, J., Sun, C., Xu, X., and Ma, Y. (2018). A
novel dynamic android malware detection system with
ensemble learning. IEEE Access, 6:30996–31011.
Galar, M., Fern
´
andez, A., Barrenechea, E., Bustince, H.,
and Herrera, F. (2011). An overview of ensemble
methods for binary classifiers in multi-class prob-
lems: Experimental study on one-vs-one and one-vs-
all schemes. Pattern Recognition, 44(8):1761–1776.
Galar, M., Fern
´
andez, A., Barrenechea, E., and Herrera,
F. (2015). Drcw-ovo: distance-based relative com-
petence weighting combination for one-vs-one strat-
egy in multi-class problems. Pattern recognition,
48(1):28–42.
Galatolo, F. A., Cimino, M. G., Marincioni, A., and Vaglini,
G. (2021). Noise boosted neural receptive fields. In
2021 IEEE Symposium Series on Computational In-
telligence (SSCI), pages 1–6. IEEE.
Goienetxea, I., Mendialdua, I., Rodr
´
ıguez, I., and Sierra, B.
(2021). Problems selection under dynamic selection
of the best base classifier in one versus one: Pseu-
dovo. International Journal of Machine Learning and
Cybernetics, 12(6):1721–1735.
NCTA 2022 - 14th International Conference on Neural Computation Theory and Applications
394