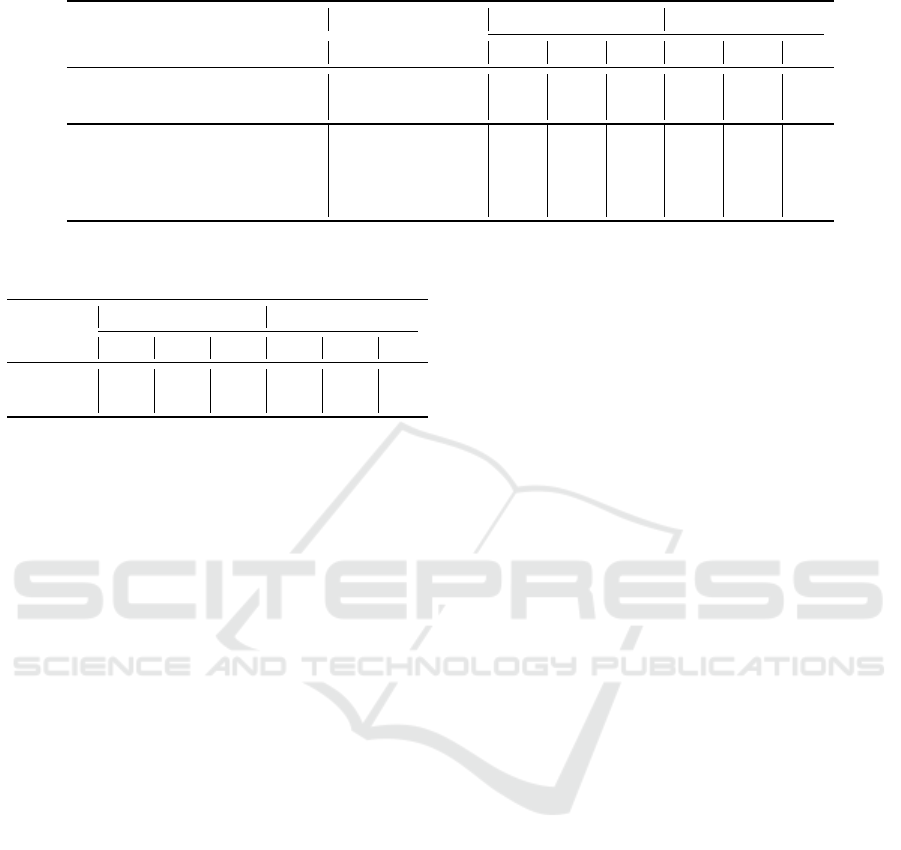
Table 4: ATE results. Best unsupervised results in bold.
Method Type
Sem2014-Rest Sem2014-Laptop
P R F1 P R F1
SemEval (Pontiki et al., 2014) Supervised 0.52 0.43 0.47 0.44 0.30 0.36
Wu et al. (2018) Semi-Supervised 0.73 0.79 0.76 0.56 0.67 0.61
Garcıa-Pablos et al. (2014) Unsupervised 0.56 0.65 0.61 0.32 0.43 0.37
Giannakopoulos et al. (2017) Unsupervised 0.74 0.42 0.54 0.67 0.31 0.42
Venugopalan and Gupta (2020) Unsupervised 0.53 0.82 0.64 0.45 0.69 0.55
UNATE Unsupervised 0.70 0.69 0.69 0.51 0.43 0.47
Table 5: ATE with an automatically annotated dataset.
Method
Sem2014-Rest Sem2014-Laptop
P R F1 P R F1
SemEval
S
0.52 0.43 0.47 0.44 0.30 0.36
SemEval
U
0.56 0.58 0.57 0.33 0.19 0.24
able alternative when no annotation is available.
As future work, we plan to use UNATE to auto-
matically generate a domain lexicon that can be used
in tasks such as aspect classification, clustering, and
visualization. Finally, like Tubishat et al. (2021), we
could explore a larger set of rules.
Acknowledgments: This work has been financed in
part by CAPES Finance Code 001 and CNPq/Brazil.
REFERENCES
Blei, D. M. (2012). Probabilistic topic models. Commun.
ACM, 55(4):77–84.
Bojanowski, P., Grave, E., Joulin, A., and Mikolov, T.
(2016). Enriching word vectors with subword infor-
mation. CoRR, abs/1607.04606.
Brody, S. and Elhadad, N. (2010). An unsupervised aspect-
sentiment model for online reviews. In NAACL, pages
804–812.
Chandola, V., Banerjee, A., and Kumar, V. (2009).
Anomaly detection: A survey. ACM computing sur-
veys (CSUR), 41(3):1–58.
Devlin, J., Chang, M.-W., Lee, K., and Toutanova, K.
(2018). Bert: Pre-training of deep bidirectional trans-
formers for language understanding. arXiv preprint
arXiv:1810.04805.
Garcıa-Pablos, A., Cuadros, M., Gaines, S., and Rigau, G.
(2014). V3: Unsupervised generation of domain as-
pect terms for aspect based sentiment analysis. In Se-
mEval, pages 833–837.
Giannakopoulos, A., Musat, C., Hossmann, A., and
Baeriswyl, M. (2017). Unsupervised aspect term ex-
traction with B-LSTM & CRF using automatically la-
belled datasets. CoRR, abs/1709.05094.
Han, X. (2009). Why can’t we dispense with the subject-
predicate form without losing something more?
Florida Philosophical Review, 9(2):79.
Hu, M. and Liu, B. (2004). Mining and summarizing cus-
tomer reviews. In SIGKDD, pages 168–177.
International Telecommunication Union (2018). Measuring
the information society report.
Jakob, N. and Gurevych, I. (2010). Extracting opinion tar-
gets in a single and cross-domain setting with condi-
tional random fields. In EMNLP, pages 1035–1045.
Jorij, A. (2017). Global ecommerce report 2017.
Liu, B. (2012). Sentiment Analysis and Opinion Mining.
Synthesis Lectures on Human Language Technolo-
gies. Morgan & Claypool Publishers.
Liu, B. (2015). Sentiment Analysis - Mining Opinions, Sen-
timents, and Emotions. Cambridge University Press.
Liu, P., Joty, S. R., and Meng, H. M. (2015). Fine-grained
opinion mining with recurrent neural networks and
word embeddings. In EMNLP, pages 1433–1443.
Luo, H., Li, T., Liu, B., and Zhang, J. (2019). DOER:
dual cross-shared RNN for aspect term-polarity co-
extraction. In ACL, pages 591–601.
Mikolov, T., Sutskever, I., Chen, K., Corrado, G. S., and
Dean, J. (2013). Distributed representations of words
and phrases and their compositionality. In NIPS, pages
3111–3119.
Pennington, J., Socher, R., and Manning, C. D. (2014).
Glove: Global vectors for word representation. In
EMNLP, pages 1532–1543.
Pontiki, M., Galanis, D., Papageorgiou, H., Manandhar, S.,
and Androutsopoulos, I. (2015). Semeval-2015 task
12: Aspect based sentiment analysis. In SemEval,
pages 486–495.
Pontiki, M., Galanis, D., Pavlopoulos, J., Papageorgiou,
H., Androutsopoulos, I., and Manandhar, S. (2014).
Semeval-2014 task 4: Aspect based sentiment analy-
sis. In SemEval, pages 27–35.
Qiu, G., Liu, B., Bu, J., and Chen, C. (2011). Opinion word
expansion and target extraction through double prop-
agation. Computational Linguistics, 37(1):9–27.
Rogers, A., Kovaleva, O., and Rumshisky, A. (2020). A
primer in bertology: What we know about how bert
works. Transactions of the Association for Computa-
tional Linguistics, 8:842–866.
Unsupervised Aspect Term Extraction for Sentiment Analysis through Automatic Labeling
353