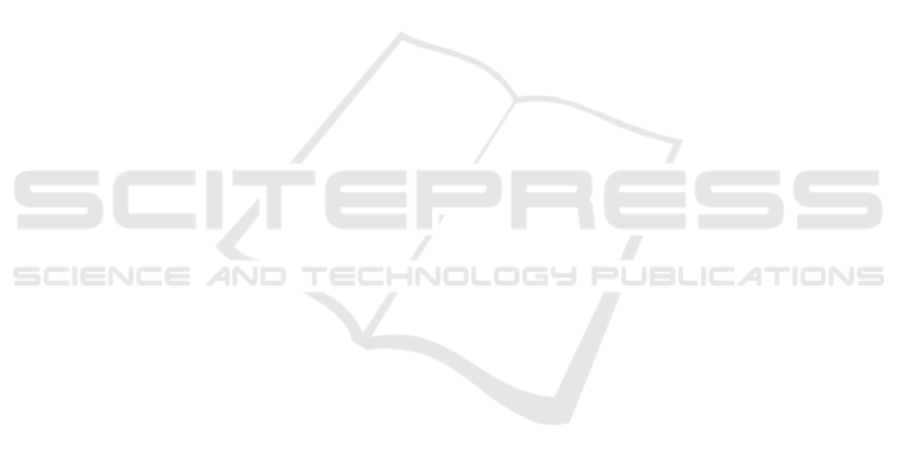
mance. Moreover, assets’ degradation can be mon-
itored with measures such as acoustic noise, power
consumption, torque and many more. By including
all these modalities, it would be possible to test the ca-
pacity of the proposed approach to be data-agnostic,
that is, valid regardless of the measure used to assess
the current degradation stage.
ACKNOWLEDGEMENTS
Work partially supported by (i) the Tuscany Region
in the framework of the SecureB2C project, POR
FESR 2014-2020, Project number 7429 31.05.2017,
(ii) the Italian Ministry of University and Research
(MUR), in the framework of the ”Reasoning” project,
PRIN 2020 LS Programme, Project number 2493 04-
11-2021, and (iii) the Italian Ministry of Education
and Research (MIUR) in the framework of the Cross-
Lab project (Departments of Excellence). The authors
thank Mario Alberto Gherardi for his work on the sub-
ject during his master thesis.
REFERENCES
Alfeo, A. L., Cimino, M. G., Manco, G., Ritacco, E., and
Vaglini, G. (2020). Using an autoencoder in the de-
sign of an anomaly detector for smart manufacturing.
Pattern Recognition Letters, 136:272–278.
Alfeo, A. L., Cimino, M. G., and Vaglini, G. (2021). Tech-
nological troubleshooting based on sentence embed-
ding with deep transformers. Journal of Intelligent
Manufacturing, 32(6):1699–1710.
Alfeo, A. L., Cimino, M. G., and Vaglini, G. (2022). Degra-
dation stage classification via interpretable feature
learning. Journal of Manufacturing Systems, 62:972–
983.
Bengio, Y., Courville, A., and Vincent, P. (2013). Represen-
tation learning: A review and new perspectives. IEEE
transactions on pattern analysis and machine intelli-
gence, 35:1798–1828.
Cano, J.-R. (2013). Analysis of data complexity measures
for classification. Expert systems with applications,
40(12):4820–4831.
Deng, L. (2014). A tutorial survey of architectures, algo-
rithms, and applications for deep learning. APSIPA
transactions on Signal and Information Processing, 3.
Elie Bursztein, James Long, S. L. O. V. F. C. (2021). Ten-
sorflow similarity: A usable, high-performance metric
learning library. Fixme.
Gao, J., Li, P., Chen, Z., and Zhang, J. (2020). A survey
on deep learning for multimodal data fusion. Neural
Computation, 32(5):829–864.
Gecgel, O., Ekwaro-Osire, S., Gulbulak, U., and Morais,
T. S. (2022). Deep convolutional neural network
framework for diagnostics of planetary gearboxes un-
der dynamic loading with feature-level data fusion.
Journal of Vibration and Acoustics, 144(3).
Hamadache, M., Jung, J. H., Park, J., and Youn,
B. D. (2019). A comprehensive review of artifi-
cial intelligence-based approaches for rolling element
bearing phm: shallow and deep learning. JMST Ad-
vances, 1(1):125–151.
Jimenez, J. J. M., Schwartz, S., Vingerhoeds, R., Grabot,
B., and Sala
¨
un, M. (2020). Towards multi-model ap-
proaches to predictive maintenance: A systematic lit-
erature survey on diagnostics and prognostics. Journal
of Manufacturing Systems, 56:539–557.
Kimotho, J. K., Sondermann-W
¨
olke, C., Meyer, T., and
Sextro, W. (2013). Machinery prognostic method
based on multi-class support vector machines and hy-
brid differential evolution–particle swarm optimiza-
tion. Chemical Engineering Transactions, 33.
Lei, Y., Li, N., Guo, L., Li, N., Yan, T., and Lin, J. (2018).
Machinery health prognostics: A systematic review
from data acquisition to rul prediction. Mechanical
systems and signal processing, 104:799–834.
Liu, Y., Hu, Z., and Zhang, Y. (2021). Bearing feature
extraction using multi-structure locally linear embed-
ding. Neurocomputing, 428:280–290.
Lorena, A. C., Garcia, L. P., Lehmann, J., Souto, M. C., and
Ho, T. K. (2019). How complex is your classification
problem? a survey on measuring classification com-
plexity. ACM Computing Surveys (CSUR), 52(5):1–
34.
Mao, W., He, J., and Zuo, M. J. (2019). Predicting re-
maining useful life of rolling bearings based on deep
feature representation and transfer learning. IEEE
Transactions on Instrumentation and Measurement,
69(4):1594–1608.
Merkt, O. (2019). Predictive models for maintenance opti-
mization: an analytical literature survey of industrial
maintenance strategies. Information Technology for
Management: Current Research and Future Direc-
tions, pages 135–154.
Nectoux, P., Gouriveau, R., Medjaher, K., Ramasso, E.,
Chebel-Morello, B., Zerhouni, N., and Varnier, C.
(2012). Pronostia: An experimental platform for bear-
ings accelerated degradation tests. In IEEE Interna-
tional Conference on Prognostics and Health Man-
agement, PHM’12., pages 1–8. IEEE Catalog Num-
ber: CPF12PHM-CDR.
Nelli, F. (2018). Machine learning with scikit-learn. In
Python Data Analytics, pages 313–347. Springer.
Ngiam, J., Khosla, A., Kim, M., Nam, J., Lee, H., and Ng,
A. Y. (2011). Multimodal deep learning. In ICML.
Nguyen, M. H. (2019). Impacts of unbalanced test data
on the evaluation of classification methods. Recall,
100:90–00.
Pandarakone, S. E., Masuko, M., Mizuno, Y., and Naka-
mura, H. (2018). Deep neural network based bearing
fault diagnosis of induction motor using fast fourier
transform analysis. In 2018 IEEE energy conversion
congress and exposition (ECCE), pages 3214–3221.
IEEE.
IN4PL 2022 - 3rd International Conference on Innovative Intelligent Industrial Production and Logistics
102